Accurate Modeling of GaN HEMTs Oriented to Analysis of Kink Effects in S22 and h21: An Effective Machine Learning Approach
IEEE JOURNAL OF THE ELECTRON DEVICES SOCIETY(2024)
摘要
In this work, for the first time, a machine learning behavioral modeling methodology based on gate recurrent unit (GRU) is developed and used to model and then analyze the kink effects (KEs) in the output reflection coefficient (S-22) and the short-circuit current gain (h(21)) of an advanced microwave transistor. The device under test (DUT) is a 0.25- mu m gallium nitride (GaN) high electron mobility transistor (HEMT) on silicon carbide (SiC) substrate, which has a large gate periphery of 1.5 mm. The scattering (S-) parameters of the DUT are measured at a frequency up to 65 GHz and at an ambient temperature up to 200 degrees C. The proposed model can accurately reproduce the KEs in S-22 and in h(21) , enabling an effective analysis of their dependence on the operating conditions, bias point and ambient temperature. It is worth noticing that the proposed transistor model shows also good performance in both interpolation and extrapolation test.
更多查看译文
关键词
GaN HEMT,GRU,kink effect,machine learning methods,semiconductor device modeling,scattering parameter measurements,temperature
AI 理解论文
溯源树
样例
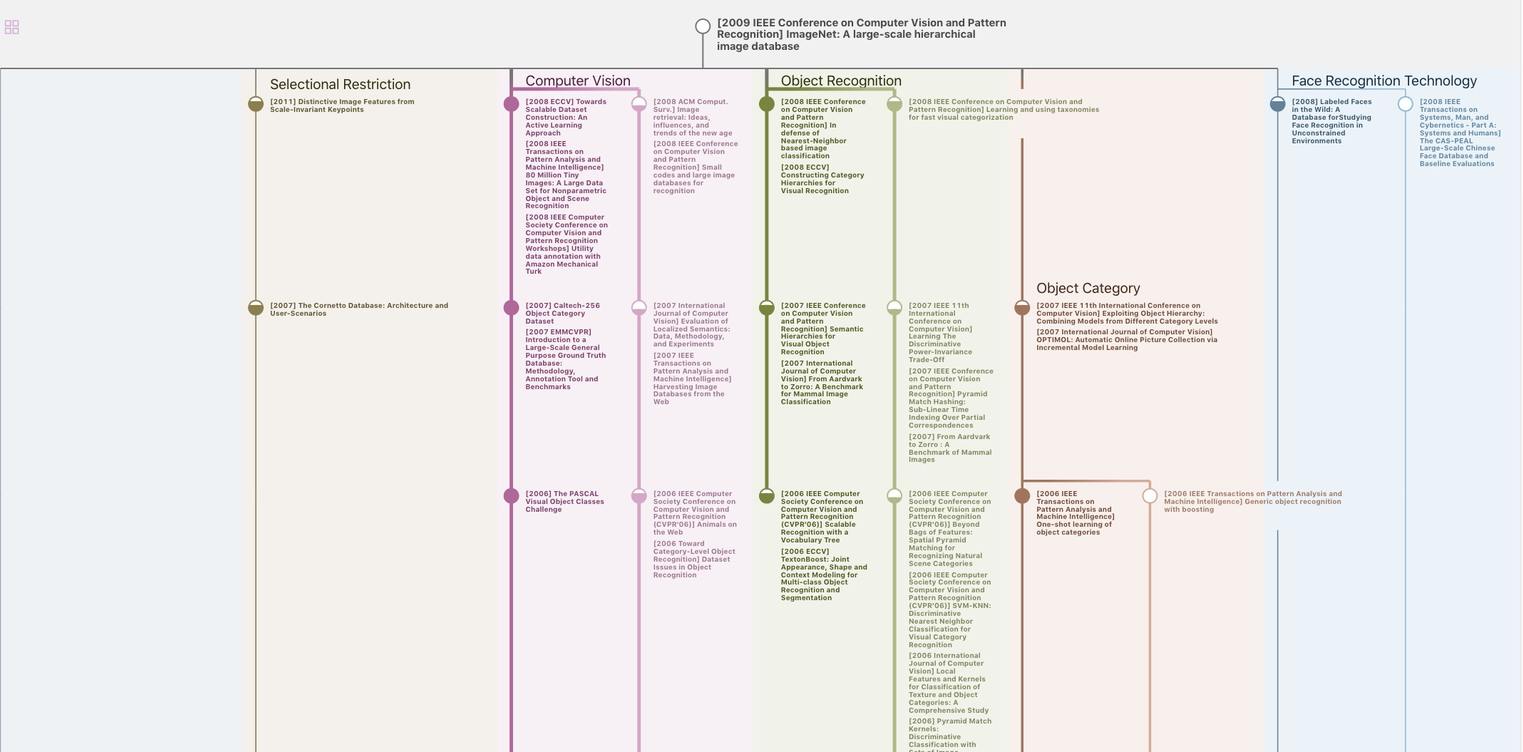
生成溯源树,研究论文发展脉络
Chat Paper
正在生成论文摘要