Efficient Point Cloud Video Recognition via Spatio-Temporal Pruning for MEC-Based Consumer Applications.
IEEE Trans. Consumer Electron.(2024)
摘要
3D point cloud videos are widely used in edge computing scenarios to achieve seamless connectivity and extremely low latency in applications such as autonomous driving. It faces difficulties primarily because point cloud data is often voluminous and contains redundancies. These issues can lead to significant drawbacks in practical applications, causing inference delays and reducing accuracy. To tackle these challenges, we propose a novel approach: a transformer-based spatio-temporal pruning method designed specifically for point cloud videos. Our goal is to expedite network inference. Initially, we utilize the attention mechanism of the transformer to calculate importance scores for different frames and tokens within 3D point cloud videos. To capture the temporal context of each point cloud token, we introduce a frame-wise relation matrix for importance score computation. Subsequently, we use these importance scores as criteria for pruning at both frame and token levels. The experimental results indicate that our approach outperforms other methods. For example, on NTU RGB+D 60 dataset, it shows improvement in accuracy by 0.5% and the average inference time decrease by 28%, while memory consumption is reduced by 28%.
更多查看译文
关键词
Deep learning,Point cloud Video,Dual-scale pruning,Mobile Edge Computing,Transformer
AI 理解论文
溯源树
样例
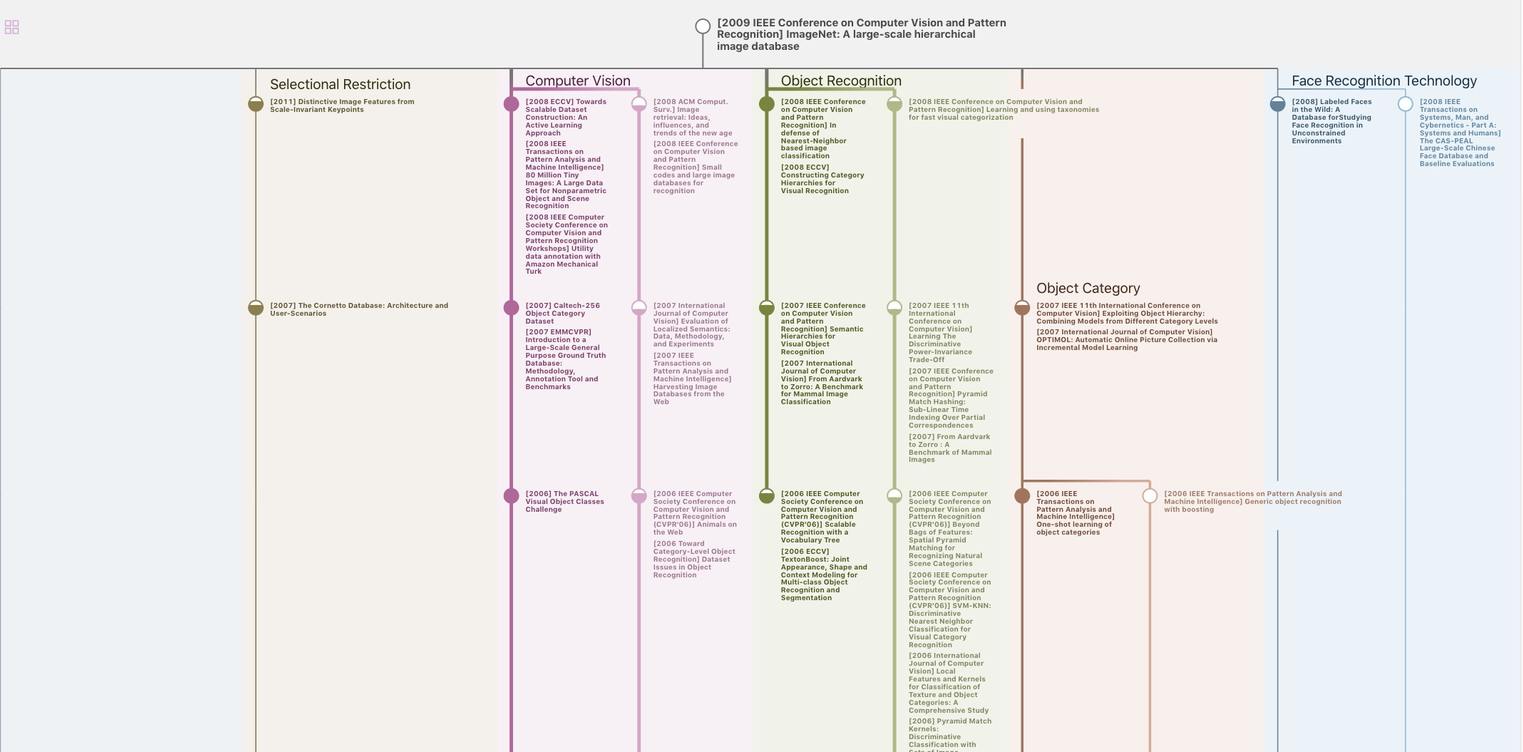
生成溯源树,研究论文发展脉络
Chat Paper
正在生成论文摘要