Consistent Pretext and Auxiliary Tasks With Relative Remaining Useful Life Estimation
IEEE TRANSACTIONS ON INDUSTRIAL INFORMATICS(2024)
摘要
Remaining useful life (RUL) estimation is a crucial enabler of predictive maintenance. Since an adequate amount of labeled data is unavailable due to a low frequency of failures, semisupervised RUL estimation methods have been proposed to utilize unlabeled data collected in maintenance intervals to improve performance. However, those methods have limited capability since they only partially utilize unlabeled data during training. This article proposes a novel semisupervised method for RUL estimation in which both pretext and auxiliary tasks aim to estimate relative RULs. It draws more unlabeled samples from shorter RULs with a geometric progression and applies different strategies for pairing unlabeled samples at different training stages. The proposed method is evaluated on NASA C-MAPSS dataset and Backblaze HDD dataset. The results show that the proposed method significantly improves the performance with scarce labeled data for training.
更多查看译文
关键词
Task analysis,Feature extraction,Estimation,Training,Data mining,Loss measurement,Data models,Deep learning,remaining useful life estimation,semisupervised learning
AI 理解论文
溯源树
样例
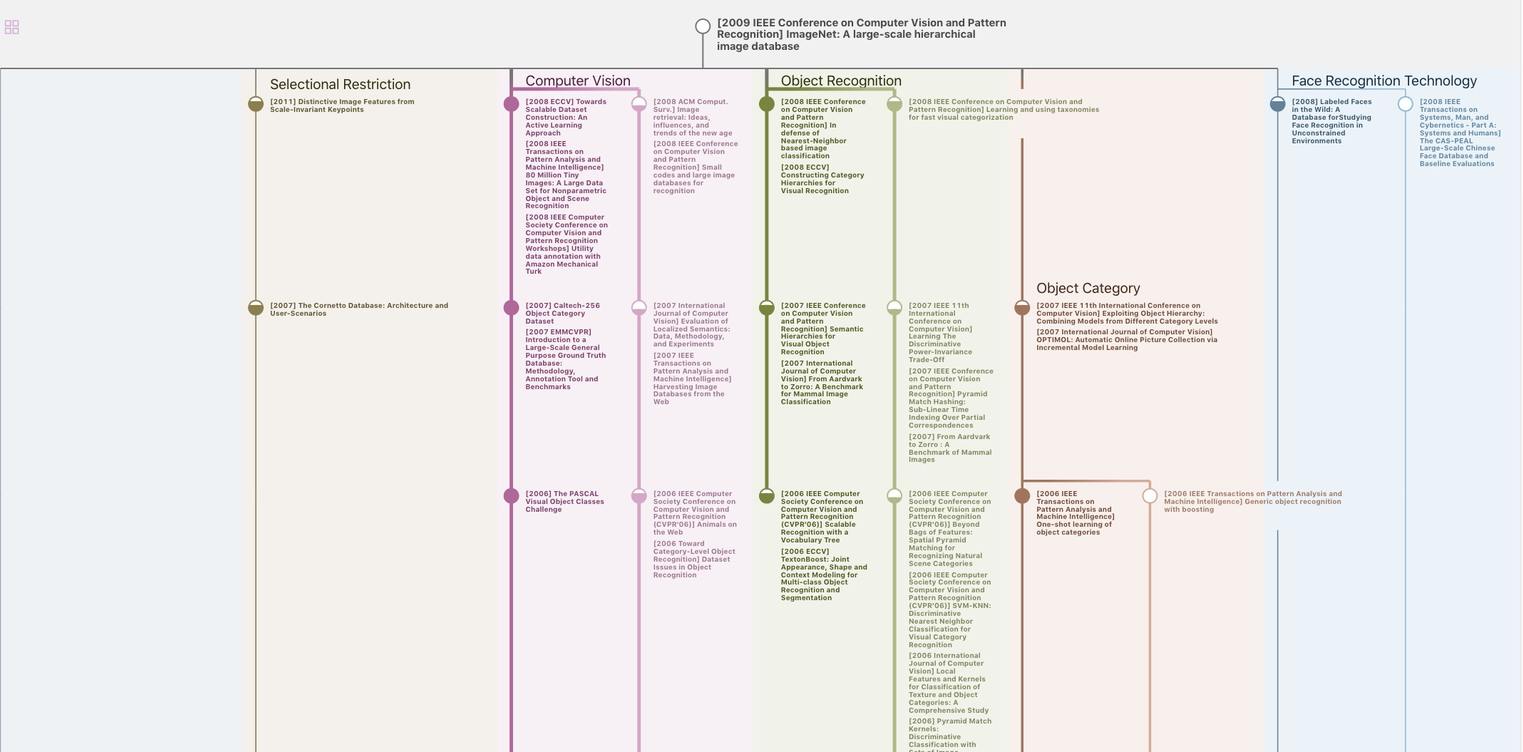
生成溯源树,研究论文发展脉络
Chat Paper
正在生成论文摘要