Decouple and Decorrelate: A Disentanglement Security Framework Combing Sample Weighting for Cross-Institution Biased Disease Diagnosis
IEEE Internet of Things Journal(2024)
摘要
There is an urgent need to address the effective diagnosis of multiple diseases across various medical institutions while ensuring the privacy of medical data in IoT environments. This requires the model to have the ability of zero-shot generalization, which can not be satisfied by existing models. To address this issue, we propose a two-stage model for medical image diagnosis, based on decoupling and decorrelating. An adversarial architecture is built using a gradient reversal discriminator to improve the model’s robustness. To further address the mixed correlation within domain-invariant features achieved by disentanglement, we propose to mitigate feature dependency through sample weighting. The effectiveness of the model is validated using both the diabetic retinopathy and the skin lesion datasets. For cross-dataset experiment, we select two datasets for symmetric decoupling and reserve the remaining dataset as the test set. The test dataset is analogous to real-world scenarios, where all the samples and labels are completely unknown to the model. The experiments show that the model achieves excellent performance and outperforms baselines in most metrics, which demonstrate the effectiveness of our approach to address the issue of multi-center data privacy in IoT, with a focus on enhancing diagnostic accuracy while ensuring data security.
更多查看译文
关键词
Domain-invariant,Security,Adversarial,Cross-domain Robustness
AI 理解论文
溯源树
样例
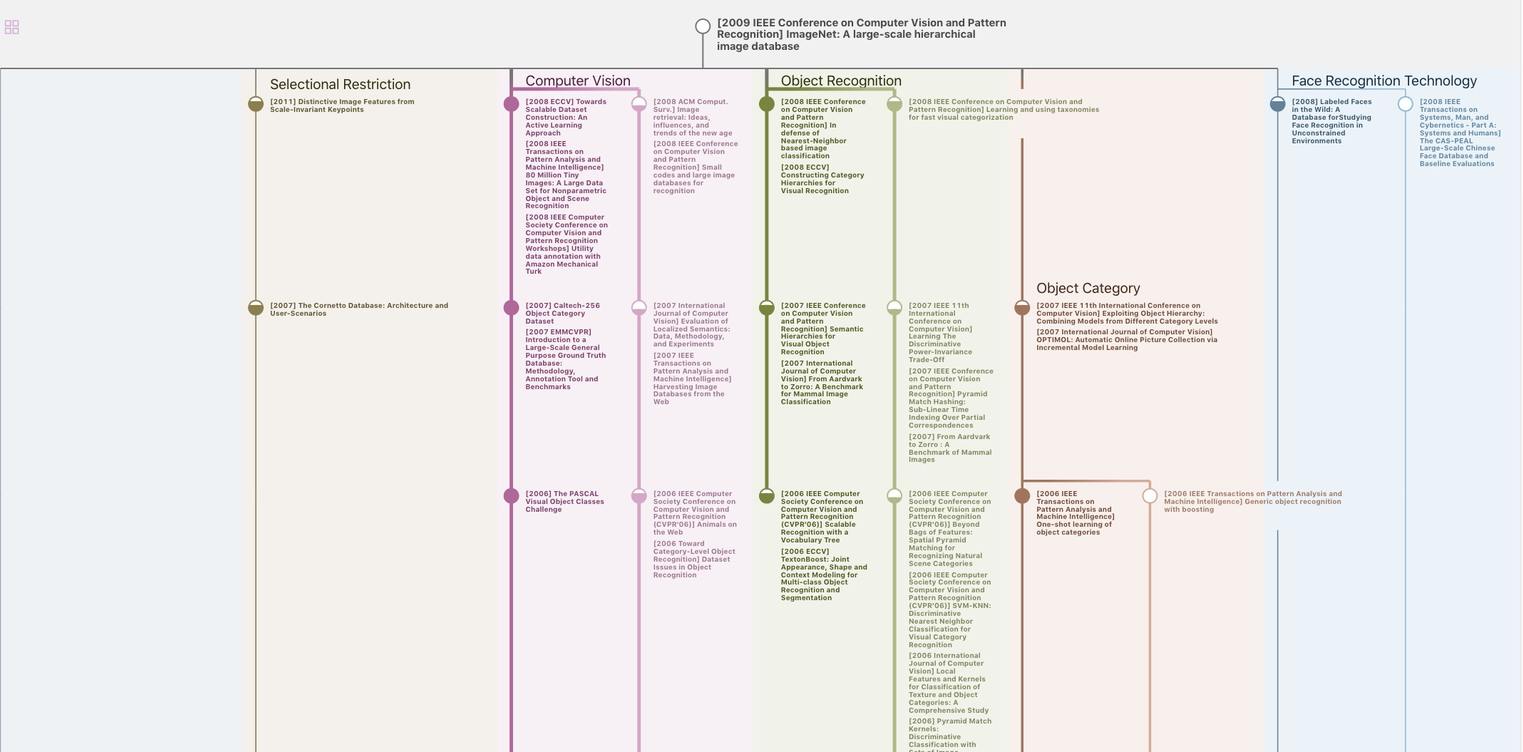
生成溯源树,研究论文发展脉络
Chat Paper
正在生成论文摘要