Harnessing Optimal Deep Learning for Consumer Interest Monitoring Through Advanced Face Analysis
IEEE transactions on consumer electronics(2024)
摘要
A facial image analysis system for monitoring customer interest employs cutting-edge facial detection technology for evaluating and analyzing customers’ expressions, offering real-time perceptions of their responses and preferences. Leveraging advanced neural networks (NNs) can dynamically and correctly analyze facial expressions, allowing retailers to separate and interpret customers’ emotions with remarkable accuracy. Deep learning (DL) systems surpass at capturing difficult patterns and nuances in facial features. This study paper presents a novel jellyfish optimizer algorithm with Deep Learning for Consumer Interest Monitoring Advanced Face Analysis (JOADL-CIMAFA) model. The main intention of the JOADL-CIMAFA method is to analyze the facial images of the consumer using the DL model for the detection and classification of customer interest. In the presented JOADL-CIMAFA technique, the EfficientNet model can be applied to the feature extraction process. For the hyperparameter tuning procedure, the JOA can be used for optimum hyperparameter selection of the EfficientNet model. Furthermore, the long short-term memory (LSTM) technique can be exploited for the identification and classification of consumer interest. To establish the enhanced outcome of the JOADL-CIMAFA system, a widespread of simulations can be implemented. The experimental values highlighted that the JOADL-CIMAFA technique illustrates superior performance over other models in terms of different measures.
更多查看译文
关键词
Feature extraction,Face recognition,Analytical models,Tuning,Monitoring,Statistics,Sociology,Consumer behavior analysis,deep learning,face analysis,jellyfish optimization algorithm,feature extraction
AI 理解论文
溯源树
样例
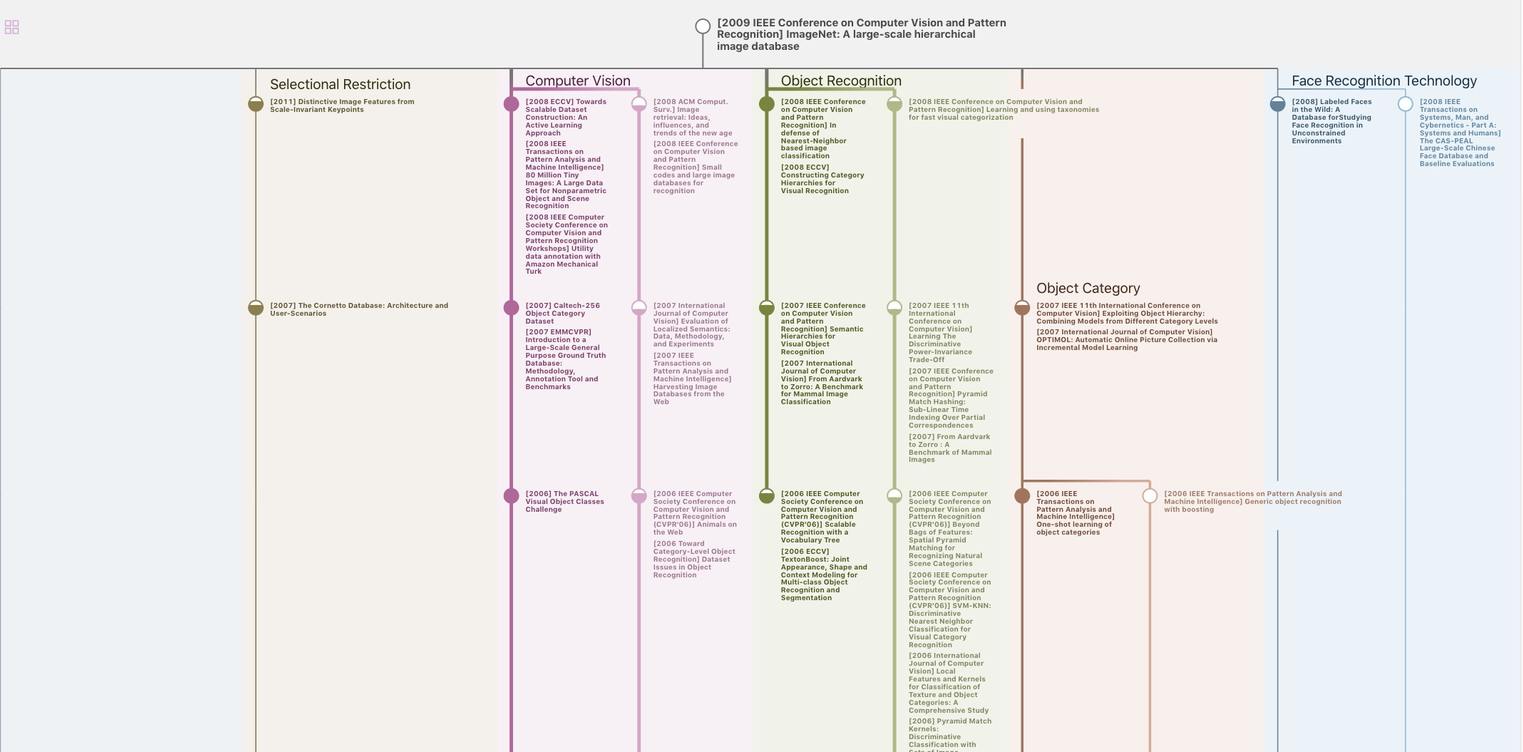
生成溯源树,研究论文发展脉络
Chat Paper
正在生成论文摘要