Adaptive Conditional Denoising Diffusion Model with Hybrid Affinity Regularizer for Generalized Zero-shot Learning
IEEE Transactions on Circuits and Systems for Video Technology(2024)
摘要
Generalized zero-shot learning (GZSL) is a challenging topic in both computer vision and machine learning. Recently, generative models (
e.g
., GAN and VAE) have attracted much attention for handling the GZSL task, however, they are sometimes prone to either model collapse or ambiguous distribution modeling. Inspired by the feature generation ability of denoising diffusion models in other visual tasks, we propose an Adaptive Conditional Denoising Diffusion Model to synthesize unseen-class visual features for GZSL on condition of a set of semantic features in this paper, called AC-DDM. Unlike traditional denoising diffusion models whose reverse process has both a fixed time interval and a fixed number of total denoising time steps, the proposed AC-DDM has a learnable distribution-constrained predictor which could adaptively learn the time interval and the number of total denoising time steps for each unseen class, so that it could synthesize more discriminative features for sample classification. In order to improve the discrimination ability of the synthesized visual features further, we also explore a hybrid affinity regularizer under the proposed AC-DDM, which forces the differences among the affinity matrices of the real and synthesized visual features to be small. Extensive experimental results on four public benchmark datasets demonstrate the superiority of the proposed model over 20 state-of-the-art models in both the ZSL and GZSL tasks.
更多查看译文
关键词
Denoising diffusion model,adaptive feature synthesis,generalized zero-shot learning
AI 理解论文
溯源树
样例
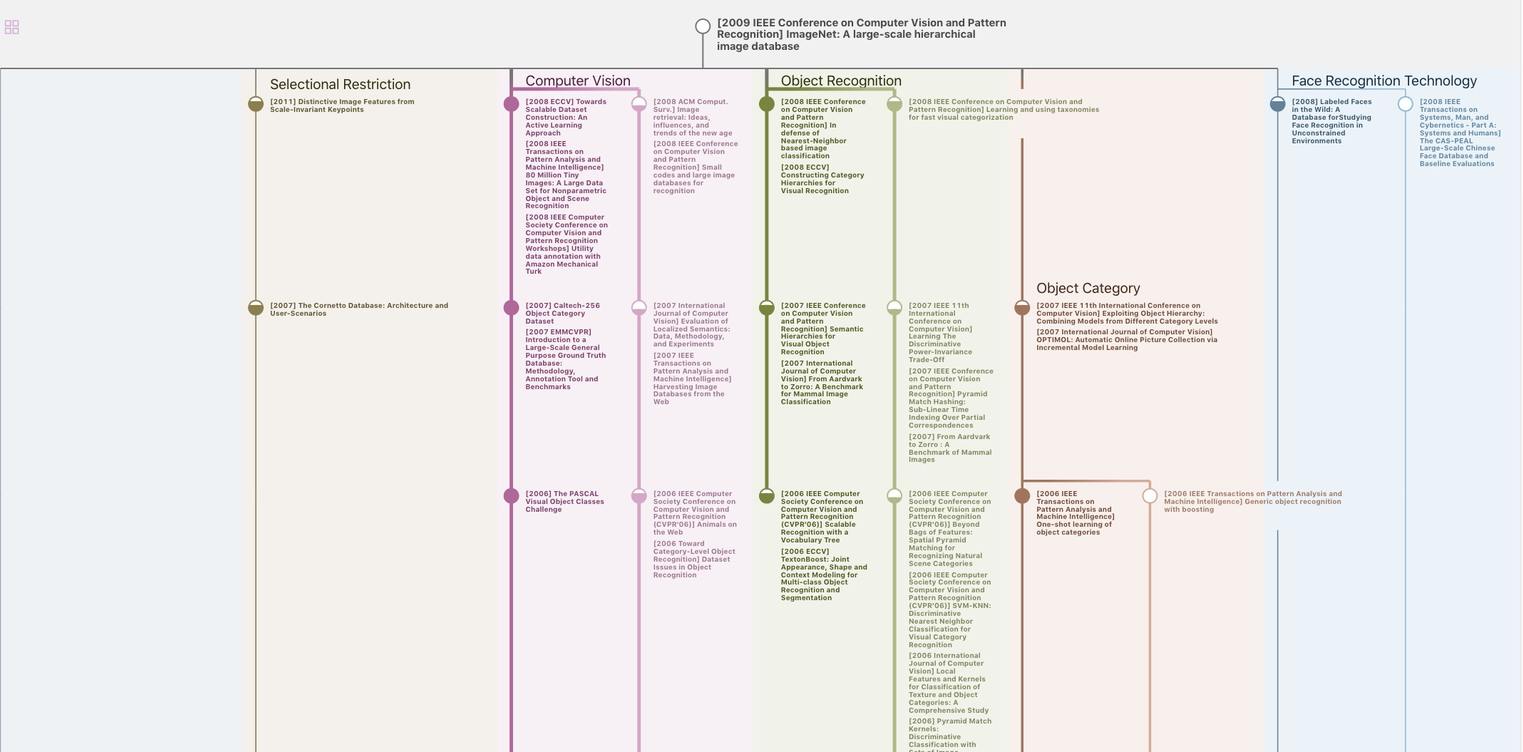
生成溯源树,研究论文发展脉络
Chat Paper
正在生成论文摘要