Online Informative Path Planning of Autonomous Vehicles Using Kernel-Based Bayesian Optimization
IEEE Transactions on Circuits and Systems II: Express Briefs(2024)
摘要
To improve environmental information gathering of intelligent vehicles in unknown scenes, this brief presents a hierarchical online informative path planning (IPP) framework containing global action optimization and local path planning. Particularly, we propose a lightweight kernel-based Bayesian optimization for IPP (KBO-IPP) to facilitate highly efficient information utility evaluation and decision-making of control actions. Specifically, KBO-IPP can infer the exact environmental mutual information (MI) and associated uncertainties with an approximate logarithmic complexity, eliminating the need for explicit model training. We develop a new information-theoretic objective function consisting of travel cost and predicted MI values with uncertainties to achieve the balance between high MI values (exploitation) and high prediction variances (exploration). To enhance the optimality of IPP, the past unselected informative actions are also incorporated into the global Bayesian optimization. Online real-world experiments validate that our proposed method shows higher efficiency with comparable performance to modern methods in unknown, complex environments.
更多查看译文
关键词
Informative path planning,mutual information,Bayesian optimization,kernel inference,intelligent vehicle
AI 理解论文
溯源树
样例
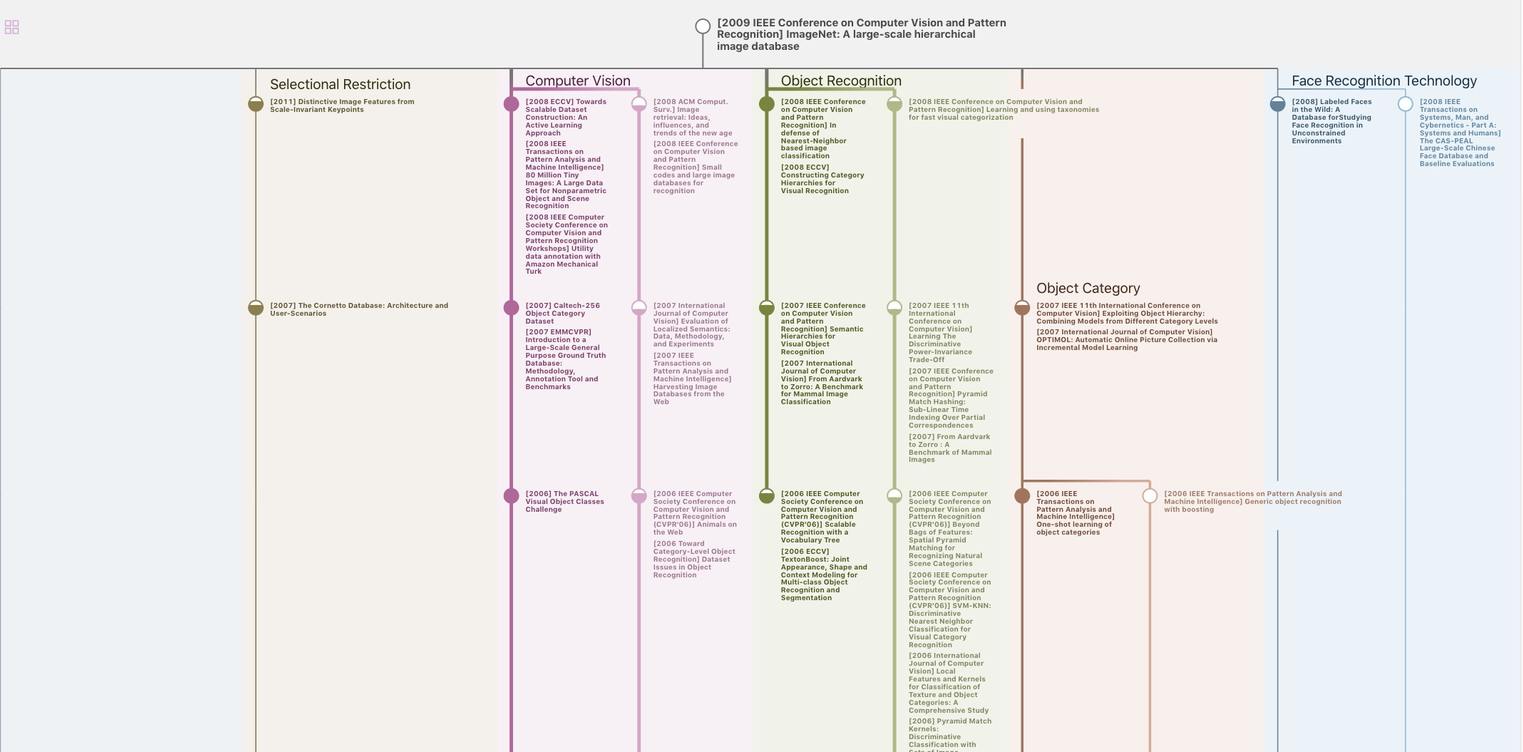
生成溯源树,研究论文发展脉络
Chat Paper
正在生成论文摘要