Cooperative Probabilistic Trajectory Prediction under Occlusion
IEEE Transactions on Intelligent Vehicles(2024)
摘要
A fundamental challenge for autonomous systems is to reliably detect and estimate occluded objects. Particularly in complex environments involving multiple agents, navigating in close proximity of occluded objects can be challenging. Recent advances in communication have tried to address this by sharing the information of occluded object among connected autonomous agents. However, communicating rich sensor information under limited bandwidth may not be feasible. Further, future state prediction of such occluded objects based on limited information can be unreliable. Therefore, this paper proposes a novel idea for estimating the states as well as probabilistically predicting the future trajectory of any object under occlusion. We leverage the idea of cooperative perception where connected autonomous agents sharing a common field of view can obtain the essential information like position and velocity of occluded object. Our novel end-to-end probabilistic model then utilizes this information to predict the future states of the occluded object with uncertainty. Unlike deterministic trajectory prediction with point estimates, the current prediction model utilizes dropout during inference to obtain probabilistic outputs. Overall experimental results showed that our model can reliably estimate and transform the states of occluded objects to the ego agent's reference with less than 5% error. Further, the predicted trajectory of occluded object is close to the ground truth trajectory with the predicted trajectory lying within 2
$\sigma$
predictive distribution where ground truth trajectory is obtained assuming no occlusion. Our proposed method holds promise for reliable prediction of surrounding objects under occlusion in a multi-agent scenario.
更多查看译文
关键词
Cooperative Perception,Bayesian Inference,Occlusion,Trajectory Prediction
AI 理解论文
溯源树
样例
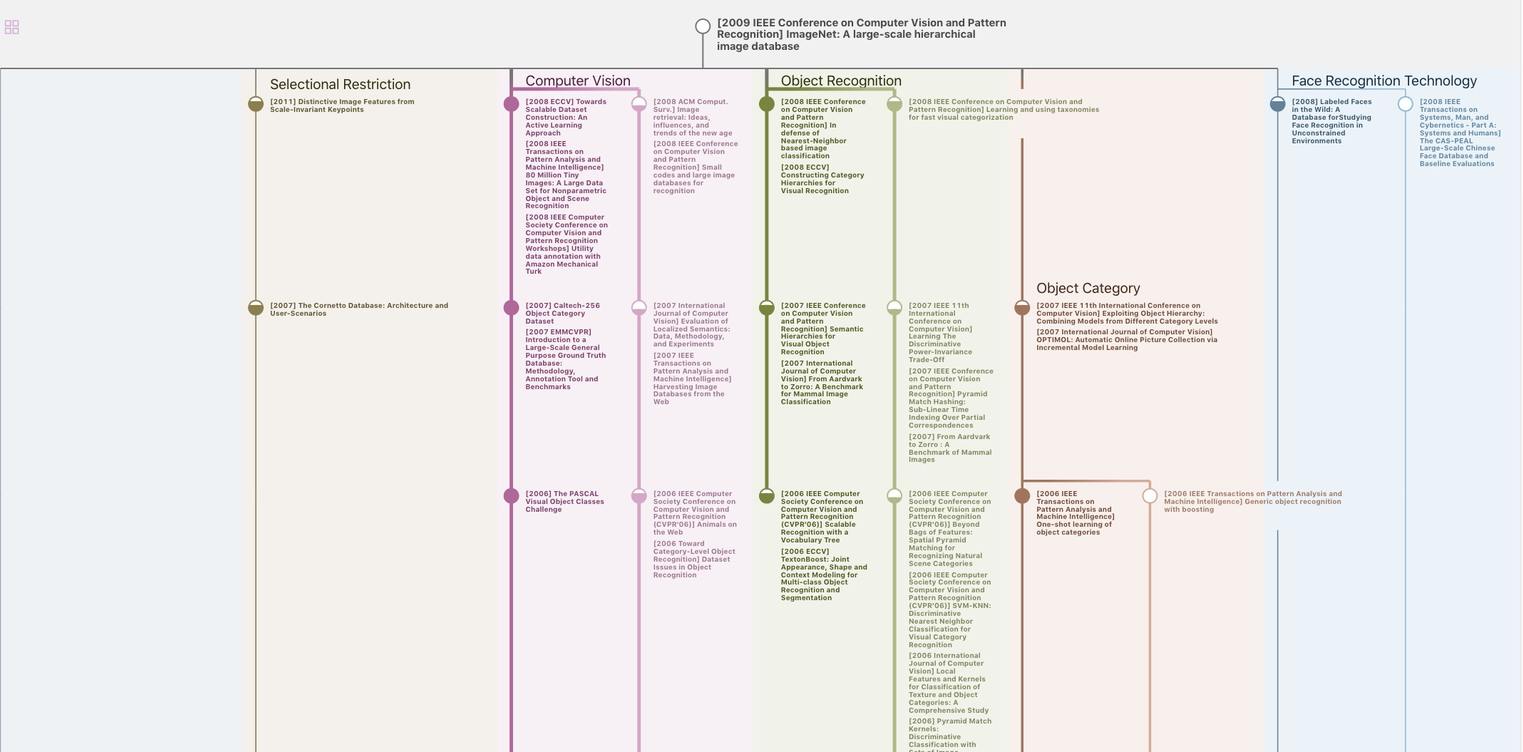
生成溯源树,研究论文发展脉络
Chat Paper
正在生成论文摘要