Enhancing TinyML-Based Container Escape Detectors With Systemcall Semantic Association in UAVs Networks
IEEE Internet of Things Journal(2024)
摘要
The adoption of lightweight container technology enables the cross-architecture deployment of Tiny Machine Learning (TinyML) models, while the implementation of container escape detectors ensures the security of both models and applications. However, a significant challenge faced by TinyML-based detectors is model aging, which leads to a substantial decline in their effectiveness as attack patterns evolve. Most existing approaches address this issue by retraining models through the labeling of new samples. However, this process can be costly and challenging to implement for updating models in resource-constrained UAVs networks. In this paper, we begin by analyzing the correlation of threat data and observe that throughout evolution, different versions of container escape attacks tend to maintain semantically identical or similar system calls. This observation prompts us to approach the model aging problem from a novel perspective: if the model can acquire knowledge of these fundamental system calls, it will be capable of effectively detecting emerging new attacks. Based on this perspective, we have developed sysE to capture system call data that remains unchanged or exhibits similarities to container escape attacks during evolution. This augmentation complements six TinyML-based detectors. Experimental results obtained from a large-scale evolving dataset demonstrate that our proposed approach effectively mitigates the aging rate of these models, reducing it from 7.3% to 21.5%. Additionally, it significantly decreases the labeling effort required from 28.06% to 65.47%.
更多查看译文
关键词
Container Escape,TinyML-based Detector,Model Aging,Systemcall Association
AI 理解论文
溯源树
样例
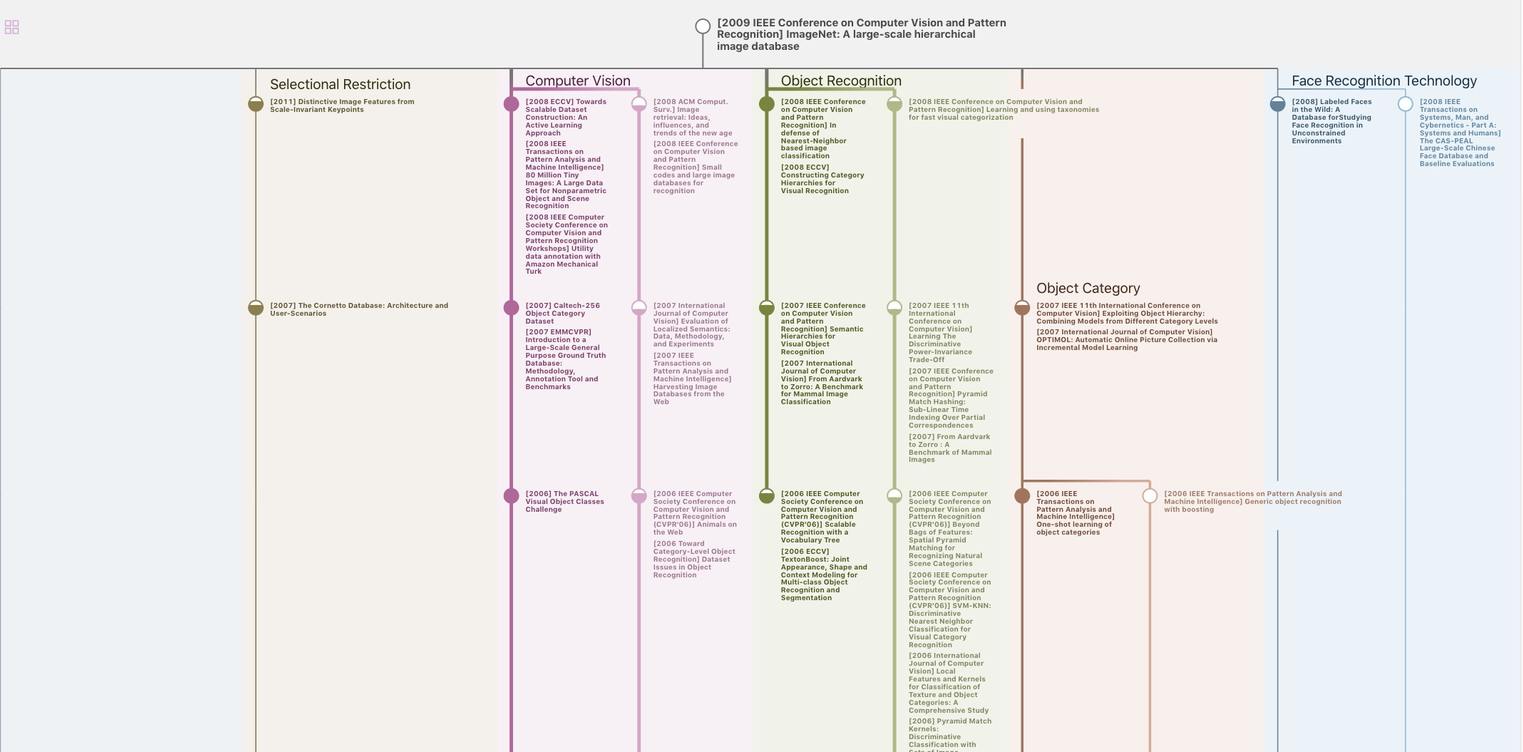
生成溯源树,研究论文发展脉络
Chat Paper
正在生成论文摘要