Data Distribution Loss for Imbalanced SAR Vehicle Target Recognition
IEEE GEOSCIENCE AND REMOTE SENSING LETTERS(2024)
摘要
The data distribution (DD) of synthetic aperture radar (SAR) vehicle targets in the actual missions is often imbalanced. However, the recent algorithms for SAR target recognition are designed either under abundant samples, or the situation of few labeled samples among all the categories. All these cases avoid facing the difficulties of imbalanced DD, that is, the difference between the number of labeled samples among categories is huge. The samples in the majority classes will get more chances to be learned by the deep neural network, which impedes the regular algorithms from achieving a high recognition rate. In this letter, a design guideline for imbalance loss and an example of DD loss based on the guideline is proposed, which provides an extremely effective way of handling the problem of imbalanced SAR target recognition. The DD loss takes the sample distribution and the data quantity of SAR vehicle targets into consideration. It can cause images with fewer samples in their categories to decrease more gradients proportionally. Moreover, the proposed DD loss adds no more burden to the networks and compared to other imbalanced algorithms with complex processes, the DD loss can be conducted easily. Plenty of experiments, which involve two various kinds of imbalanced datasets, are implemented and the proposed DD loss shows excellent performance among these imbalanced datasets. When there are only 40 labeled samples in minority categories, the DD loss can achieve over 95% in nine different cases, which exceeds other methods and losses of at least 7%.
更多查看译文
关键词
Synthetic aperture radar,Target recognition,Deep learning,Classification algorithms,Training,Testing,Mathematical models,Convolutional neural networks (CNN),imbalanced learning,loss functions,synthetic aperture radar (SAR)
AI 理解论文
溯源树
样例
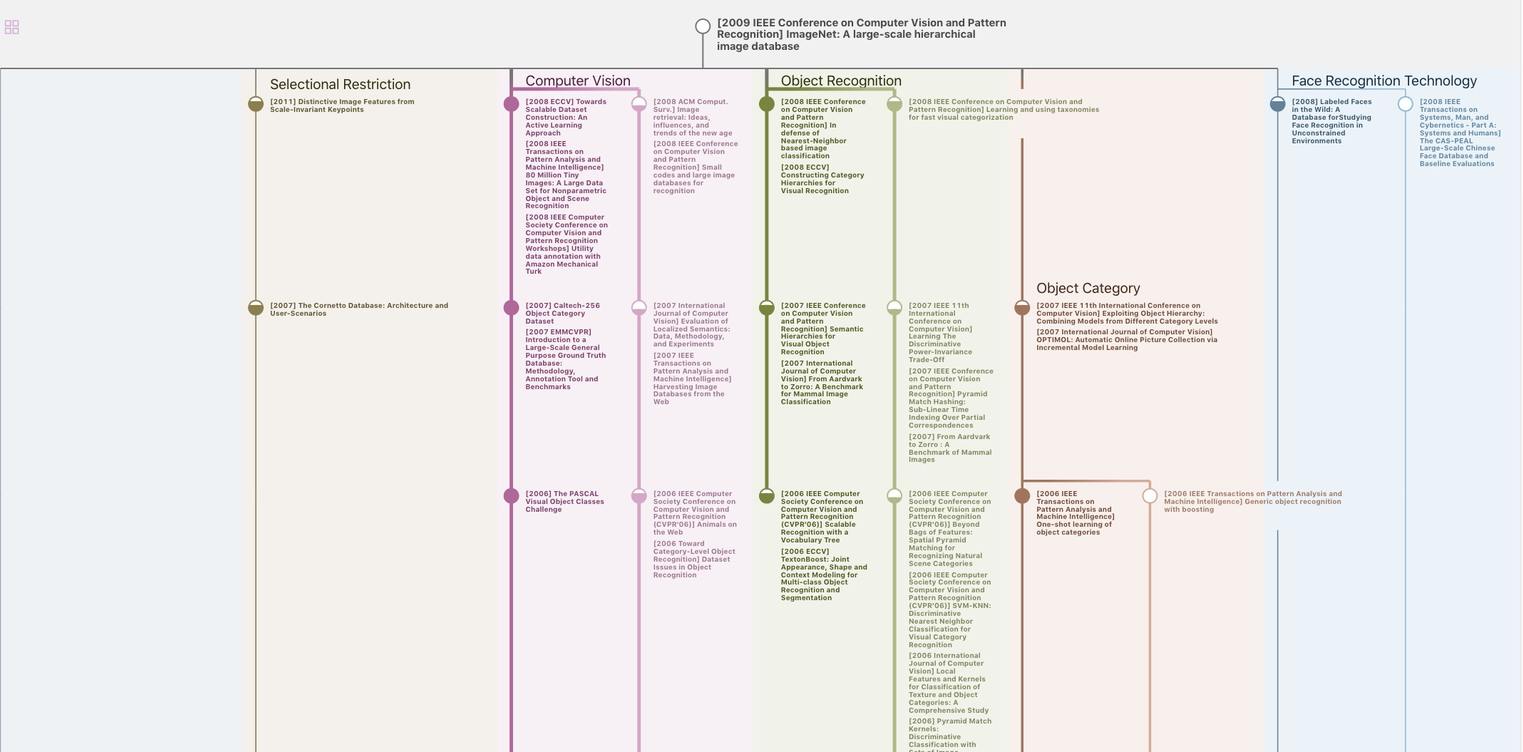
生成溯源树,研究论文发展脉络
Chat Paper
正在生成论文摘要