WVFL: Weighted Verifiable Secure Aggregation in Federated Learning
IEEE Internet of Things Journal(2024)
摘要
Federated learning has shown great potential in Internet of Things (IoTs) for performing intelligent decision making. It allows IoT devices to collaboratively train a neural network upon the data they collect while separately keeping these data staying local. However, several research works have shown that such architecture still faces security challenges that adversaries could raise inference attack to the transferring model parameters to reveal data from devices. Moreover, another security risk in federated learning is that malicious devices may launch model pollution attack to reduce the quality of the aggregated model, or dishonest server may output incorrect aggregated result to the devices. Most existing privacy-preserving federated learning protocols could not deal with both problems. In this paper, we present WVFL, a secure weighted aggregation protocol in which aims to minimize the effect of wrong local models to the aggregated model, meanwhile allowing devices to verify the correctness of the aggregation result. All important intermediate values in the process are in encrypted form so that they would not be revealed to both devices and servers to guarantee privacy. At the end of this paper, we give implementation of our WVFL scheme, showing its efficiency compared with previous work.
更多查看译文
关键词
Secure Weighted Aggregation,Verifiability,Secure Multi-Party Computation,Federated Learning
AI 理解论文
溯源树
样例
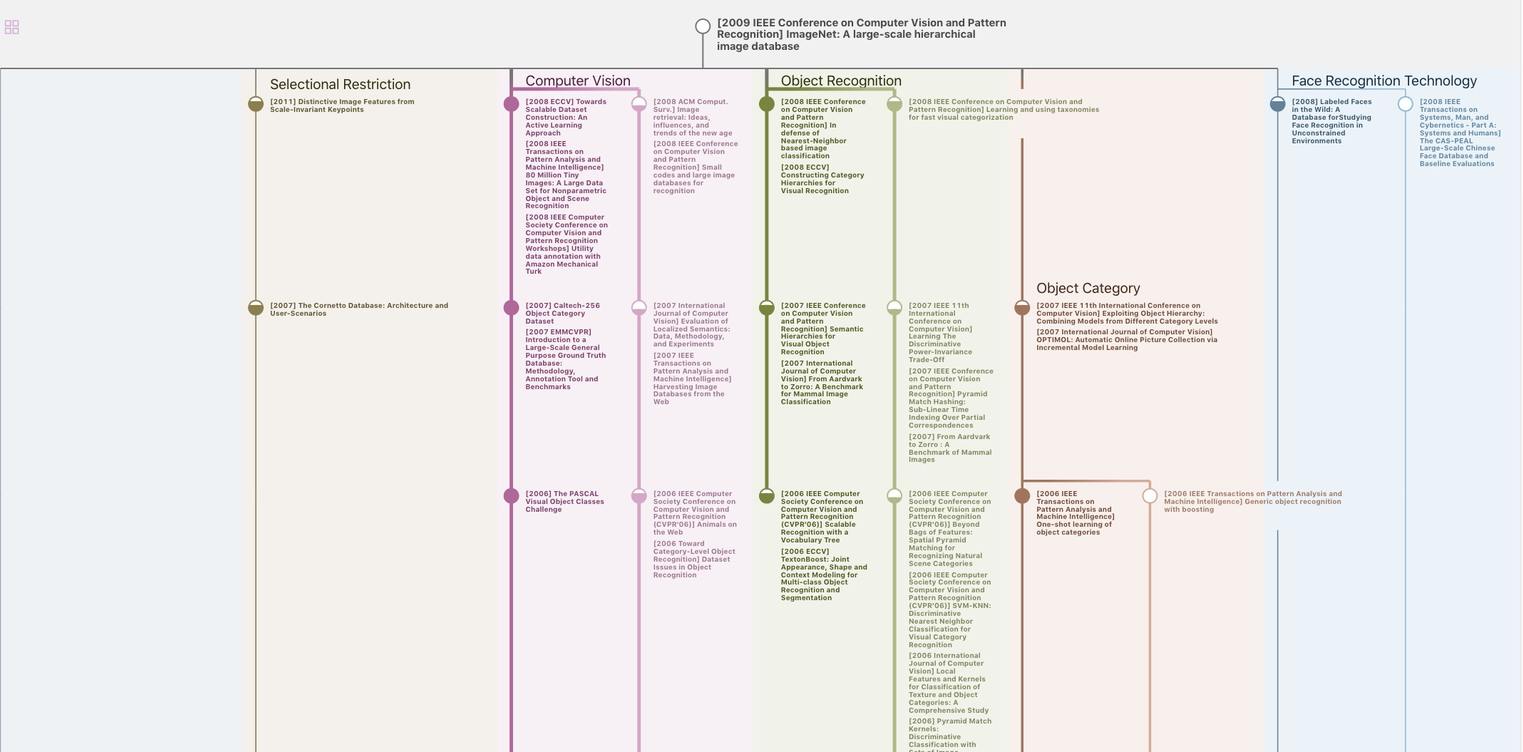
生成溯源树,研究论文发展脉络
Chat Paper
正在生成论文摘要