RobLoc: Robust Wireless Localization With Dynamic Self-Adaptive Learning
IEEE Internet of Things Journal(2024)
摘要
Recently, deep-learning-based wireless localization with fingerprinting has gained significant accuracy improvement. However, mainstream schemes cannot overcome the vulnerability of RF signals with complicated environmental dynamics which extremely exacerbates localization accuracy and severely limits widespread practical applications. To address this issue, we propose RobLoc, a novel approach of robust localization which can acquire environmental adaptability to achieve accurate location estimations. RobLoc first exploits dimensionality reduction to explore the geometric structure underlying RF fingerprints in the Grassmann manifold which possesses a stable spatial correlation with the environmental dynamics. Furthermore, we design the manifold embedded dynamic adaptation network to perform both global and local distribution matching and further quantitatively self-evaluate their respective contributions for automatic radio map adaptation. According to such dynamic self-adaptive learning, RobLoc can learn finer environment-independent representation by joint geometrical and statistical alignment to attain the effective enhancement of channel knowledge transfer across different environments. Finally, extensive experimental results in many typical indoor scenarios demonstrate that the proposed RobLoc system can achieve better localization performance than advanced works, facilitating fingerprint-based localization for fully practical LBS with a wide range of deployments.
更多查看译文
关键词
Indoor localization,fingerprinting,radio map adaptation,dynamic distribution adaptation
AI 理解论文
溯源树
样例
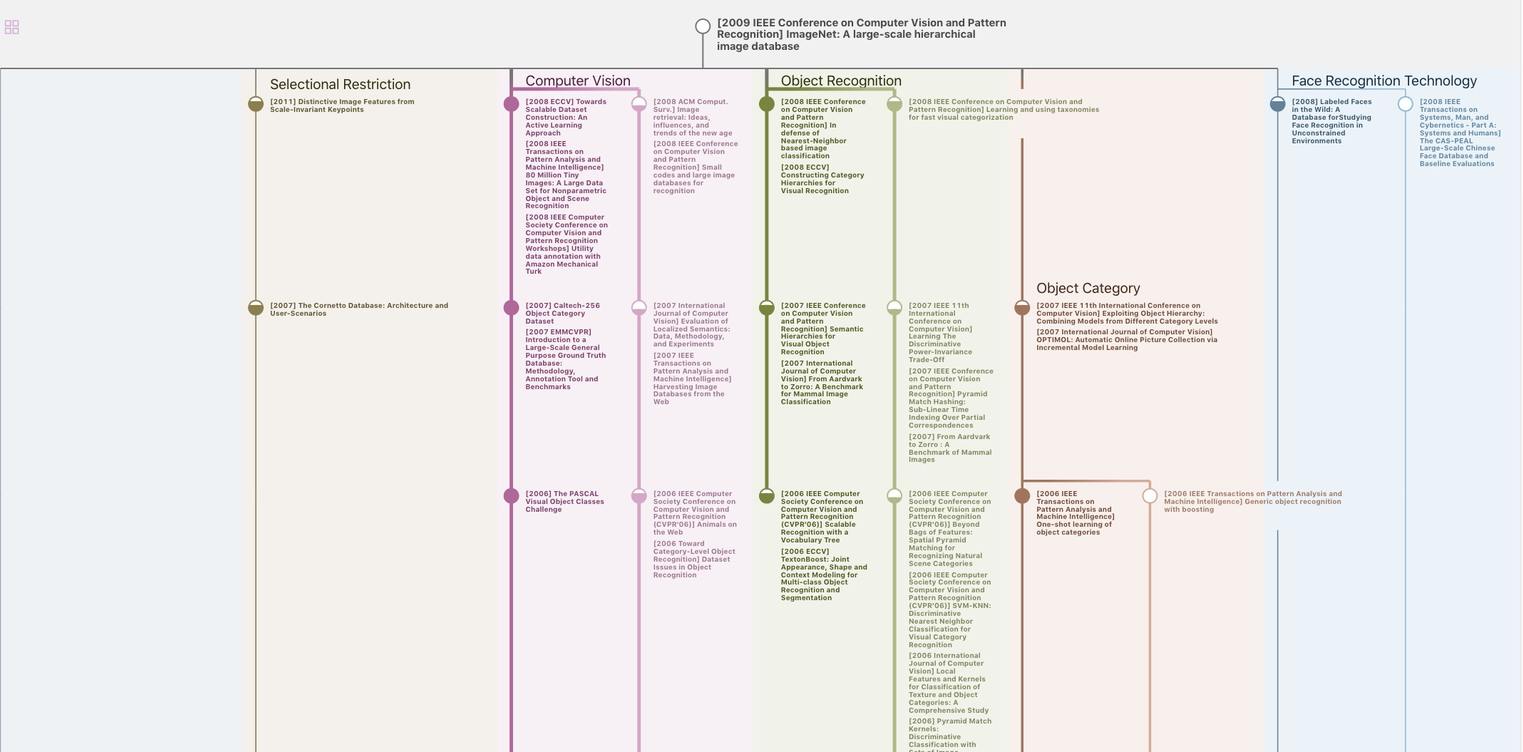
生成溯源树,研究论文发展脉络
Chat Paper
正在生成论文摘要