Feature Generating Network With Attribute-Consistency for Zero-Shot Fault Diagnosis
IEEE TRANSACTIONS ON INDUSTRIAL INFORMATICS(2024)
摘要
The absence of fault data in certain categories presents a significant challenge in data-driven fault diagnosis, as obtaining a complete fault dataset is often unfeasible. Zero-shot learning has emerged as a viable solution to this problem. Nonetheless, it often encounters problem of unreliable diagnosis results due to domain shift. In this article, a feature generating network with attribute-consistency is developed for zero-shot fault diagnosis, which introduces the attribute consistency constraint and feature transformation with attribute information. The implementation process comprises two parts, unseen fault class generation and discriminative feature transformation. The attribute consistency constraint adopted in data generation can make the generated data represent their attribute well. For feature transformation, a concatenation operation is used to transforming the generated samples into more discriminative representations. The effectiveness of the proposed method is verified using a public dataset for fault diagnosis purpose. Results indicate that the proposed method outperforms the state-of-art zero-shot diagnosis method.
更多查看译文
关键词
Fault diagnosis,Training,Feature extraction,Generative adversarial networks,Generators,Task analysis,Informatics,Attribute consistency,feature transformation,generating network,zero-shot fault diagnosis
AI 理解论文
溯源树
样例
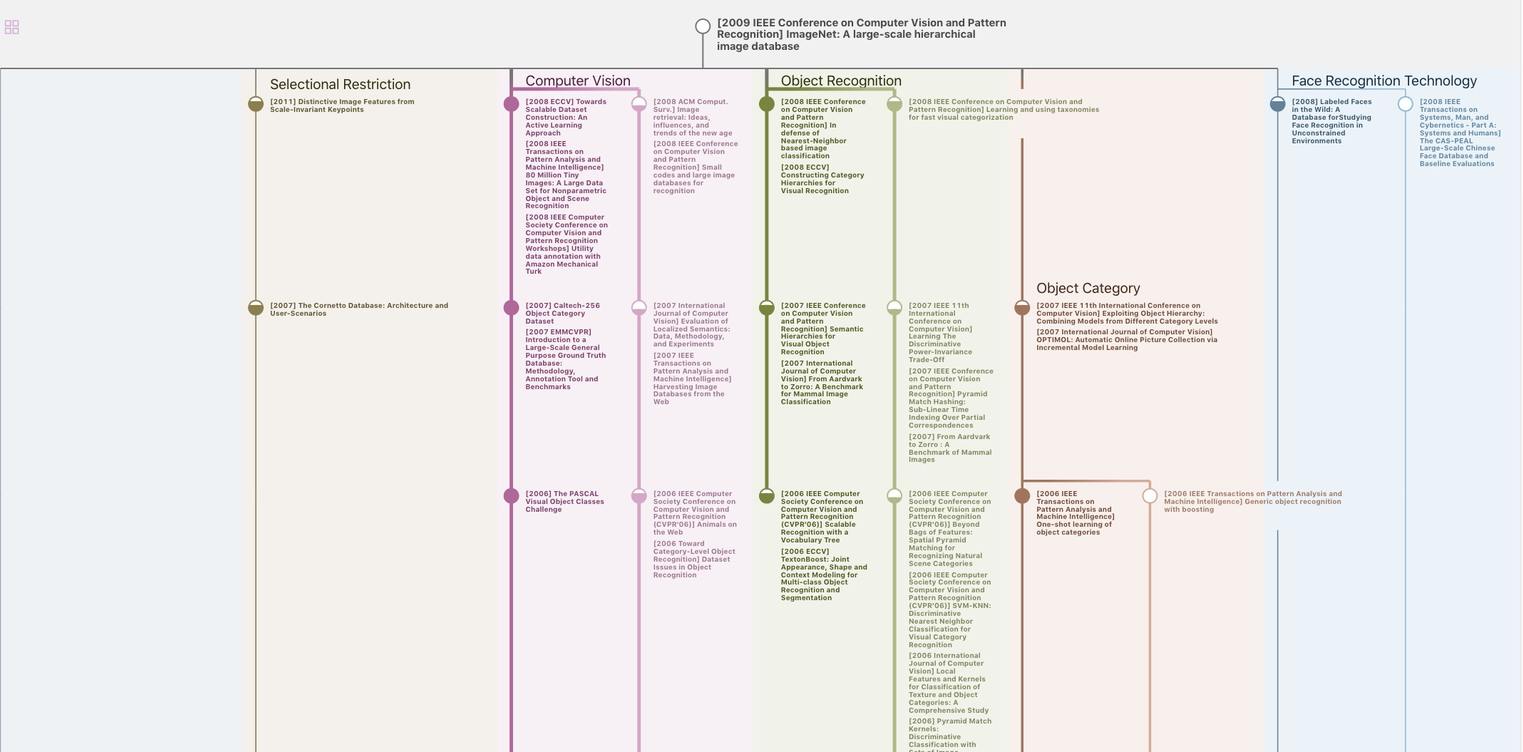
生成溯源树,研究论文发展脉络
Chat Paper
正在生成论文摘要