PIN: Sparse Aperture ISAR Imaging via Self-Supervised Learning
IEEE GEOSCIENCE AND REMOTE SENSING LETTERS(2024)
摘要
The sparse aperture (SA) phenomenon, when encountered in the context of inverse synthetic aperture radar (ISAR) imaging, poses a formidable challenge in acquiring high-resolution ISAR images to establish ground truth. This challenge imposes limitations on the practical implementation of data-driven SA-ISAR imaging methods. In response to this issue, we introduce a novel deep learning approach called Parallel ISTA Net (PIN) to enhance the quality of SA-ISAR imaging and reduce the reliance on high-quality labeled data. The model is an interpretable deep unfolding model that achieves self-supervised training through a parallel architecture. The PIN model uses a multisampling matrix training strategy to enhance the robustness of the network through the symmetry constraints provided by the parallel framework. In addition, we introduce a weighting factor to adjust the loss function to improve imaging quality further. Real-measured data imaging results show that this method can achieve robust imaging performance comparable to supervised methods at low sampling rates of 50% and 25%.
更多查看译文
关键词
Imaging,Radar imaging,Training,Image reconstruction,Apertures,Pins,Sparse matrices,Compressive sensing (CS),inverse synthetic aperture radar (ISAR),self-supervised,sparse aperture (SA)
AI 理解论文
溯源树
样例
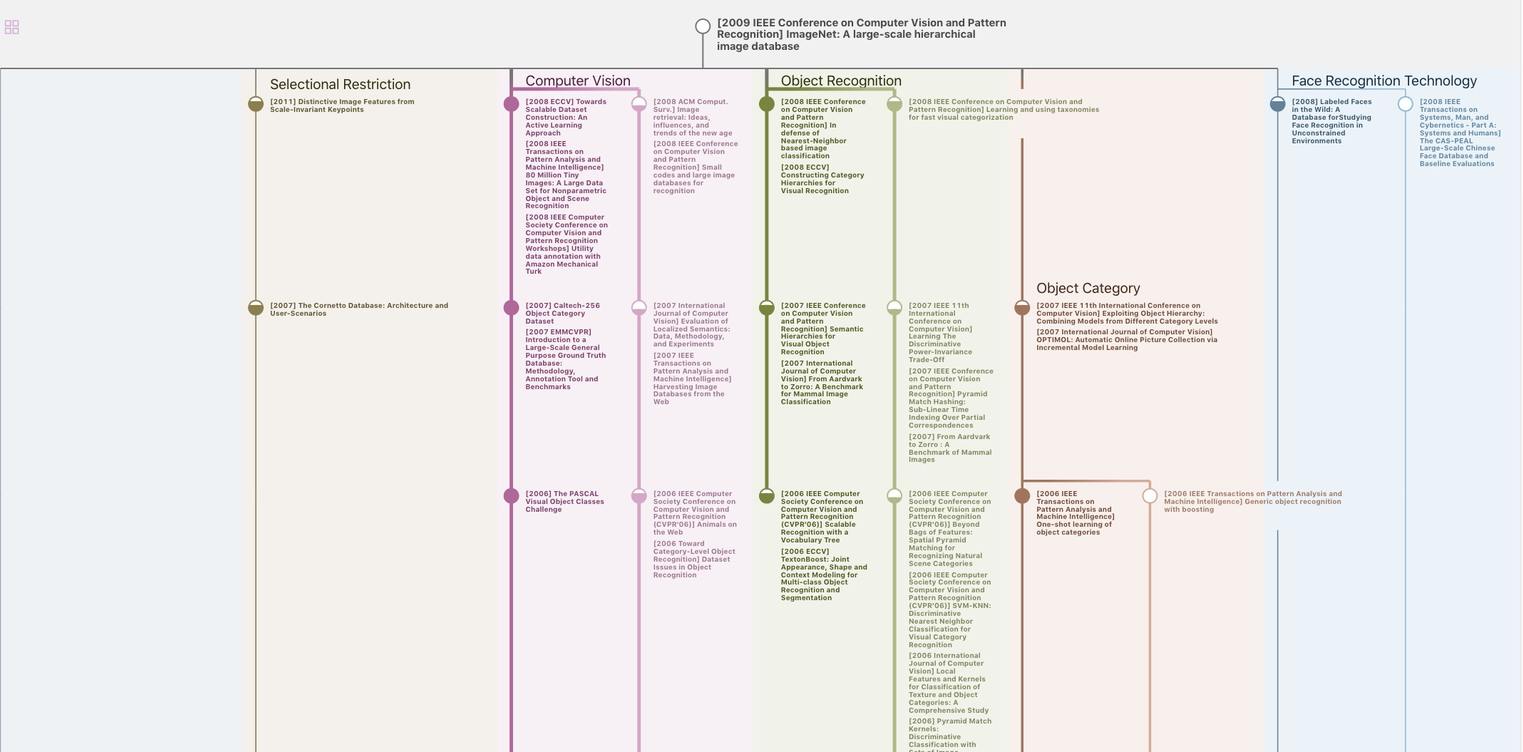
生成溯源树,研究论文发展脉络
Chat Paper
正在生成论文摘要