Fast and Resource Efficient Atrial Fibrillation Detection Framework for Long Term Health Monitoring Devices
IEEE SENSORS LETTERS(2024)
摘要
This letter presents a novel single feature-based atrial fibrillation (AF) detection framework for addressing the critical challenge of resource constraints of affordable wearable health monitoring devices equipped with sensors. The proposed method consists of simple R peak detection for extracting R-R interval, calculation of Shannon entropy of word sequence of symbolic dynamics of heart rate sequence followed by AF/non-AF classification using six classifiers. On four standard databases, with 10 and 30 s electrocardiogram (ECG) segments, the sensitivity (SE) and specificity (SP) of the support vector machine is 100% and 99.92%-100%, respectively. For decision tree, random forest, multilayer perception, naive Bayes, and light gradient boosting algorithms, the SE is 100%, and SP ranges between 99.96% and 100% for 10 and 30 s ECG segments. For further analysis, the datasets with best performance are also tested with approximate entropy and k-nearest neighbor. The best model is decision tree with the lowest model size of 1.30-1.33 kB and processing time (PT) of 2.16 and 0.97 mu s for 10 and 30 s segments, respectively. The realtime implementation on the Raspberry Pi computing platform demonstrates that all methods have small model size with memory space of 1.30-194 KB and PT of 4.82-56.7 mu s, outperforming computationally expensive deep learning-based AF detection methods. The significance and importance of the framework lie in its ability to provide accurate AF detection with low PT and memory space using a single feature, making it suitable for resource-constrained long-term health monitoring devices.
更多查看译文
关键词
Feature extraction,Electrocardiography,Rail to rail inputs,Monitoring,Databases,Sensors,Computational modeling,Sensor signal processing,atrial fibrillation (AF),sensor-equipped wearables,Shannon entropy (SH),symbolic dynamics
AI 理解论文
溯源树
样例
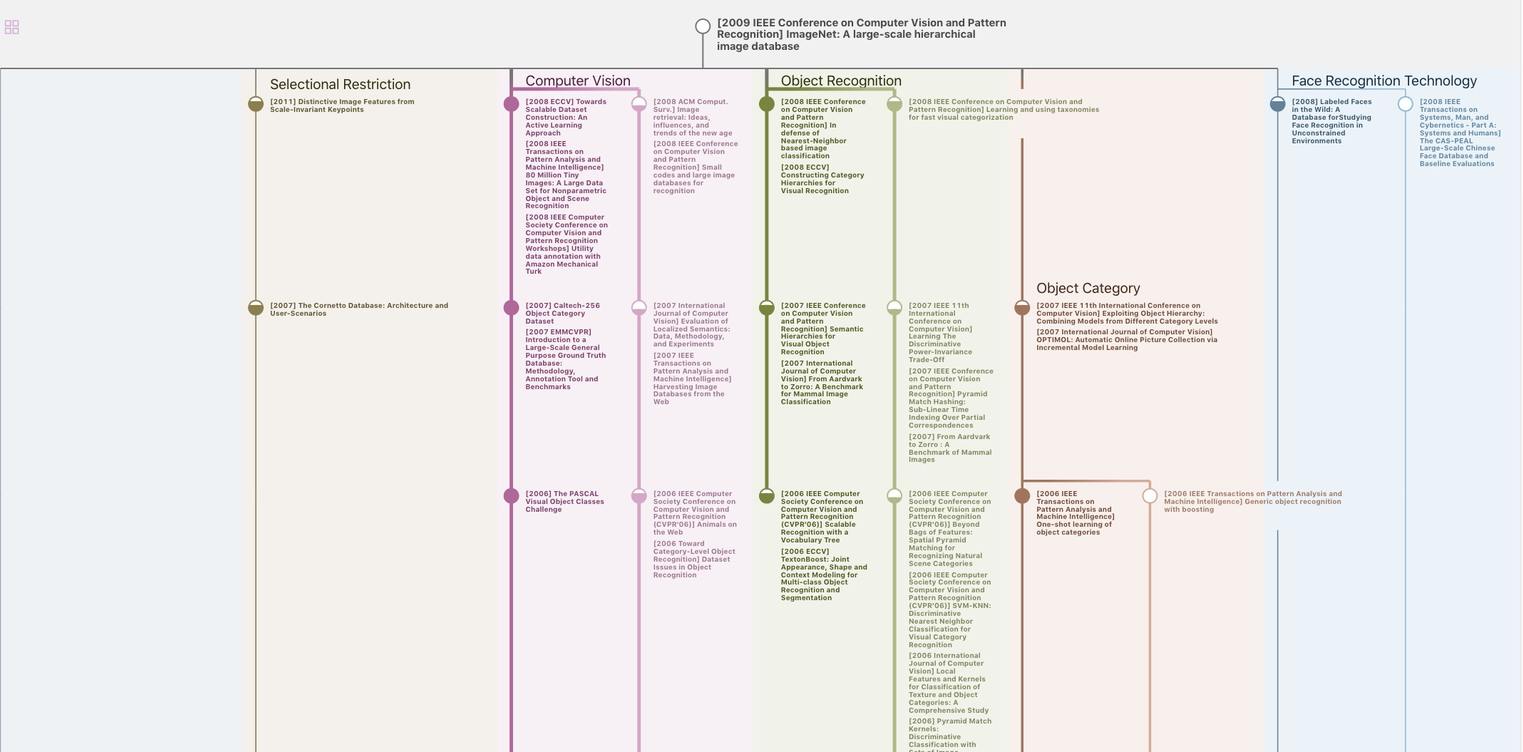
生成溯源树,研究论文发展脉络
Chat Paper
正在生成论文摘要