A Battery Prognostics and Health Management Technique Based on Knee Critical Interval and Linear Complexity Self-Attention Transformer in Electric Vehicles
IEEE TRANSACTIONS ON INTELLIGENT TRANSPORTATION SYSTEMS(2024)
摘要
Accurately estimating the remaining useful life (RUL) of lithium-ion batteries is crucial for the safe and reliable operation of batteries in electric vehicles. Due to the slow aging process and complex chemical reactions of batteries, it is challenging to obtain the complete battery lifecycle data to predict the RUL. To embrace these challenges, we propose an intelligent Transformer network with the improved self-attention mechanism based on data of the key interval area. Firstly, the knee-point and knee-onset in the capacity decay curve of the battery are identified by the Bacon-Watts model. The feature data that does not meet the minimum aging period between the knee-point and knee-onset in the aging curve is eliminated to ensure the accuracy and quickness of the prediction. Secondly, the denoising autoencoder (DAE) is used to mine the correlation between the aging characteristics in this interval and the low-dimensional hidden feature of battery aging is reconstructed. Finally, the Transformer network with improved multi-head self-attention mechanism is taken to capture the dependencies between features at different positions. A feed-forward neural network is employed to determine the weights of the features at the different sample time, which are then used in conjunction with fully connected layers and a prediction layer to estimate the remaining lifespan of lithium batteries. The proposed method is validated using the CALCE battery dataset. Simulation results show that the method achieves accurate and fast health prognosis with an average error of 0.0091 for the accuracy metric RE when only key feature interval data are used. There is also a significant advantage in computation time compared to other state-of-the-art algorithms, and our method can provide more accurate and faster health prognosis of batteries.
更多查看译文
关键词
Batteries,Aging,Degradation,Predictive models,Lithium-ion batteries,Mathematical models,Feature extraction,Lithium-ion battery,remaining useful life,knee-point and knee-onset,linear complexity self-attention,transformer network
AI 理解论文
溯源树
样例
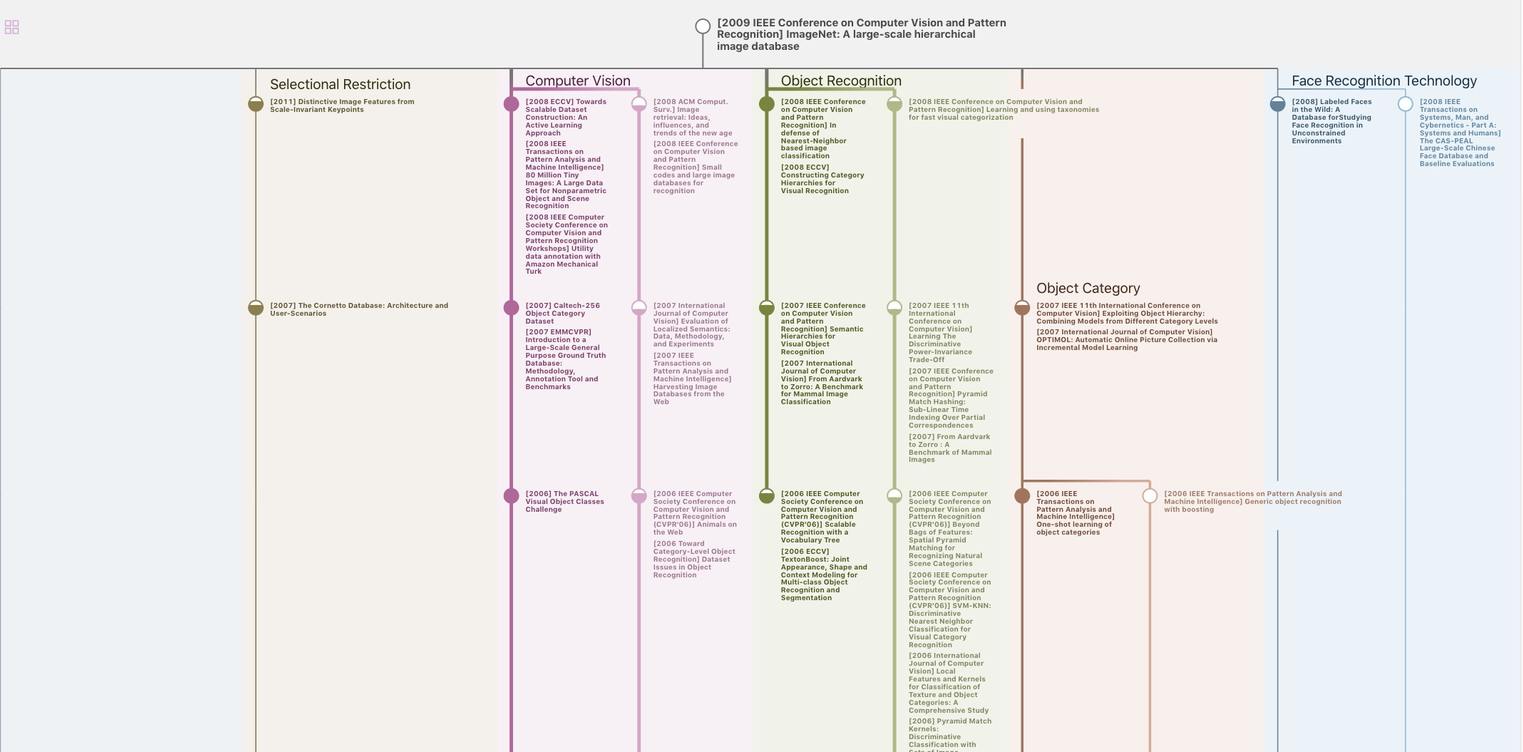
生成溯源树,研究论文发展脉络
Chat Paper
正在生成论文摘要