Energy Consumption Optimization of UAV-Assisted Traffic Monitoring Scheme With Tiny Reinforcement Learning
IEEE Internet of Things Journal(2024)
摘要
Unmanned Aerial Vehicles (UAVs) can capture pictures of road conditions in all directions and from different angles by carrying high-definition cameras, which helps gather relevant road data more effectively. However, due to their limited energy capacity, drones face challenges in performing related tasks for an extended period. Therefore, a crucial concern is how to plan the path of UAVs and minimize energy consumption. To address this problem, we propose a multi-agent deep deterministic policy gradient-based (MADDPG) algorithm for UAV path planning (MAUP). Considering the energy consumption and memory usage of MAUP, we have conducted optimizations to reduce consumption on both fronts. Firstly, we define an optimization problem aimed at reducing UAV energy consumption. Secondly, we transform the defined optimization problem into a reinforcement learning problem and design MAUP to solve it. Finally, we optimize energy consumption and memory usage by reducing the number of neurons in the hidden layer of MAUP and conducting fine-grained pruning on connections. The final simulation results demonstrate that our method effectively reduces the energy consumption of UAVs compared to other methods.
更多查看译文
关键词
UAV Path Planning,Energy Consumption Optimization,Multi-Agent Reinforcement Learning,Tiny Machine Learning,MADDPG
AI 理解论文
溯源树
样例
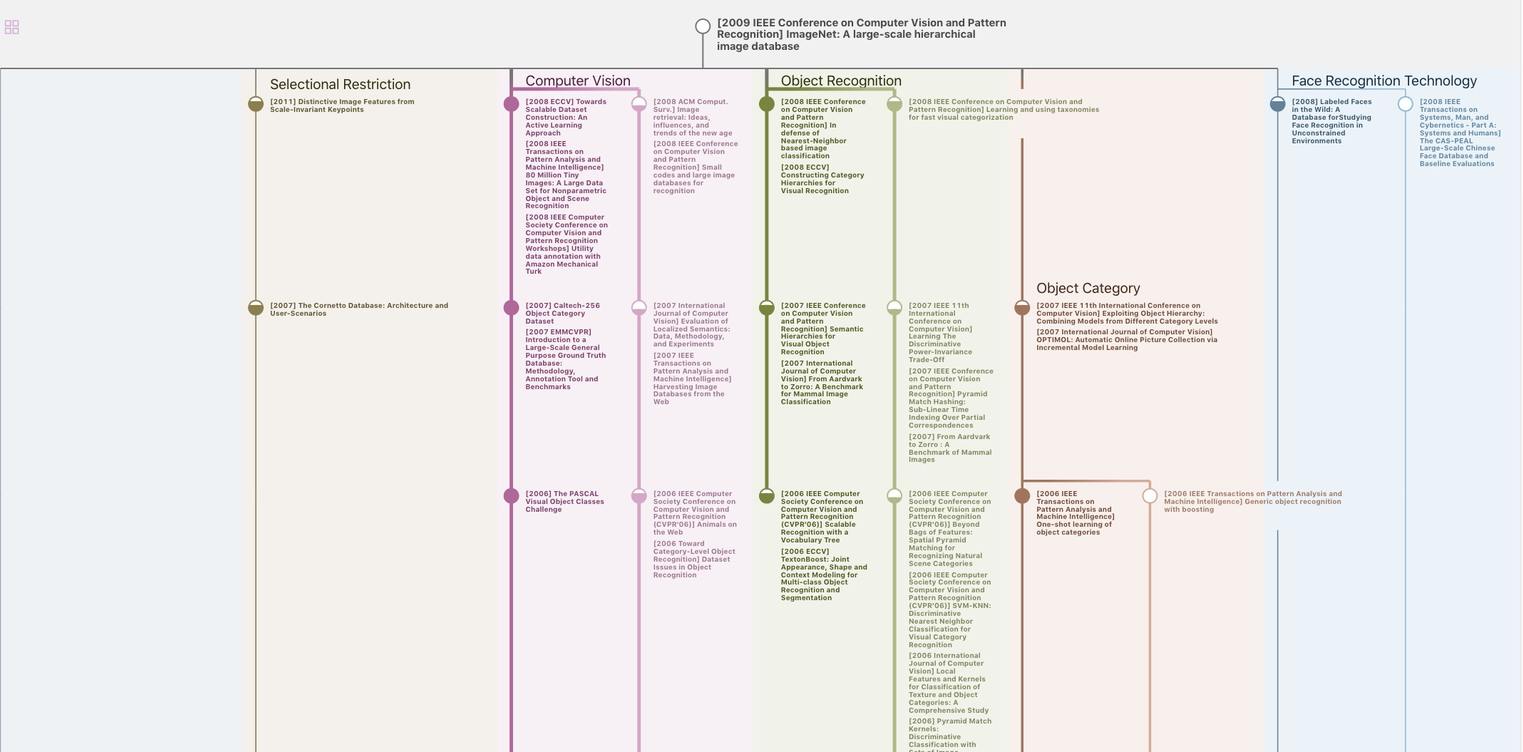
生成溯源树,研究论文发展脉络
Chat Paper
正在生成论文摘要