Seg2Sonar: A Full-Class Sample Synthesis Method Applied to Underwater Sonar Image Target Detection, Recognition, and Segmentation Tasks
IEEE TRANSACTIONS ON GEOSCIENCE AND REMOTE SENSING(2024)
摘要
To overcome the challenges of limited samples, difficult acquisition, under-representation, and labeling in utilizing sonar images and deep learning (DL) for target detection, recognition, and segmentation tasks for full-class underwater targets, we propose the Seg2Sonar network based on spatially adaptive denormalization (SPADE). This network generates images through segmentation maps, thus eliminating the need for sample annotation. Additionally, we incorporate the skip-layer channel-wise excitation (SLE) module into the SPADE network to enhance feature extraction ability with minimal training samples. To improve the realism of generated images, we introduce the focal frequency loss (FFL) module, and propose the elasticity loss (EL) strategy to improve the random combination capability of the network, considering the characteristics of low resolution and severe distortion of sonar images. Furthermore, we propose a weight adjustment (WA) strategy that tackles the challenge of low and unbalanced feature representation with few samples by taking into account the unbalanced distribution of features using prior information. These four improvements enable efficient sample augmentation of sonar images with limited samples. Building upon the improved Seg2Sonar network, we propose an underwater full-class target augmentation strategy. Based on the imaging characteristics of sonar images, we classify underwater full-class targets into four categories: texture level, group level, shape level, and intensity level. We provide the corresponding augmentation strategies by leveraging similar features among sonar target images or adding external radar/optical features to supplement the diversity of features. Our experimental results demonstrate the efficacy of our proposed method in achieving sample augmentation of underwater full-class targets with minimal samples (less than ten) or even zero samples. The approach achieves about 90% accuracy in detection, recognition, and segmentation for all types of targets through DL methods. Our findings provide a promising solution for efficient sample augmentation of underwater full-class targets with limited samples.
更多查看译文
关键词
Sonar,Image segmentation,Sonar detection,Shape,Target recognition,Image recognition,Feature extraction,Few samples,generating adversarial network (GAN),sample augmentation,sonar images,target detection,target segmentation
AI 理解论文
溯源树
样例
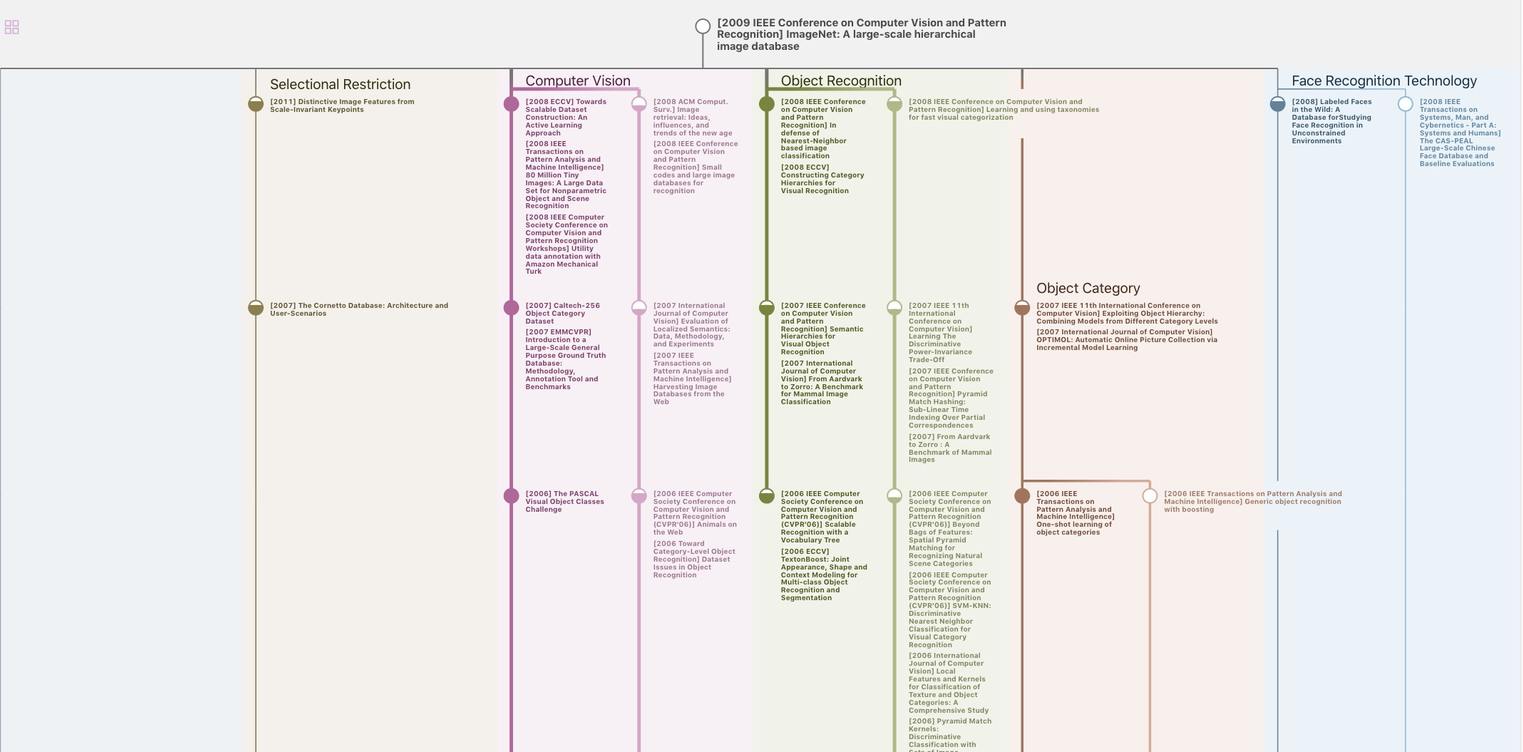
生成溯源树,研究论文发展脉络
Chat Paper
正在生成论文摘要