ViT-FuseNet: Multimodal Fusion of Vision Transformer for Vehicle-Infrastructure Cooperative Perception
IEEE ACCESS(2024)
摘要
Perception plays a vital role in autonomous driving as it serves as a prerequisite for downstream planning and decision tasks. Existing research has mainly focused on developing vehicle-side perception models using a single type of sensors. However, relying solely on one type of on-board sensors to perceive the surrounding environment leads to perceptual deficiencies owing to inherent characteristics and sensor sparsity. To address this bottleneck, we propose ViT-FuseNet, a novel vehicle-infrastructure cooperative perception framework that utilizes a Vision Transformer to fuse feature maps extracted from LiDAR and camera data. The key component is a multimodal fusion module designed based on a cross-attention mechanism. ViT-FuseNet has two distinct advantages: i) it incorporates roadside LiDAR point clouds as additional inputs to enhance the 3D object detection capability of the vehicle; and ii) for the effective fusion of data from two different modal sensors, we employ a cross-attention mechanism for feature fusion, rather than directly merging camera features with point clouds at the raw data level. Extensive experiments are conducted using the DAIR-V2X Dataset to demonstrate the effectiveness of the proposed method. Compared with advanced cooperative perception methods, our method achieves a 6.17% improvement in 3D-mAP (IoU=0.5) and an 8.72% improvement in 3D-mAP (IoU=0.7). Moreover, the framework achieves the highest 3D-mAP (IoU=0.5) in all three object categories of benchmarks for single-vehicle perception.
更多查看译文
关键词
Vehicle-infrastructure cooperative perception,multimodal fusion,object detection,vision transformer,cross-attention
AI 理解论文
溯源树
样例
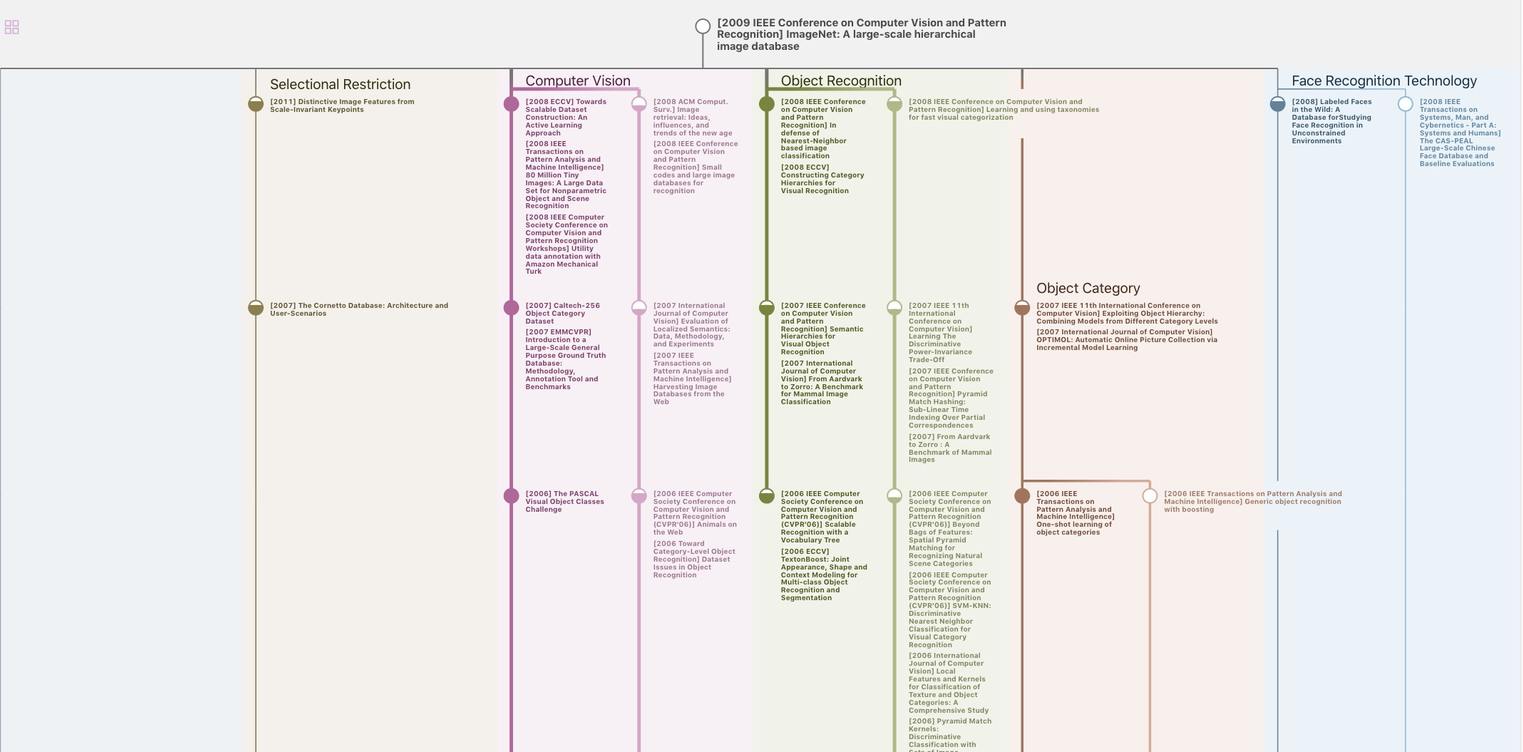
生成溯源树,研究论文发展脉络
Chat Paper
正在生成论文摘要