Recurrent Neural Network-Assisted Truncation of Convolutional Perfectly Matched Layers for FDTD
IEEE antennas and wireless propagation letters/Antennas and wireless propagation letters(2024)
Abstract
To improve the efficiency of finite-difference time-domain (FDTD) method for unbounded electromagnetic problems, a truncation method for the convolutional perfectly matched layers (CPMLs) is proposed based on a recurrent neural network (RNN). In this work, the RNN is used to capture the time-marching information in the FDTD, complete the offline training of field components at the truncated CPML interfaces, and predict the field components on the cells of the truncated interfaces at each time step. By using this method, the unknowns are well reduced, which results in an improvement in computational efficiency. Additionally, since some of the CPMLs are preserved, the late-time electromagnetic response is still stable without error accumulation. The proposed method can balance accuracy and efficiency by an optimal truncation position for CPMLs, which is validated by two numerical examples.
MoreTranslated text
Key words
Finite difference methods,Time-domain analysis,Artificial neural networks,Computational efficiency,Recurrent neural networks,Perfectly matched layers,Mathematical models,Absorbing boundary condition,convolutional perfectly matched layer (CPML),finite-difference time-domain (FDTD),neural network (NN)
AI Read Science
Must-Reading Tree
Example
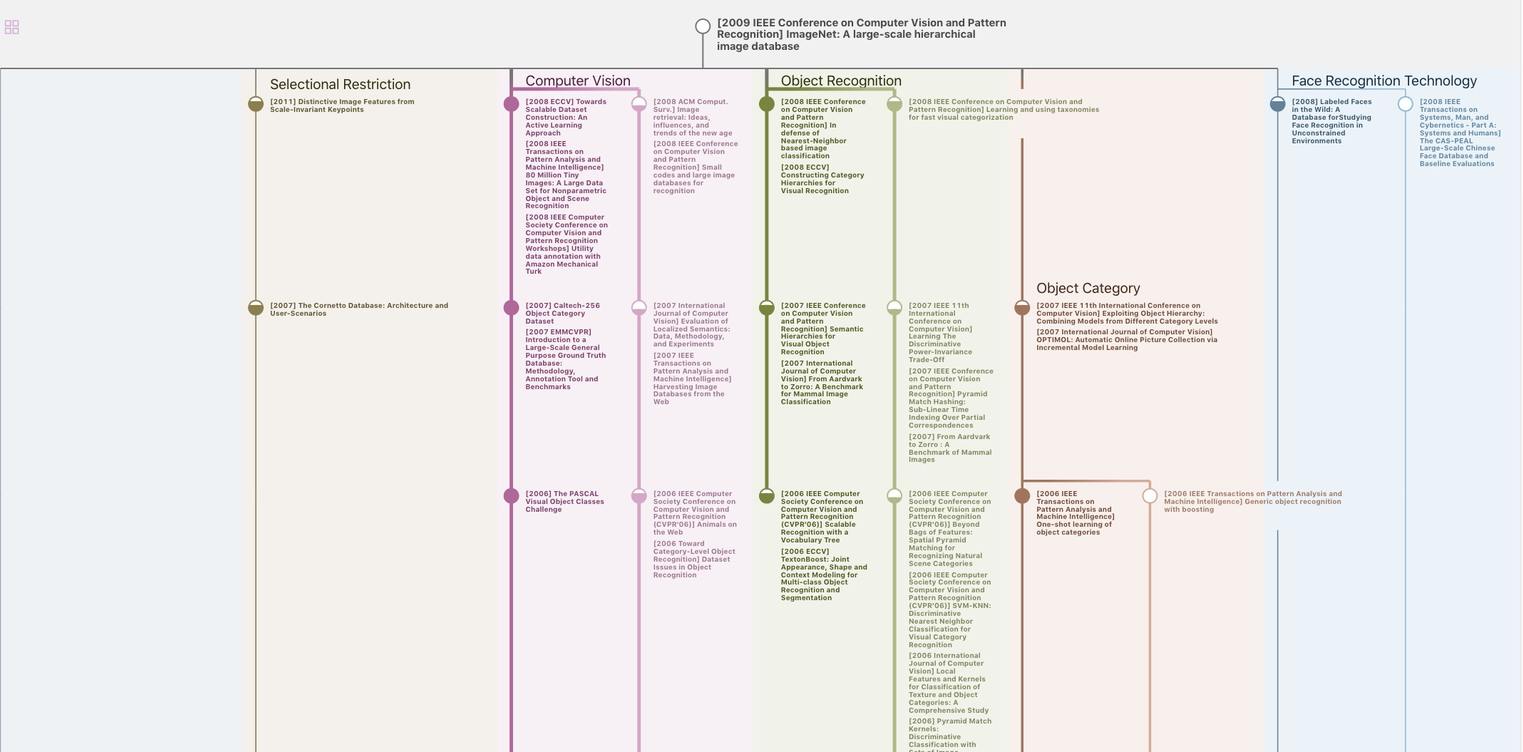
Generate MRT to find the research sequence of this paper
Chat Paper
Summary is being generated by the instructions you defined