Analysing Performances of DL-Based ECG Noise Classification Models Deployed in Memory-Constraint IoT-Enabled Devices
IEEE Transactions on Consumer Electronics(2024)
摘要
Electrocardiogram (ECG) is the most commonly observed signal for monitoring cardiovascular activities. Recent advances in wearable technology resulted in easy, comfortable and continuous acquisition of ECG signals in non-clinical settings. One of the most important concerns with wearable ECG sensors is noise sensitivity, which affects the precision and dependability of the recorded signals. Recently, deep learning (DL)-based ECG noise detection is widely used to limit the impact of noise in ECG signal and provides better signal quality. However, existing studies did not investigate the deployability of the noise detection and classification model in different devices (e.g., resourceful, semi-resourceful and resource-constraint) and its effect on the performance of the deployed model. This study investigates the impact of model conversions on performances that were done for deploying developed DL-based ECG classifiers in resourceful, semi-resourceful and resource-constraint IoT-enabled devices. That said, we propose a DL-based ECG noise classifier framework to provide noise-free ECG signals on smart devices. Our results show that the VGG model gains accuracy and F1 score 97.31% and 94.18% for resourceful devices, whereas the converted DL-based model for semi-resourceful and resource-constraint devices shows 92.84% and 84.59% respectively. At the same time, the Resnet model improves the 1.34% F1 score from resourceful DL-based models. In addition, Alexnet and VGG model is not fit for resource-constraint devices due to memory limitation. The study provides a guideline for designing DL-based models based on the target IoT deployment environment, which can be followed for problems other than ECG noise classification.
更多查看译文
关键词
ECG,Deep Learning,Internet of Things,TensflowLite,Micro-controller,Classification,Performance
AI 理解论文
溯源树
样例
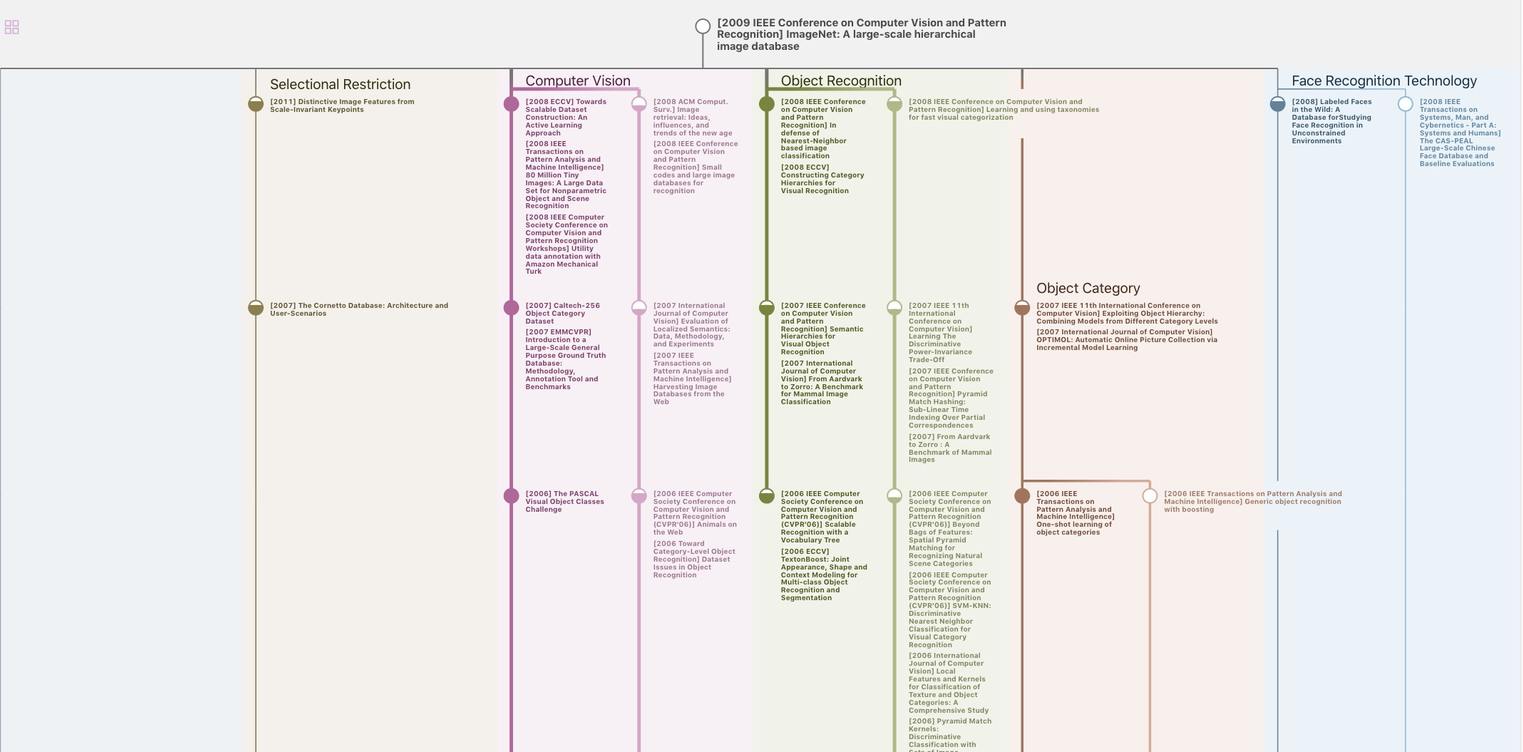
生成溯源树,研究论文发展脉络
Chat Paper
正在生成论文摘要