Target-Aware Tracking with Spatial-Temporal Context Attention
IEEE Transactions on Circuits and Systems for Video Technology(2024)
摘要
Current trackers only rely on a fixed target template to localize the target in each frame, which is however prone to fail in case of fast appearance changes or the presence of distractor objects. Having some historical knowledge about the tracked targets as well as their surrounding scenes can be highly beneficial for robust tracking. This historical information can be propagated through the sequence and used to timely perceive the change in target appearance and explicitly avoid distractor objects. In this work, we propose a Spatial-Temporal Context Attention (STCA) model which utilizes the appearance and state information of previously tracked targets as well as their surrounding scenes to more accurately localize the real target in the current frame. We embed an improved position encoder into the STCA, which enables the target template, context template and search patch to perform extensive interactional fusion through simultaneously self-attention and cross-attention calculation. By embedding the STCA module into Transformer, we construct a target-aware based online tracking network (named TATrack) that has a backbone to extract features better suited to the tracking task, a neck to further suppress distractors and highlight target, and a classification-regression head to make the tracking scores consistently reflect the quality of the bounding boxes. In addition, we also design a simple yet effective online updating approach to select high-quality context templates. Our tracker reaches the latest level on several benchmarks, including LaSOT, TrackingNet, GOT10k, OTB100 and UAV123. The code and trained models are available at https://github.com/hekaijie123/TATrack.
更多查看译文
关键词
Visual tracking,Online update,Feature fusion,Background clutters
AI 理解论文
溯源树
样例
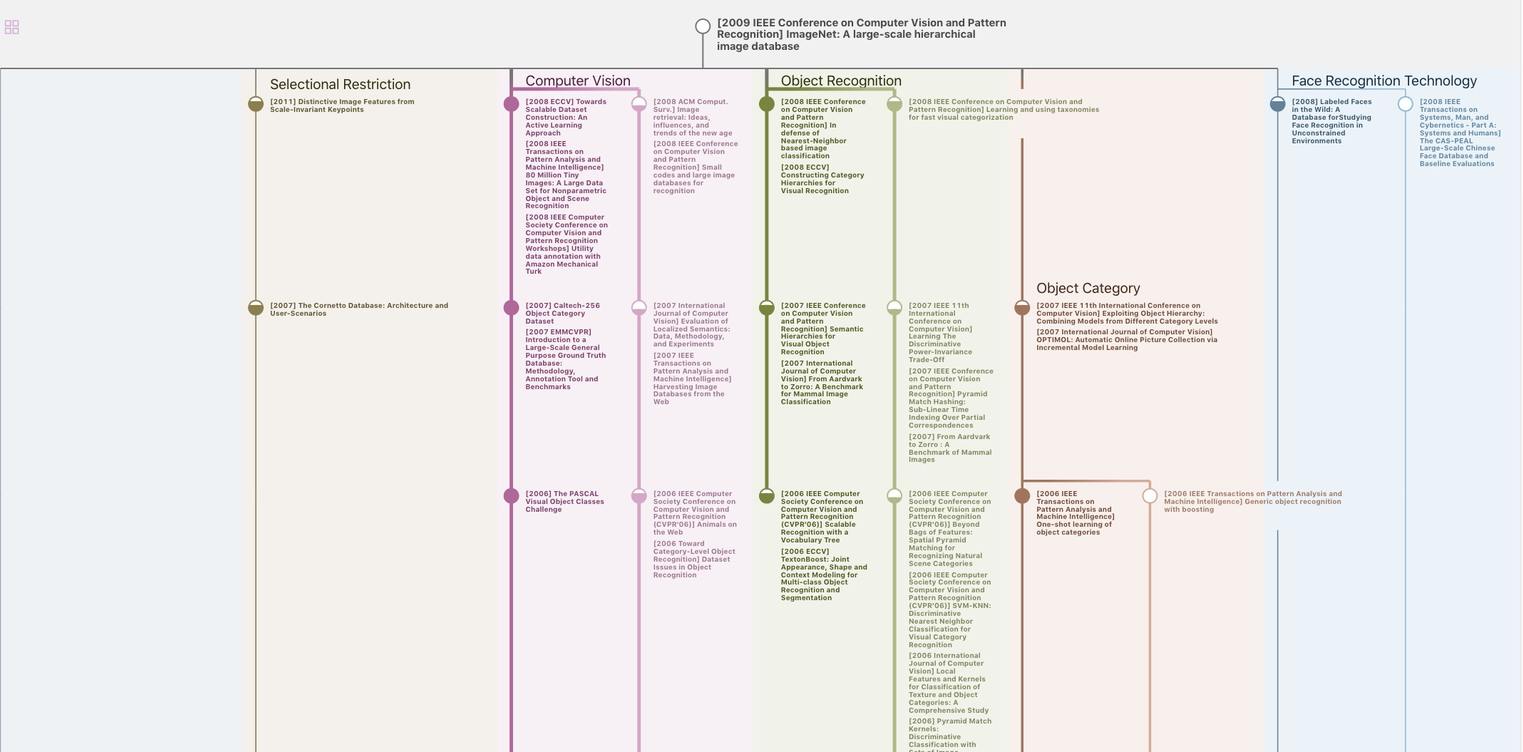
生成溯源树,研究论文发展脉络
Chat Paper
正在生成论文摘要