Personnel Trajectory Extraction From Port-Like Videos Under Varied Rainy Interferences
IEEE TRANSACTIONS ON INTELLIGENT TRANSPORTATION SYSTEMS(2024)
摘要
Large-scale deployed cameras in the automated container terminal (ACT) area helps on-site staff better identify unexpected yet emergency events by monitoring port personnel trajectories. Rainy weather is a common yet typical problem which may significantly deteriorate trajectory extraction performance. To tackle the problem, the study proposes an ensemble framework to extract personnel trajectory from port-like surveillance videos under varied rainy weather scenarios. Firstly, the proposed framework learns fine-grained personnel features with the help of the object query and transformer encoder-decoder module from the input port-like image sequences, and thus obtains port personnel locations from the input low-visibility images. Secondly, the personnel positions are further associated in a frame-by-frame manner with the help of neighboring kinematic movement information and feature information. Finally, a memory mechanism is introduced in the proposed framework to suppress personnel trajectory discontinuity outlier. In that manner, we can obtain accurate yet consistent personnel trajectories, and each person is assigned with a unique ID. We verified the proposed model performance on three port-like rainy videos involving with interferences of rain, rain streak and fog. Experimental results show that the proposed port personnel trajectory extraction framework can obtain satisfied performance considering that the average multi-target accuracy (MOTA), the average value of judging the same target (IDF1), average recall rate (IDR) and average precision (IDP) were larger than 92%.
更多查看译文
关键词
Personnel,Trajectory,Videos,Feature extraction,Meteorology,Rain,Interference,Personnel trajectory extraction,rainy interference,ensemble transformer framework,memory mechanism,automated container terminal
AI 理解论文
溯源树
样例
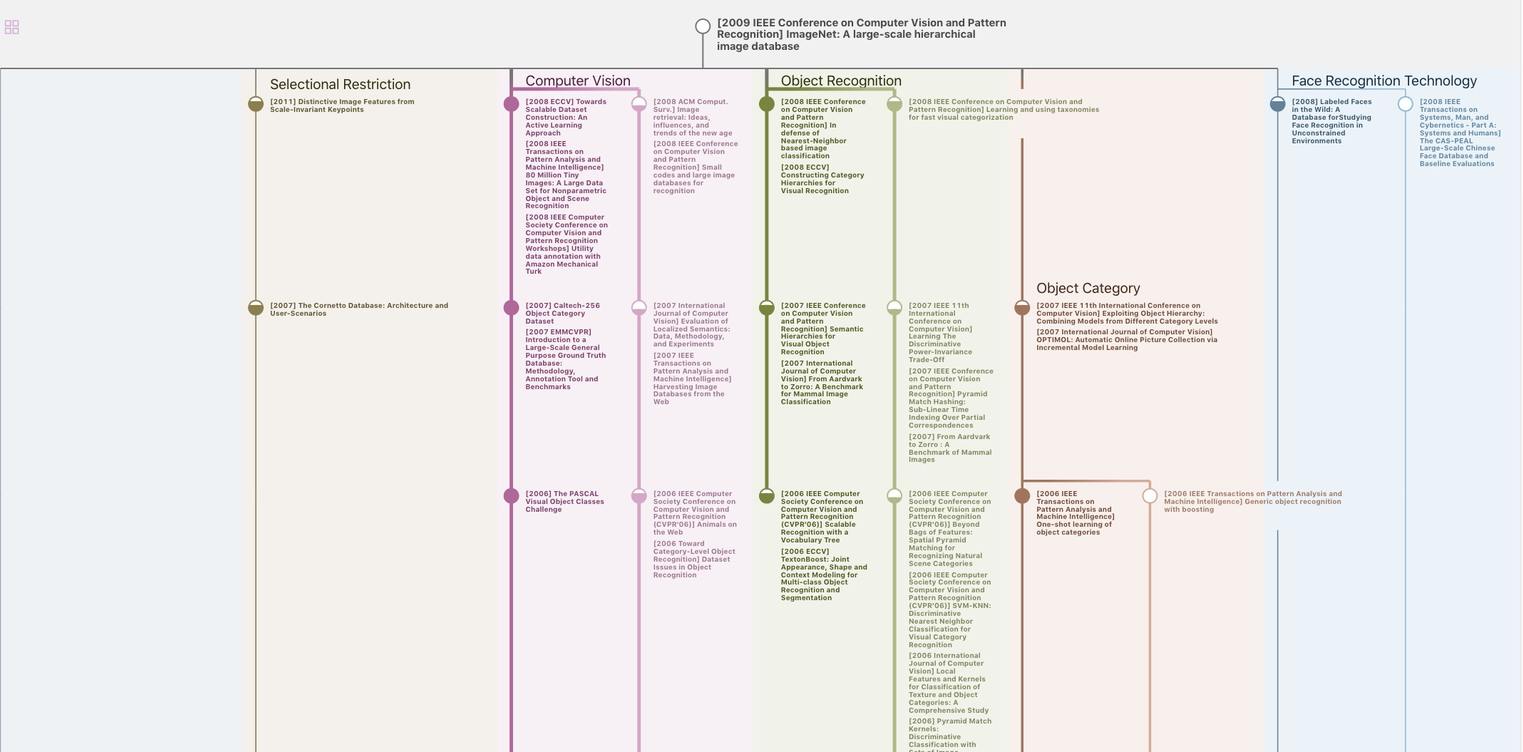
生成溯源树,研究论文发展脉络
Chat Paper
正在生成论文摘要