DSFL: A Decentralized SplitFed Learning Approach for Healthcare Consumers in the Metaverse
IEEE transactions on consumer electronics(2024)
摘要
The consumer health industry has witnessed a transformative revolution driven by the Internet of Medical Things (IoMT) and the Metaverse. Additionally, Artificial Intelligence (AI) further enhances this evolution by unlocking the potential for data-driven insights. Yet, there is an imperative requirement to safeguard consumer healthcare data, particularly with the challenges posed by resource-constrained consumer healthcare devices and the complex, non-Independent and Identically Distributed (non-IID) nature of data flows between the Metaverse and healthcare entities. The introduction of Digital Twin (DTs) has emerged as a groundbreaking innovation in the consumer health industry, offering real-time, dynamic digital representations of physical entities and providing invaluable insights and situational awareness. To address the multifaceted challenges, this paper introduces Decentralized SplitFed Learning (DSFL), a collaborative AI learning technique designed for deployment within the resource-constrained consumer health industry environment. DSFL optimizes resource utilization and mitigates computation burdens through decentralized model distribution. Furthermore, DSFL tackles the issue of non-IID data distribution within the dynamic Metaverse-driven consumer healthcare ecosystem by incorporating a clustering-based mechanism, which enhances learning performance by aligning the DTs’ representations of healthcare consumers’ real-time data statistics with the everchanging data streams within the Metaverse.
更多查看译文
关键词
Medical services,Metaverse,Servers,Computational modeling,Federated learning,Training,Industries,Artificial intelligent,consumer healthcare,digital twin,edge computing,federated learning,metaverse,privacy-preservation,split learning
AI 理解论文
溯源树
样例
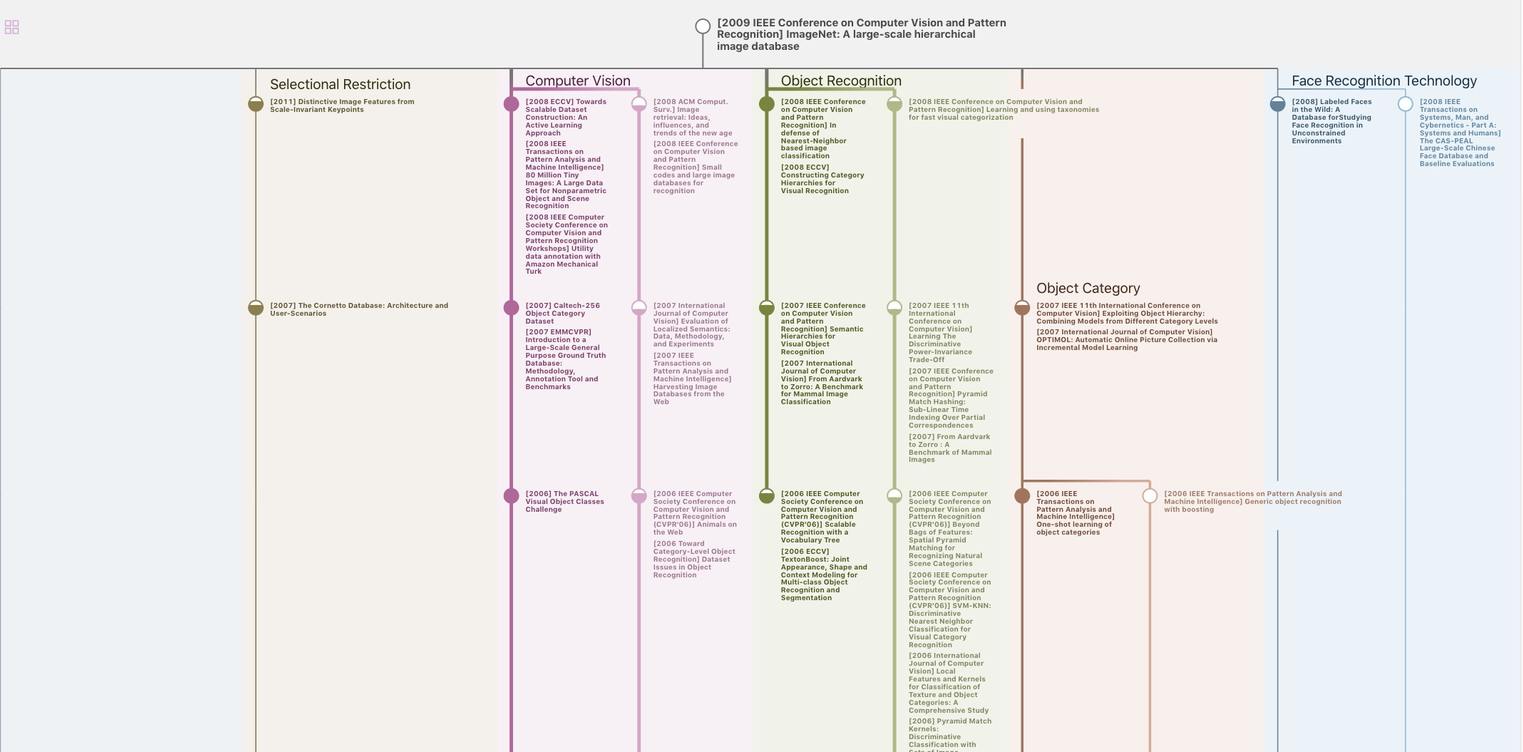
生成溯源树,研究论文发展脉络
Chat Paper
正在生成论文摘要