Fusing Fuzzy Entropy With Gaussian and Exponential Membership Functions Outperforms Traditional Entropy Metrics in Monitoring the Depth of Anesthesia Using a Single Frontal EEG Channel
IEEE SENSORS LETTERS(2024)
摘要
While entropy metrics derived from electroencephalography (EEG) have shown significant promise in monitoring the depth of anesthesia (DoA), the applicability of fuzzy entropy (FuzzEn) initially proposed to address the limitations of conventional entropy metrics, regarding the sample size and class boundaries, remains unexplored in this context. This letter addresses two primary objectives: proposing a new method for DoA monitoring using a fusion of FuzzEn with Gaussian and exponential membership functions, specifically designed for a wearable single frontal EEG channel system, and evaluating its comparative effectiveness against other entropy metrics. First, the EEG signal undergoes denoising and is then decomposed into subbands. Second, seven entropy metrics, including FuzzEn, are extracted from each subband. Finally, each set of individual entropy metrics obtained from all subbands is separately fed into a regressor. In direct comparison with other metrics, such as sample entropy and approximate entropy, the proposed fused FuzzEn exhibited clear superiority with a higher mean correlation coefficient (0.85 versus 0.63, 0.61) and lower mean absolute error (5.4 versus 8.7, 8.9) between the reference and estimated DoA index values. The obtained results underscore the potential of the proposed FuzzEn for DoA monitoring.
更多查看译文
关键词
Sensor applications,anesthesia,depth of anesthesia (DoA),electroencephalography (EEG),fuzzy entropy (FuzzEn),wearable
AI 理解论文
溯源树
样例
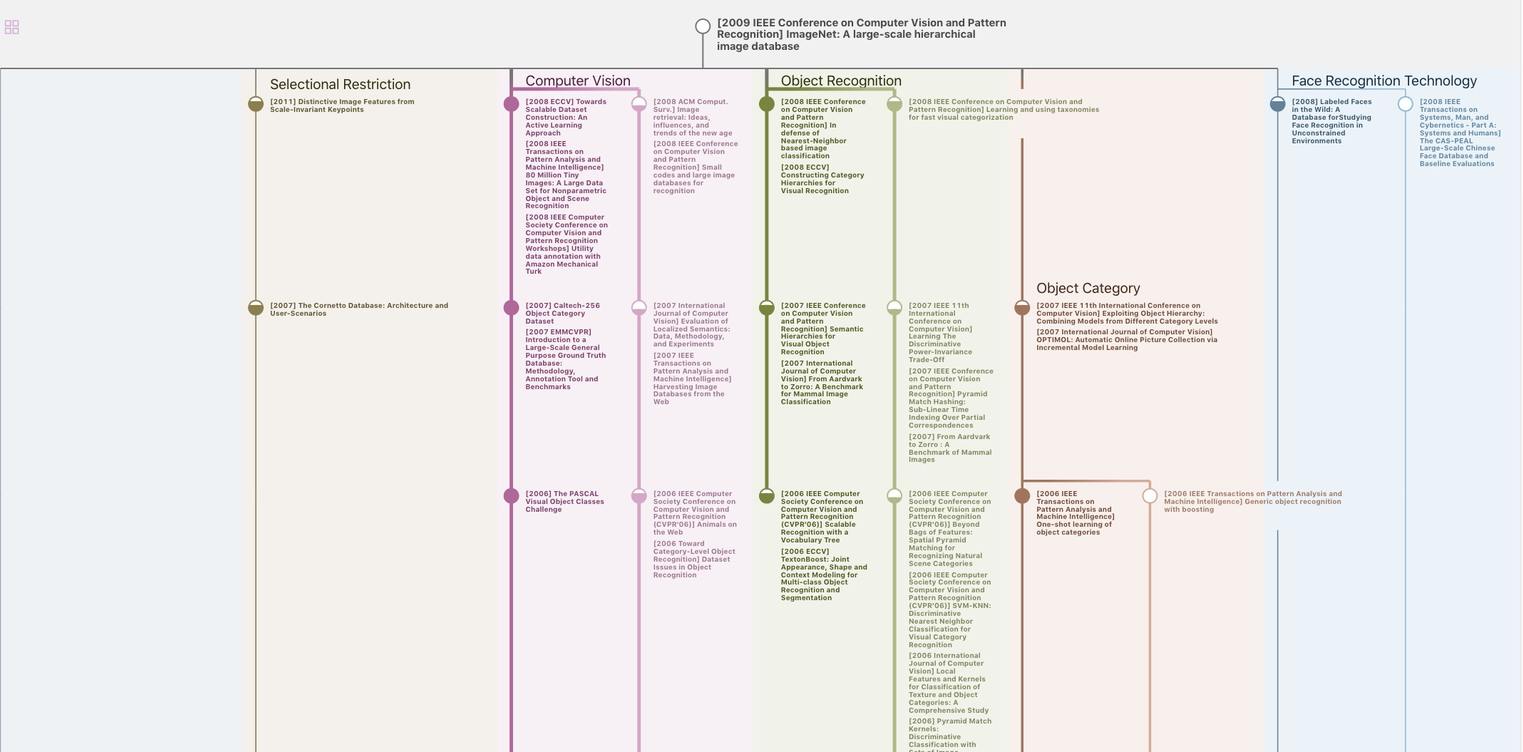
生成溯源树,研究论文发展脉络
Chat Paper
正在生成论文摘要