Exploring Rhythms and Channels-Based EEG Biomarkers for Early Detection of Alzheimer's Disease
IEEE TRANSACTIONS ON EMERGING TOPICS IN COMPUTATIONAL INTELLIGENCE(2024)
摘要
There is no treatment that permanently cures Alzheimer's disease (AD); however, early detection can alleviate the severe effects of the disease. To support early detection of the different stages of AD (e.g., mild, moderate), the key aim of this study is to develop a computer aided diagnostic (CAD) framework that include a long short-term memory (LSTM) network using massive multi-channel electroencephalogram (EEG) data. Although EEG rhythms and EEG channels jointly possess important biomarkers that may be used for diagnosis of AD, but the traditional methods did not explore this issue in any research. To address this problem, this study introduces a new framework to identify the optimal EEG rhythms and channels required for the diagnosis of AD. The proposed framework was tested on a real-time AD EEG dataset. The results reveal that together, the gamma and beta rhythms in the channels, Cz, F4, P4, T6, Pz were the most reliable biomarker for identifying AD and the proposed LSTM based model yielded the best performance. Additionally, another mild cognitive impairment (MCI) EEG dataset was used to test the proposed approach, and the results were excellent (accuracy>99%). The proposed framework will be useful for creating a CAD system to perform automatic AD diagnosis.
更多查看译文
关键词
Alzheimer's disease (AD),electroencephalography (EEG),biomarkers of EEG,long short-term memory (LSTM),mild cognitive impairment (MCI),feature extraction,classification
AI 理解论文
溯源树
样例
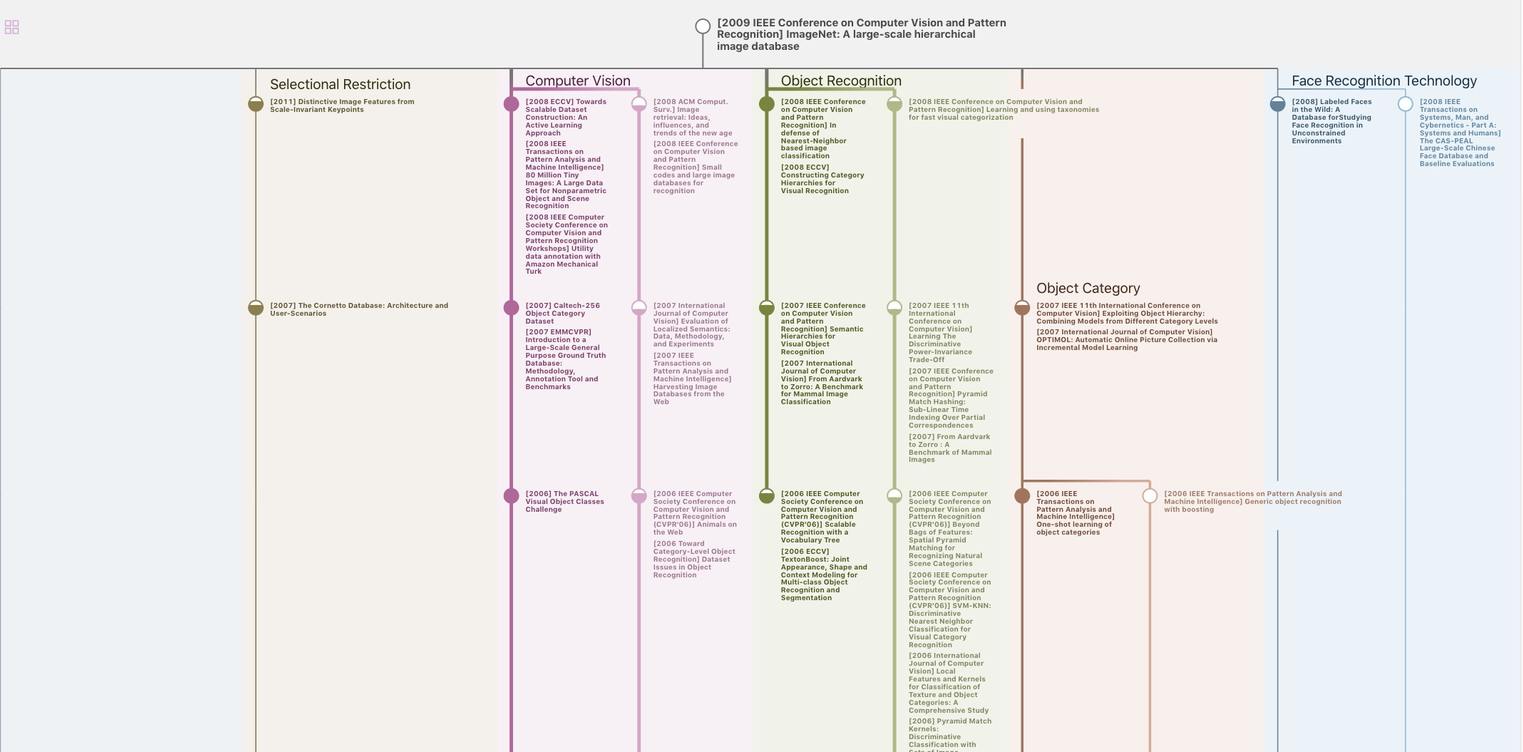
生成溯源树,研究论文发展脉络
Chat Paper
正在生成论文摘要