Optimized Clinical Feature Analysis for Improved Cardiovascular Disease Risk Screening
IEEE Open Journal of Engineering in Medicine and Biology(2024)
摘要
Objective: To develop a clinical decision support tool that can predict cardiovascular disease (CVD) risk with high accuracy while requiring minimal clinical feature input, thus reducing the time and effort required by clinicians to manually enter data prior to obtaining patient risk assessment. Results: In this study, we propose a robust feature selection approach that identifies five key features strongly associated with CVD risk, which have been found to be consistent across various models. The machine learning model developed using this optimized feature set achieved state-of-the-art results, with an AUROC of 91.30%, sensitivity of 89.01%, and specificity of 85.39%. Furthermore, the insights obtained from explainable artificial intelligence techniques enable medical practitioners to offer personalized interventions by prioritizing patient-specific high-risk factors. Conclusion: Our work illustrates a robust approach to patient risk prediction which minimizes clinical feature requirements while also generating patient-specific insights to facilitate shared decision-making between clinicians and patients.
更多查看译文
关键词
cardiovascular disease,feature interpretation,machine learning,personalized medicine,risk prediction
AI 理解论文
溯源树
样例
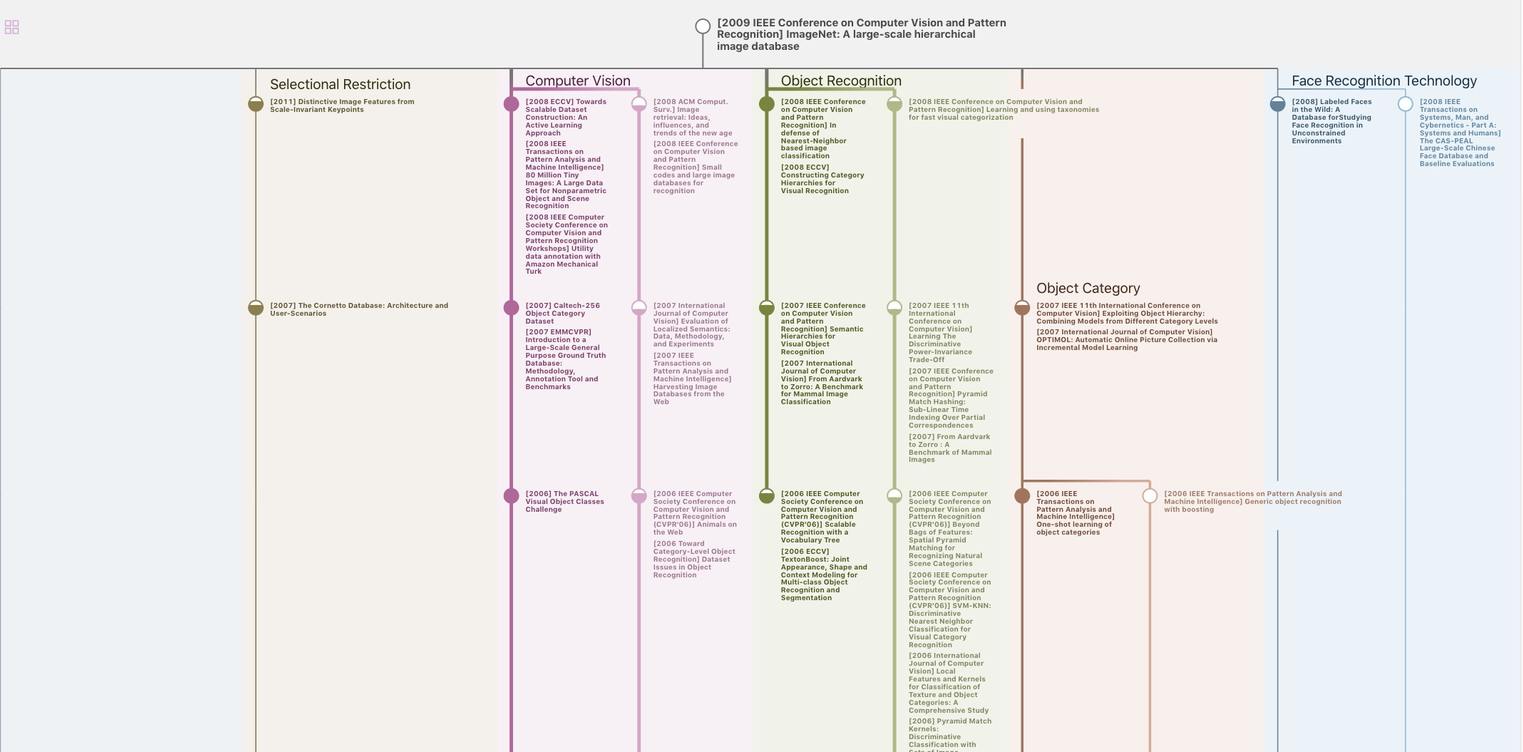
生成溯源树,研究论文发展脉络
Chat Paper
正在生成论文摘要