Auxiliary Model and Key-Term Separation-Based Identification of Hammerstein OE System With Missing Outputs and Outliers
IEEE TRANSACTIONS ON INSTRUMENTATION AND MEASUREMENT(2024)
摘要
This article investigates the problem of robust parameter estimation for the Hammerstein output-error (OE) system with outlier-contaminated and randomly missing outputs. The outliers and data missing are relatively common problems in industrial processes, which can seriously degrade the quality of the collected dataset. In this article, to ensure the robustness against outliers, the measurement noise of the system is mathematically modeled by using a heavy-tailed student's t-distribution; to address the missing outputs and the hidden intermediate term in the Hammerstein OE system, the auxiliary model technique and the key-term separation principle are adopted to parameterize the nonlinear system output reasonably. The parameter estimation problem based on the nonideal dataset is solved by using the generalized expectation maximization (GEM) algorithm, and a robust identification algorithm for the Hammerstein OE system is realized. The robust approach integrates the problems of outliers and missing measurements and gives the mathematical expressions for the unknown model parameters and noise parameters through a rigorous mathematical derivation. Finally, the effectiveness of the proposed approach is verified by a numerical example and a chemical reaction process.
更多查看译文
关键词
Generalized expectation maximization (GEM) algorithm,Hammerstein output-error (OE) system,key-term separation principle,outliers and data missing,system identification
AI 理解论文
溯源树
样例
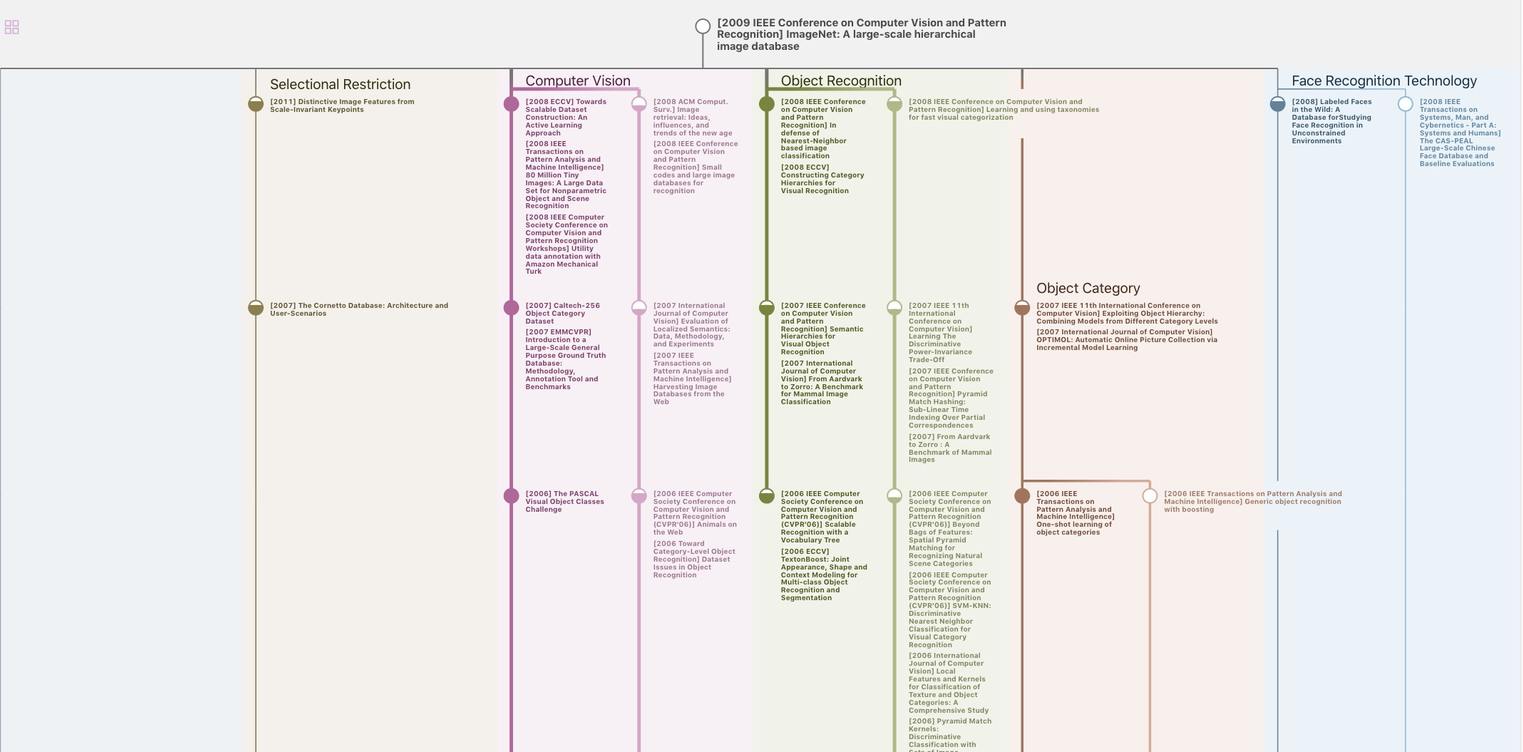
生成溯源树,研究论文发展脉络
Chat Paper
正在生成论文摘要