Double-Loop-Optimization-Based Joint Parameter Tuning for Dynamic Matrix Control With Validation in Intelligent Cigarette Drying Process
IEEE Transactions on Industrial Informatics(2024)
摘要
Dynamic matrix control (DMC) is widely used in intelligent manufacturing. Its performance is heavily affected by coupled parameters, e.g., horizons and weighting matrices. However, existing methods only focus on tuning of single type of parameter and cannot jointly tune them to improve the performance of DMC comprehensively. To bridge the gap, we propose a framework to tune DMC parameters based on double-loop-optimization jointly. The horizons of prediction and control are tuned in the outer loop, while the weighting matrices of error and control are tuned in the inner loop. An improved genetic algorithm fused with the particle swarm optimization is developed to minimize the cost function considering the constraints on horizons and weighting matrices. In the developed algorithm, population evolution and individual updating are deeply integrated to improve global optimality. The efficacy of the proposed framework is verified with real data of moisture control in cigarette drying process, which improves the quality of the moisture control by stabilizing the production equipment and reducing energy consumption.
更多查看译文
关键词
Double-loop-optimization (DLO) framework,dynamic matrix control (DMC),hybrid optimization algorithm,intelligent moisture control,joint tuning
AI 理解论文
溯源树
样例
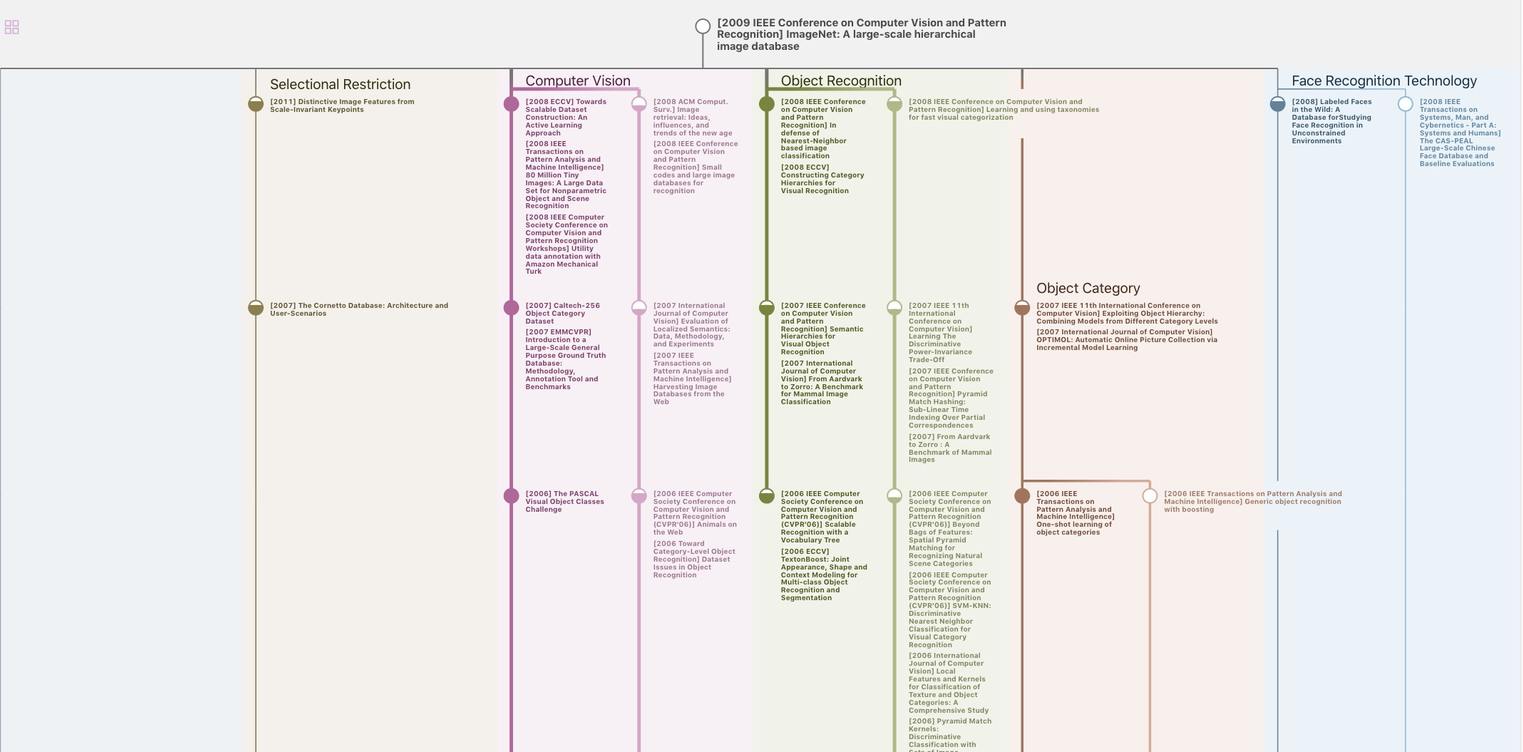
生成溯源树,研究论文发展脉络
Chat Paper
正在生成论文摘要