HmmSeNet: A Novel Single Domain Generalization Equipment Fault Diagnosis Under Unknown Working Speed Using Histogram Matching Mixup
IEEE TRANSACTIONS ON INDUSTRIAL INFORMATICS(2024)
摘要
Equipments regularly change working speeds during real-time production owing to process requirements. Applying deep learning models trained in a single speed domain straightforwardly to other unknown speed domains is extremely challenging single-domain generalization problem. Therefore, this article proposes a histogram matching mixup based sequential embedding network (HmmSeNet) for single-domain generalization of intelligent fault diagnosis under unknown speeds. HmmSeNet consists of four components: histogram matching mixup (HMM); sequential embedding (SE); separable convolution; and decision making. First, inspired by histogram matching and Mixup, the HMM data augmentation method is proposed. HMM is capable of synthesizing data with the same semantic information, but different distributions from a single source domain data during the training process, thus augmenting the source speed domain to the unknown speed domains. Then, SE utilizes trainable linear dimensional boosting to approximate the distribution between samples, which reduces the effect of sample amplitude distribution shifts caused by speed changes and allows the model to learn domain-invariant features. Finally, three layers of separable convolution and global average pooling are used to accomplish an accurate and robust recognition task. Experimental results on three datasets show that the proposed approach is only trained on a single speed domain, while it has good diagnostic performance on other unknown speed domains, even varying speed domains. The powerful generality and flexible deployment capability of HmmSeNet for speed changes are also demonstrated by ablation experimental analysis and dimensional analysis.
更多查看译文
关键词
Vibrations,Computational modeling,Histogram matching mixup (HMM),intelligent fault diagnosis,sequential embedding (SE),single domain generalization,unknown speed
AI 理解论文
溯源树
样例
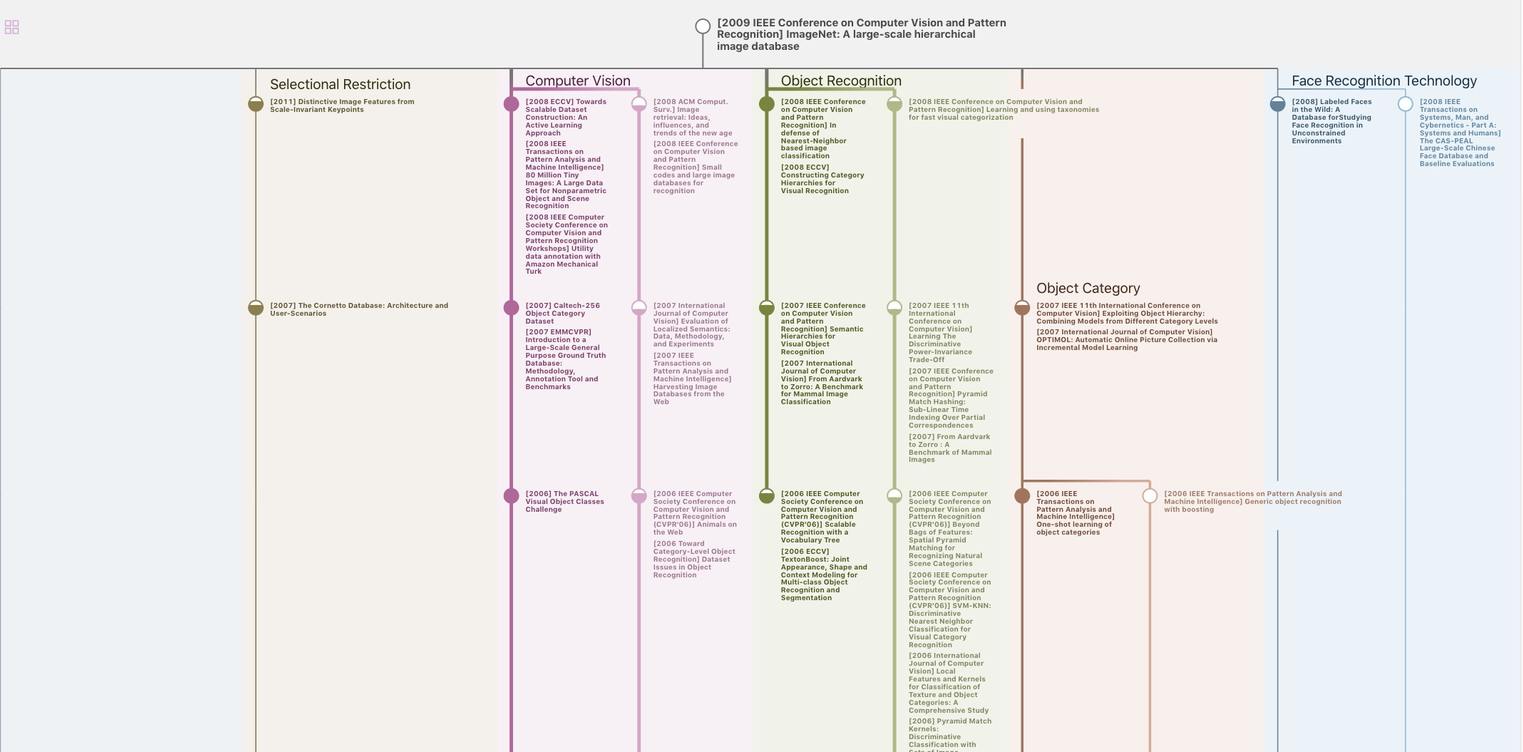
生成溯源树,研究论文发展脉络
Chat Paper
正在生成论文摘要