Key-Performance-Indicator-Related Fault Detection Based on Deep Orthonormal Subspace Analysis
IEEE TRANSACTIONS ON INDUSTRIAL INFORMATICS(2024)
摘要
As a novel multivariable regression method, orthonormal subspace analysis (OSA) divides input data and key performance indicators (KPIs) data into three orthonormal subspaces, resulting in effective detection of KPI-related faults in industrial processes. However, the detection of incipient faults is still a challenging problem. To this end, a deep OSA model is established to extract incipient fault information and detect it in this article. First, the correlation matrix (CM), based on the KPI-related information and input-related information that are completely orthogonal to irrelevant information, is calculated to accurately describe the correlation between input and KPIs. Second, the deep singular value decomposition is performed on CM to divide KPI-related information into multiple subspaces, deeply separate KPI-related information and, not least, mine incipient features of data. Furthermore, the detection statistics of multiple subspaces, fault detectability, and complexity are presented. Finally, a numerical example and an actual thermal power plant are employed to confirm the validity of the proposed method. The results demonstrate that the proposed deep OSA achieves much better detectability for incipient KPI-related fault in theoretical and engineering application than some other existing methods.
更多查看译文
关键词
Correlation matrix (CM),deep orthonormal subspace analysis (OSA),fault detection,key performance indicator (KPI)
AI 理解论文
溯源树
样例
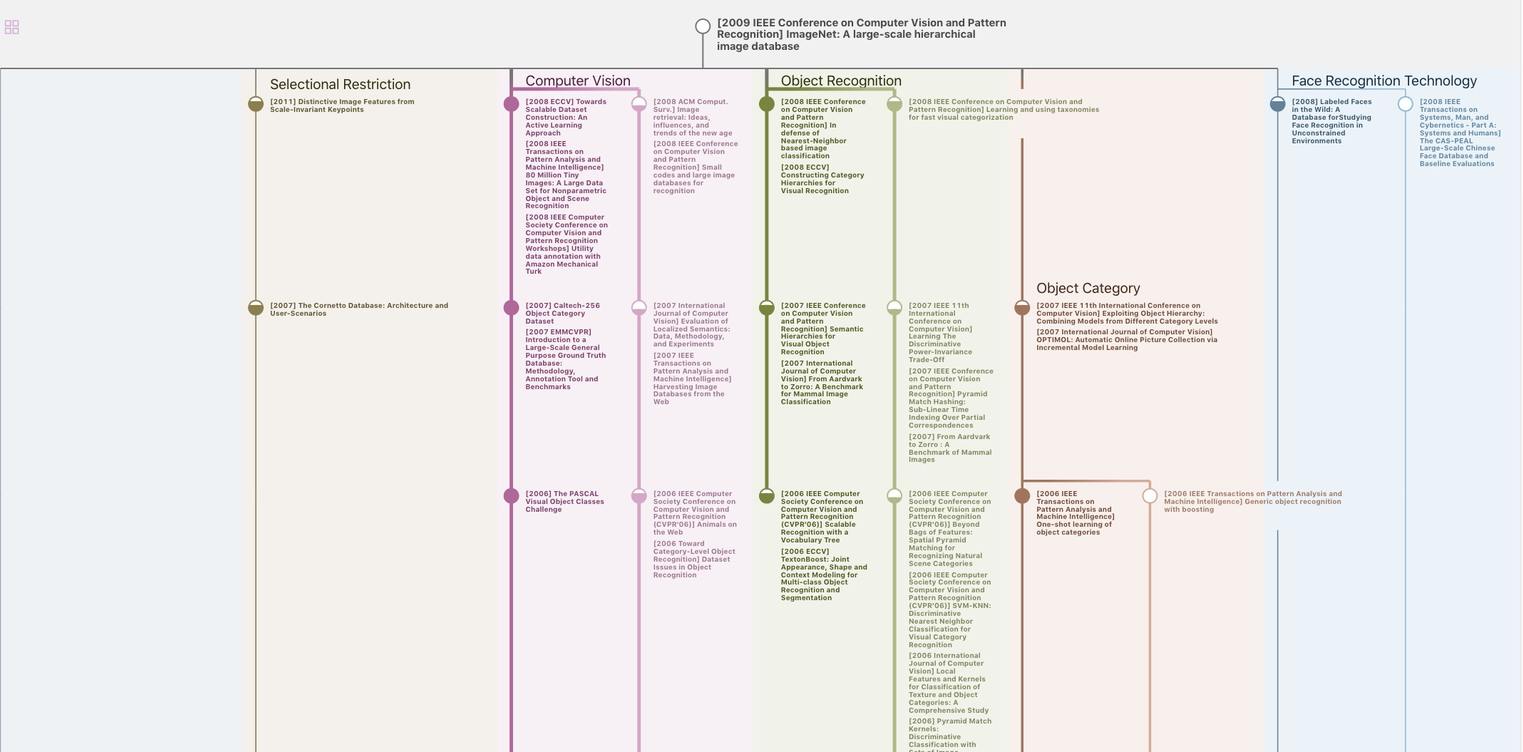
生成溯源树,研究论文发展脉络
Chat Paper
正在生成论文摘要