Multichannel Linear Prediction-Based Speech Dereverberation Considering Sparse and Low-Rank Priors.
IEEE ACM Trans. Audio Speech Lang. Process.(2024)
摘要
This paper addresses the multi-channel linear prediction (MCLP)-based speech dereverberation problem by jointly considering the sparsity and low-rank priors of speech spectrograms. We utilize the complex generalized Gaussian (CGG) distribution as the source model and the generalized nonnegative matrix factorization (NMF) as the spectral model. The difference between the presented model and existing ones for MCLP is twofold. First, we adopt the CGG distribution with a timefrequency-variant scale parameter instead of that with a timefrequency-invariant scale parameter. Second, the time-frequencyvarying scale parameter is approximated by NMF in a lowrank manner. Based on the maximum-likelihood criterion, speech dereverberation is formulated as an optimization problem that minimizes the prediction error weighted by the reciprocal of sparse and low-rank parameters. A convergence-guaranteed algorithm is derived to estimate the parameters using the majorization-minimization technology. The WPE, NMF-based WPE and CGG-based WPE can be treated as special cases of the proposed method with different shape and domain parameters. As a byproduct, the proposed method provides a simple and elegant way to derive the CGG-based WPE algorithm. A series of experiments show the superiority of the proposed method over WPE, NMF-based WPE and CGG-based WPE methods.
更多查看译文
关键词
Speech dereverberation,multichannel linear prediction,weighted prediction error,complex generalized Gaussian,nonnegative matrix factorization
AI 理解论文
溯源树
样例
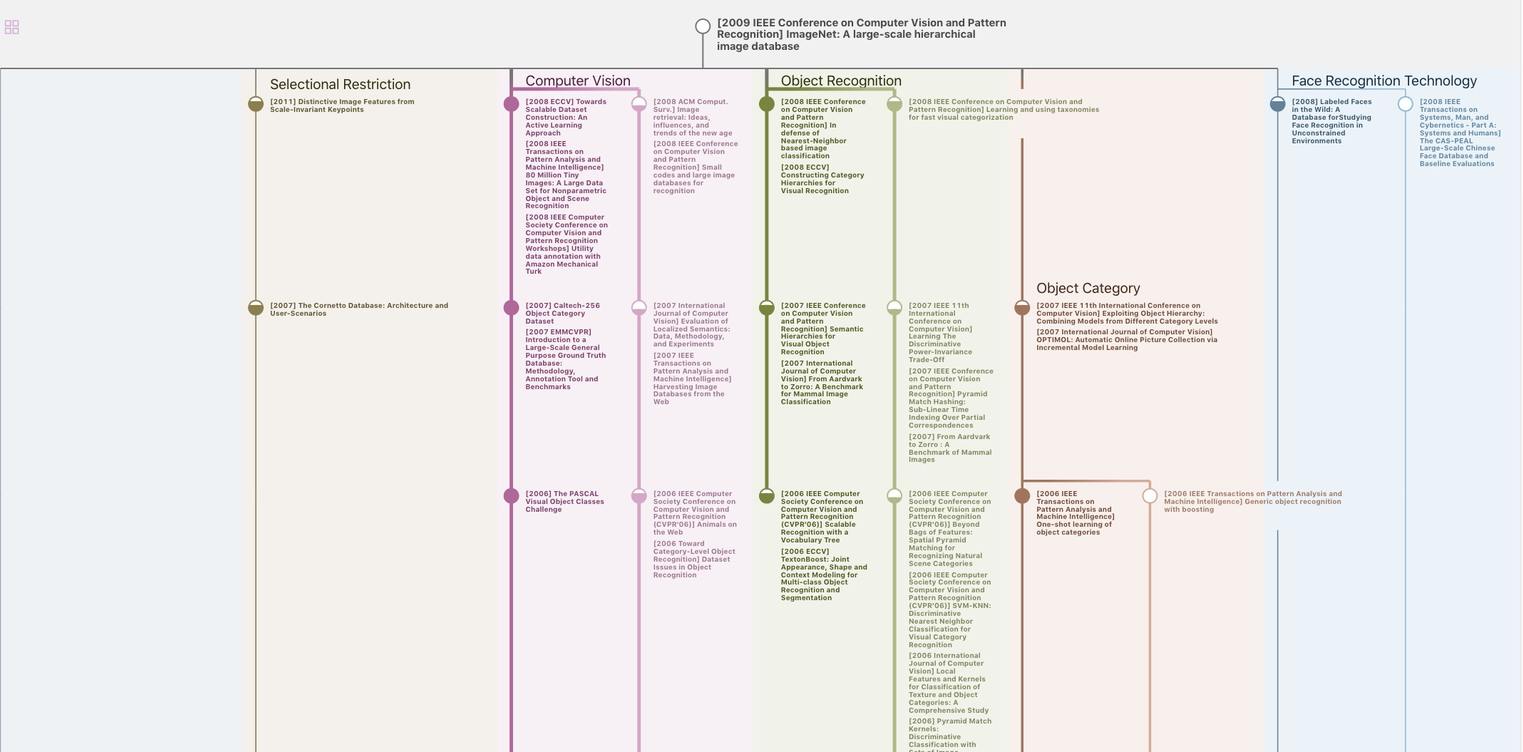
生成溯源树,研究论文发展脉络
Chat Paper
正在生成论文摘要