From Dense to Sparse: Low-Latency and Speed-Robust Event-Based Object Detection
IEEE Transactions on Intelligent Vehicles(2024)
摘要
Recently, bio-inspired event cameras have seen increased use for object detection in autonomous driving due to their advantages of high temporal resolution and high dynamic range. However, how to leverage the high-speed and asynchronous characteristics of event streams to achieve accurate and robust detection within low end-to-end latency remains a key unresolved issue. Prior methods not only struggle with high latency but also encounter difficulties in robustly detecting objects at varying speeds. In this paper, we propose a novel dense-to-sparse event-based object detection framework called DTSDNet. The event temporal image is first proposed to preserve motion and temporal information in the event stream. Then, rich spatial features from the dense pathway are integrated into the sparse pathway through the attention-based dual-pathway aggregation module. To assess the speed robustness of the model and event representation, we propose a simple yet effective relative speed estimation method. The experimental results demonstrate that the model and event representation can achieve state-of-the-art (SOTA) detection performance and superior speed robustness on the event-based object detection datasets. Moreover, this dense-to-sparse framework can reduce the accumulation time of the event stream by a factor of 5 (from 50 ms to 10 ms) while maintaining SOTA detection performance, meeting the low-latency requirements of perception in real-time driving.
更多查看译文
关键词
Event cameras,Object detection,Event-based vision,Deep learning,Low latency,Speed robust
AI 理解论文
溯源树
样例
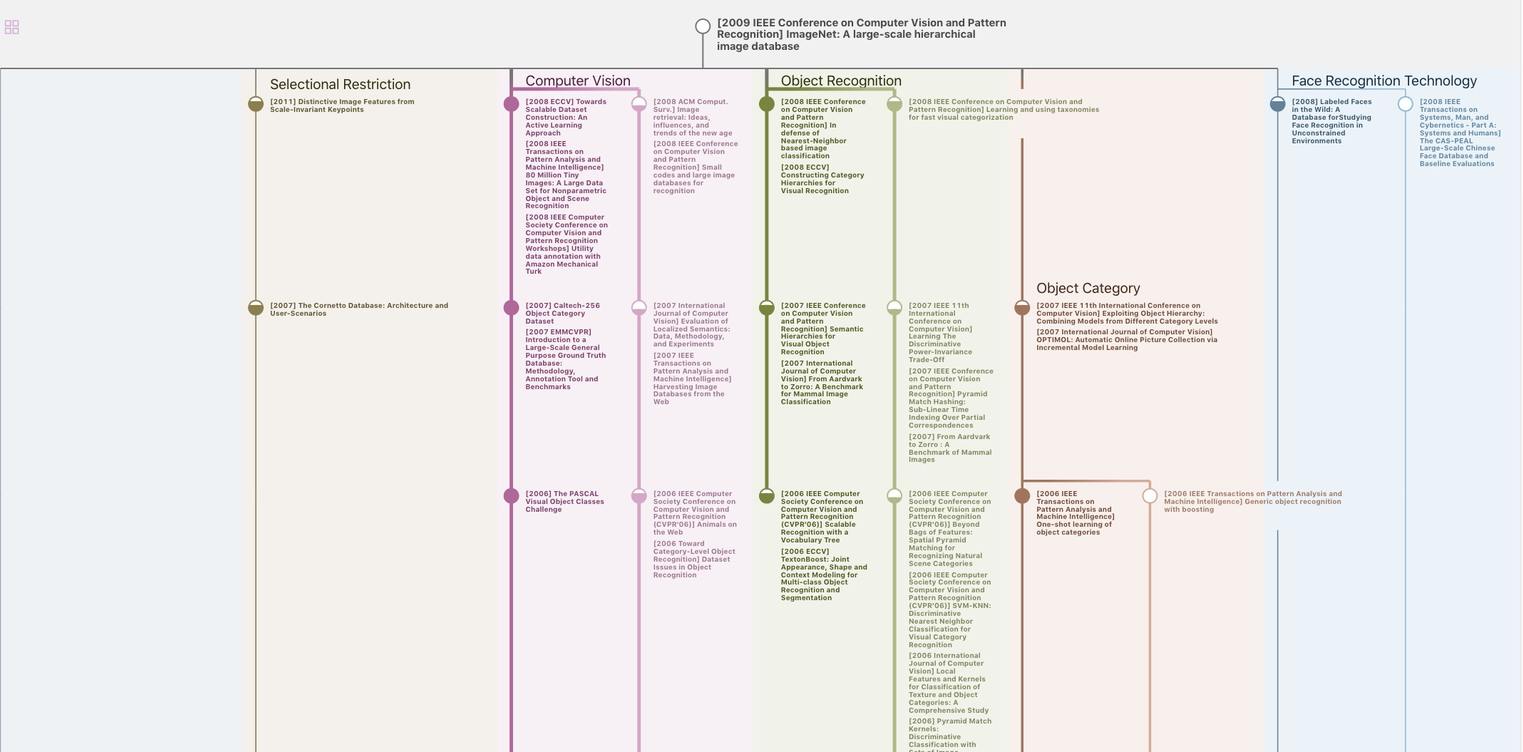
生成溯源树,研究论文发展脉络
Chat Paper
正在生成论文摘要