An End-to-End Deep Reinforcement Learning Based Modular Task Allocation Framework for Autonomous Mobile Systems
IEEE TRANSACTIONS ON AUTOMATION SCIENCE AND ENGINEERING(2024)
摘要
Intelligent decision-making systems that can solve task allocation problems are critical for multi-robot systems to conduct industrial applications in a collaborative and automated way, such as warehouse inspection using mobile robots, hydrographic surveying using unmanned surface vehicles, etc. This paper, therefore, aims to address the task allocation problem for multi-agent autonomous mobile systems to autonomously and intelligently allocate multiple tasks to a fleet of robots. Such a problem is normally regarded as an independent decision-making process decoupled from the following task planning for the member robots. To avoid the sub-optimal allocation caused by the decoupling, an end-to-end task allocation framework is proposed to tackle this combinatorial optimisation problem while taking the succeeding task planning into account during the optimisation process. The problem is formulated as a special variant of the multi-depot multiple travelling salesmen problem (mTSP). The proposed end-to-end task allocation framework employs deep reinforcement learning methods to replace the handcrafted heuristics used in previous works. The proposed framework features a modular design of the reinforcement learning agent which can be customised for various applications. Moreover, a real-robot implementation setup based on the Robot Operating System 2 is presented to fulfil the simulation-to-reality gap. A warehouse inspection mission is executed to validate the training outcome of the proposed framework. The framework has been cross-validated via both simulated and real-robot tests with various parameter settings, where adaptability and performance are well demonstrated. Note to Practitioners-This paper is motivated by the problem of dispatching a fleet of autonomous mobile robots to tackle a mission that can be resolved into multiple waypoint-following tasks. An end-to-end modular framework is proposed, making task allocation decisions based on the given waypoint information. By using the reinforcement learning technique, the deep neural network could learn sophisticated policies for allocating tasks. The policies are trained in a specific pattern which ensures their joint optimisation for a solver that outputs the near optimal task execution sequences in an efficient way. This leads to a multiple travelling salesmen problem (mTSP) solution. Pre-trained policies are tested in several industrial scenarios reflecting the applications of search and rescue, maritime surveying, and warehouse automation, among others. A hardware implementation configuration based on the Robot Operating System 2 is also presented to support the practical deployment the framework.
更多查看译文
关键词
Deep reinforcement learning,task allocation,multi-agent planning,field robotics
AI 理解论文
溯源树
样例
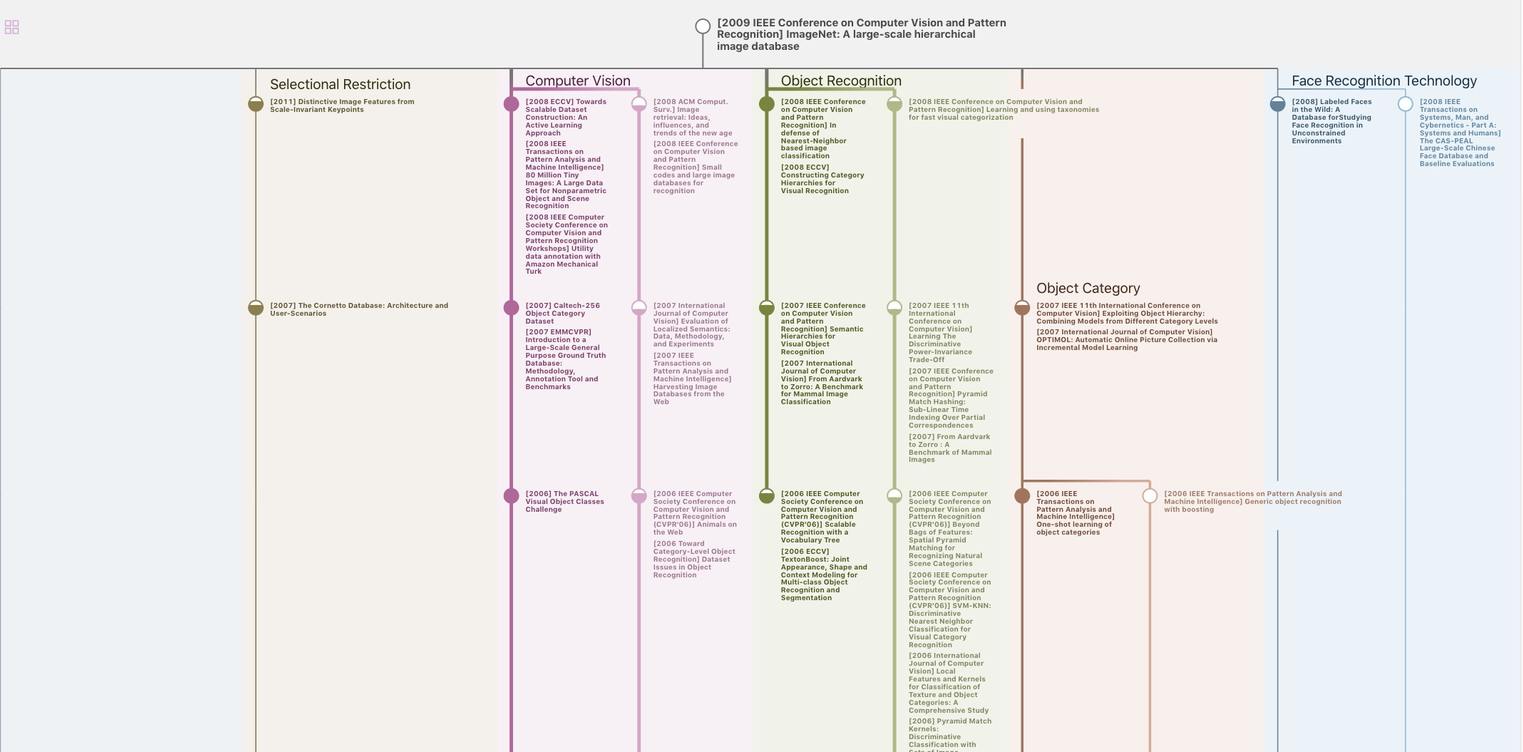
生成溯源树,研究论文发展脉络
Chat Paper
正在生成论文摘要