A Domain Generalization Network Exploiting Causal Representations and Non-Causal Representations for Three-Phase Converter Fault Diagnosis
IEEE TRANSACTIONS ON INSTRUMENTATION AND MEASUREMENT(2024)
摘要
Deep learning (DL) methods have attracted increasing attention and have shown competitive performance in power converter fault diagnosis. However, models trained by existing fault data cannot function well for converter systems with unknown parameters in unknown environments. In addition, obtaining the target data distribution in advance limits the application of transfer learning (TL) methods based on domain adaptation (DA) in real-time fault diagnosis. To address this challenge, this article proposes a domain generalization network exploiting causal representations and non-causal representations (DGNECN) for power converter fault diagnosis under unknown working conditions. First, structural causal models (SCMs) are introduced in converter fault diagnosis, and invariant causal representations with fault information are extracted by the causal factorization module based on a causal matching strategy. Subsequently, the domain-specific non-causal representations are captured by the domain classifier. Finally, the two representations are further purified by disentanglement within a unified framework while optimizing non-causal representations through meta-learning to achieve stable generalization to unknown working conditions. Extensive comparison and ablation experiments confirm the effectiveness and superiority of DGNECN in the domain generalization (DG) setting. DGNECN achieved an average accuracy of 97.38% even in unknown noise and frequency.
更多查看译文
关键词
Feature extraction,Data mining,Mathematical models,Data models,Optimization,Neural networks,Insulated gate bipolar transistors,Causal representations,domain generalization (DG),fault diagnosis,non-causal representations,three-phase inverter
AI 理解论文
溯源树
样例
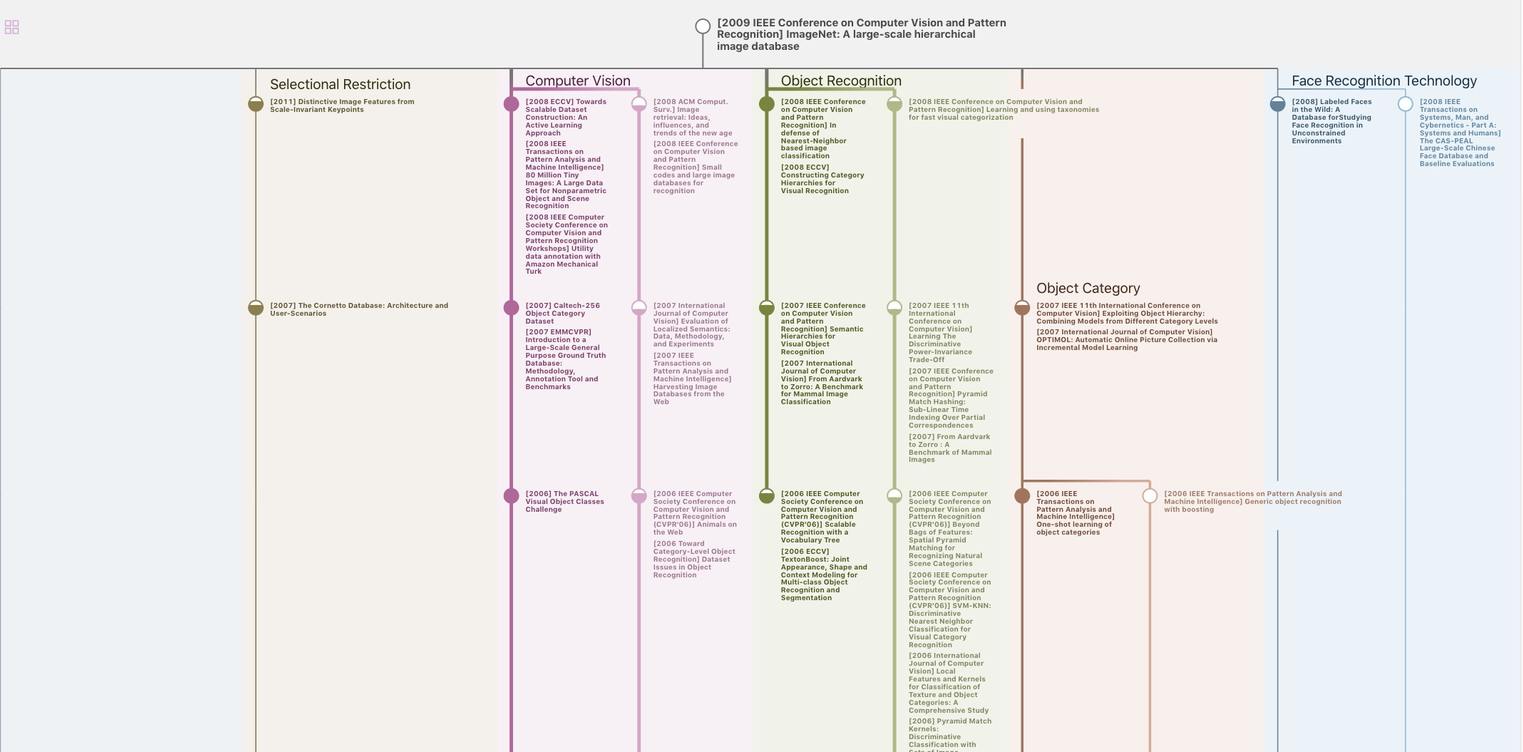
生成溯源树,研究论文发展脉络
Chat Paper
正在生成论文摘要