TV-Net: A Structure-Level Feature Fusion Network Based on Tensor Voting for Road Crack Segmentation
IEEE TRANSACTIONS ON INTELLIGENT TRANSPORTATION SYSTEMS(2024)
摘要
Pavement cracks are a common and significant problem for intelligent pavement maintainment. However, the features extracted in pavement images are often texture-less, and noise interference can be high. Segmentation using traditional convolutional neural network training can lose feature information when the network depth goes larger, which makes accurate prediction a challenging topic. To address these issues, we propose a new approach that features an enhanced tensor voting module and a customized pixel-level pavement crack segmentation network structure, called TV-Net. We optimize the tensor voting framework and find the relationship between tensor scale factors and crack distributions. A tensor voting fusion module is introduced to enhance feature maps by incorporating significant domain maps generated by tensor voting. Additionally, we propose a structural consistency loss function to improve segmentation accuracy and ensure consistency with the structural characteristics of the cracks obtained through tensor voting. The sufficient experimental analysis demonstrates that our method outperforms existing mainstream pixel-level segmentation networks on the same road crack dataset. Our proposed TV-Net has an excellent performance in avoiding noise interference and strengthening the structure of the fracture site of pavement cracks. Code is available at https://github.com/sues-vision/ TV-Net.git.
更多查看译文
关键词
Crack detection,convolutional neural network,tensor voting,U-Net
AI 理解论文
溯源树
样例
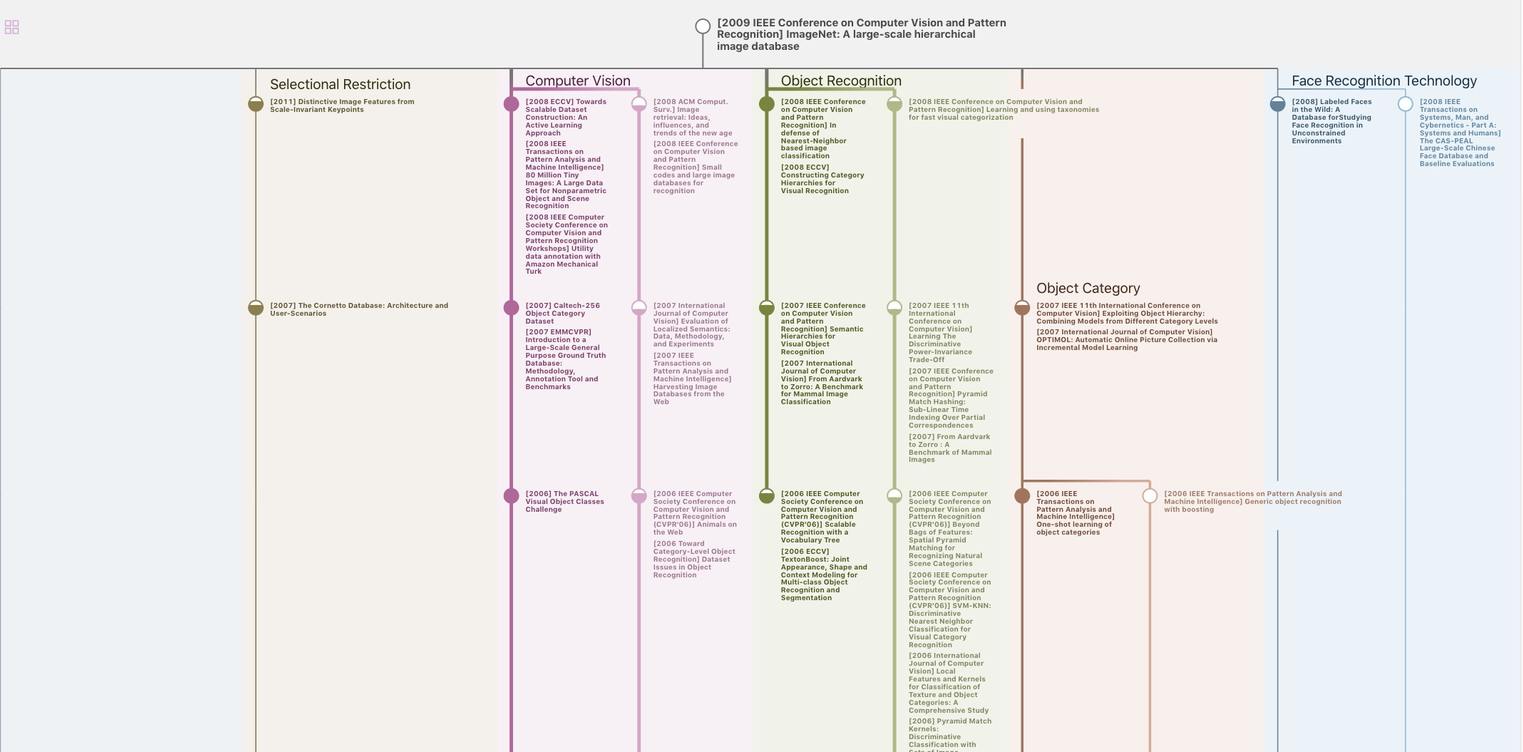
生成溯源树,研究论文发展脉络
Chat Paper
正在生成论文摘要