Artificial Neural Networks With Fast Transfer Learning for Statistical Signal Integrity Analysis of MWCNT and MLGNR Interconnect Networks
IEEE TRANSACTIONS ON ELECTROMAGNETIC COMPATIBILITY(2024)
摘要
In this article, artificial neural network (ANN) metamodels have been developed for the fast statistical signal integrity analysis of multiwalled carbon nanotube and multilayer graphene nanoribbon interconnect networks. These ANN metamodels, referred to as primary ANNs, are trained using transfer learning strategies where the initial guess of the weights and bias terms are learned from a pretrained secondary ANN. The secondary ANN is trained using data extracted from a cheap and approximate equivalent single conductor model of the interconnects. Starting with this informed initial guess, the weights and bias terms of the primary ANN can be optimized using a very small dataset generated from the rigorous but massive multiconductor circuit model of the interconnects. This makes the primary ANN significantly more efficient to train than existing metamodels. Two distinct transfer learning strategies based on the full and partial transfer of knowledge between the primary and secondary ANNs have been developed in this work.
更多查看译文
关键词
Artificial neural networks (ANNs),multilayer graphene nanoribbons (MLGNRs),multiwalled carbon nanotubes (MWCNTs),on-chip interconnects,signal integrity analysis,statistical analysis,transfer learning
AI 理解论文
溯源树
样例
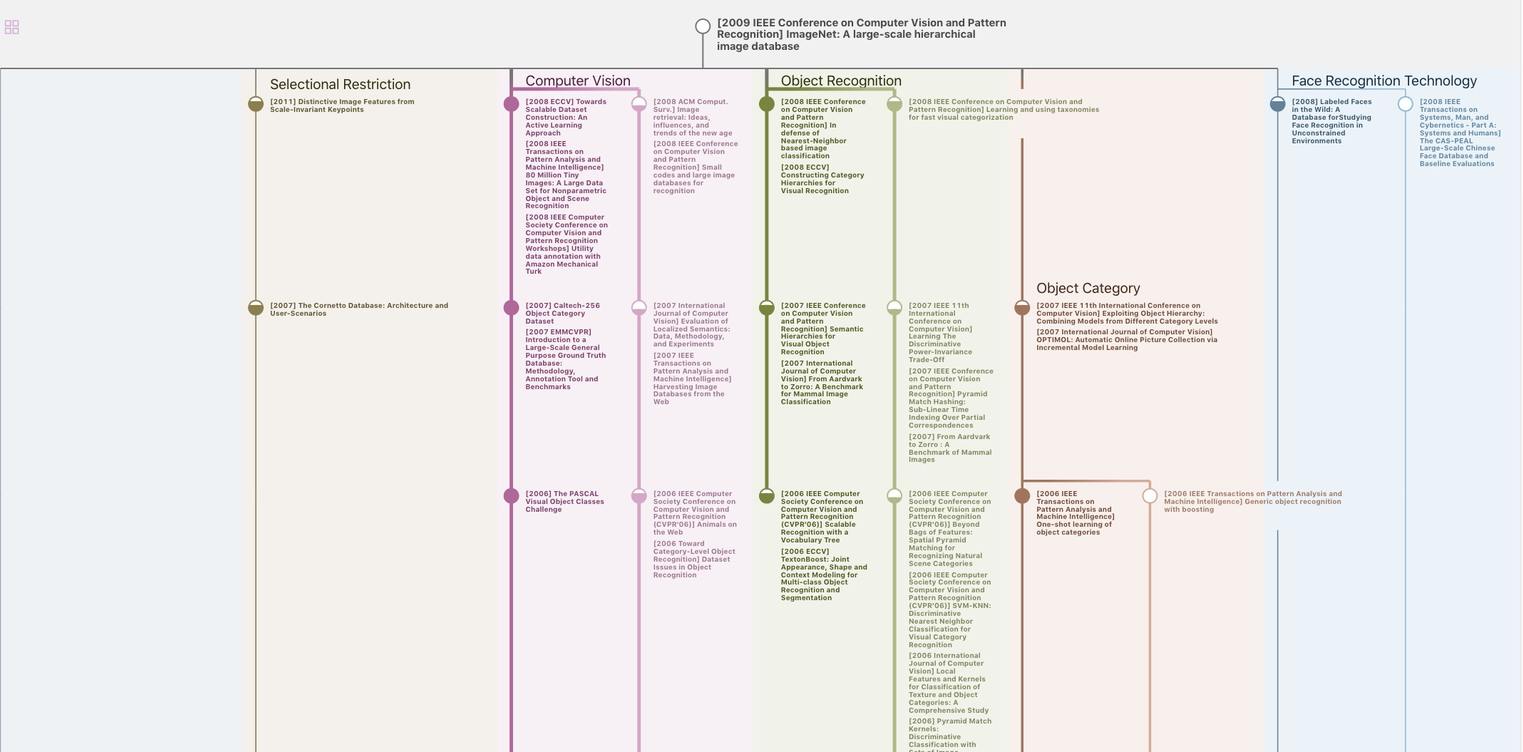
生成溯源树,研究论文发展脉络
Chat Paper
正在生成论文摘要