A Fuzzy Graph Convolutional Network Model for Sentence-Level Sentiment Analysis
IEEE TRANSACTIONS ON FUZZY SYSTEMS(2024)
摘要
Sentiment analysis in the text plays a more and more significant role in many systems, e.g., sentence-level sentiment analysis (SLSA) in recommender and decision-making systems. Various methods have been developed to improve the performance of SLSA, the newest as graph convolutional networks (GCNs)-based methods with promising accuracy. However, it often happens that many sentences in the text contain high ambiguity of sentiment. GCNs are not capable of capturing these inherent ambiguities with performance. Meanwhile, the fuzzy logic theory can improve knowledge representation under uncertainty. These facts motivate us to propose a novel SLSA method by integrating fuzzy logic into GCNs, called the fuzzy graph convolutional network (FGCN). In this novel model, the BERT+BiLSTM model is first used to convert sentences into a matrix of contextualized vectors. Second, the fuzzy membership function is integrated into the contextualized matrix to transform it into the fuzzy contextualized representation. Third, the sentence adjacency matrix combines the syntactic information extracted from the dependency tree. Fourth, the fuzzy membership function is continuously used to transform the sentence adjacency matrix into the fuzzy adjacency matrix. After that, the defuzzy membership function is used to transform the fuzzy adjacency matrix to continuous values before deriving significant features. Next, the fuzzy adjacency matrix and the fuzzy contextualized representation are concatenated to create the final representation and fed into GCN layers to capture the high-level features of the sentence. Finally, the sentiment classifier is constructed to learn the output distribution by applying the softmax function over the final representation. Unlike conventional GCNs, the FGCN integrates fuzzy membership functions into graph convolutional layers to reduce the ambiguities of sentiment in sentence representation. This enables to achieve efficiently extracting high level sentiment features in sentences. The experimental results on benchmark datasets prove that the FGCN can enhance the performance in terms of accuracy and $F_{1}$ score of SLSA in comparison with the state-of-the-art methods.
更多查看译文
关键词
Fuzzy graph convolutional network (FGCN),fuzzy logic,graph convolutional network (GCN),sentiment analysis
AI 理解论文
溯源树
样例
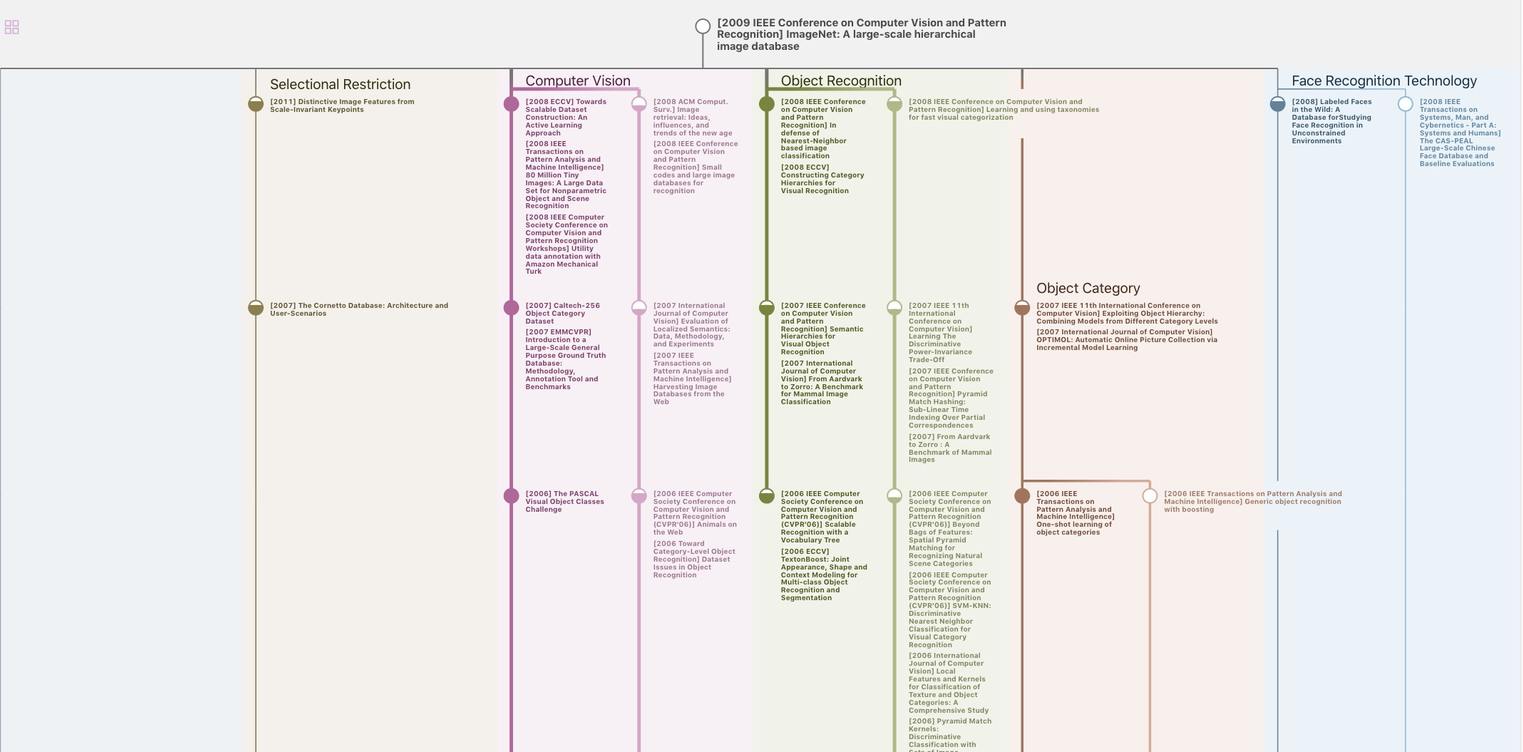
生成溯源树,研究论文发展脉络
Chat Paper
正在生成论文摘要