Coarse-to-Fine Cross-View Interaction Based Accurate Stereo Image Super-Resolution Network.
IEEE Trans. Multim.(2024)
摘要
Recently, parallax attention based stereo image super-resolution (SR) methods, which can better explore cross-view information, have been widely studied. Despite the impressive performance of these methods, almost all of them calculate parallax attention maps at a single low resolution, which will lead to ambiguous stereo correspondence. Besides, the widely used parallax attention module (PAM) cannot handle the illuminance variations in stereo image pairs, and cannot distinguish the contribution of the captured cross-view features to the reconstruction of the target view. To this end, in this paper, we propose a coarse-to-fine cross-view interaction based network (C2FNet) to achieve more accurate cross-view information capturing. Firstly, in C2FNet, a coarse-to-fine cascaded parallax attention structure (C2F-CPAS), which conforms with the human visual mechanism, is constructed to gradually perform parallax attention from the low-resolution to high-resolution level. Thus, richer textures can be used to learn more reliable stereo correspondence. Meanwhile, a multi-level attention transfer loss is designed to further calibrate the accuracy of stereo correspondence at each level. Secondly, we propose a modified PAM (MPAM) to alleviate the limitations of common PAM so that illuminance-robust stereo correspondence can be learned and more important cross-view information can be selected. Extensive experimental results show that our proposed C2FNet outperforms the state-of-the-art methods on various datasets.
更多查看译文
关键词
Stereo image super-resolution,Coarse-to-fine structure,Attention transfer loss,Modified parallax attention
AI 理解论文
溯源树
样例
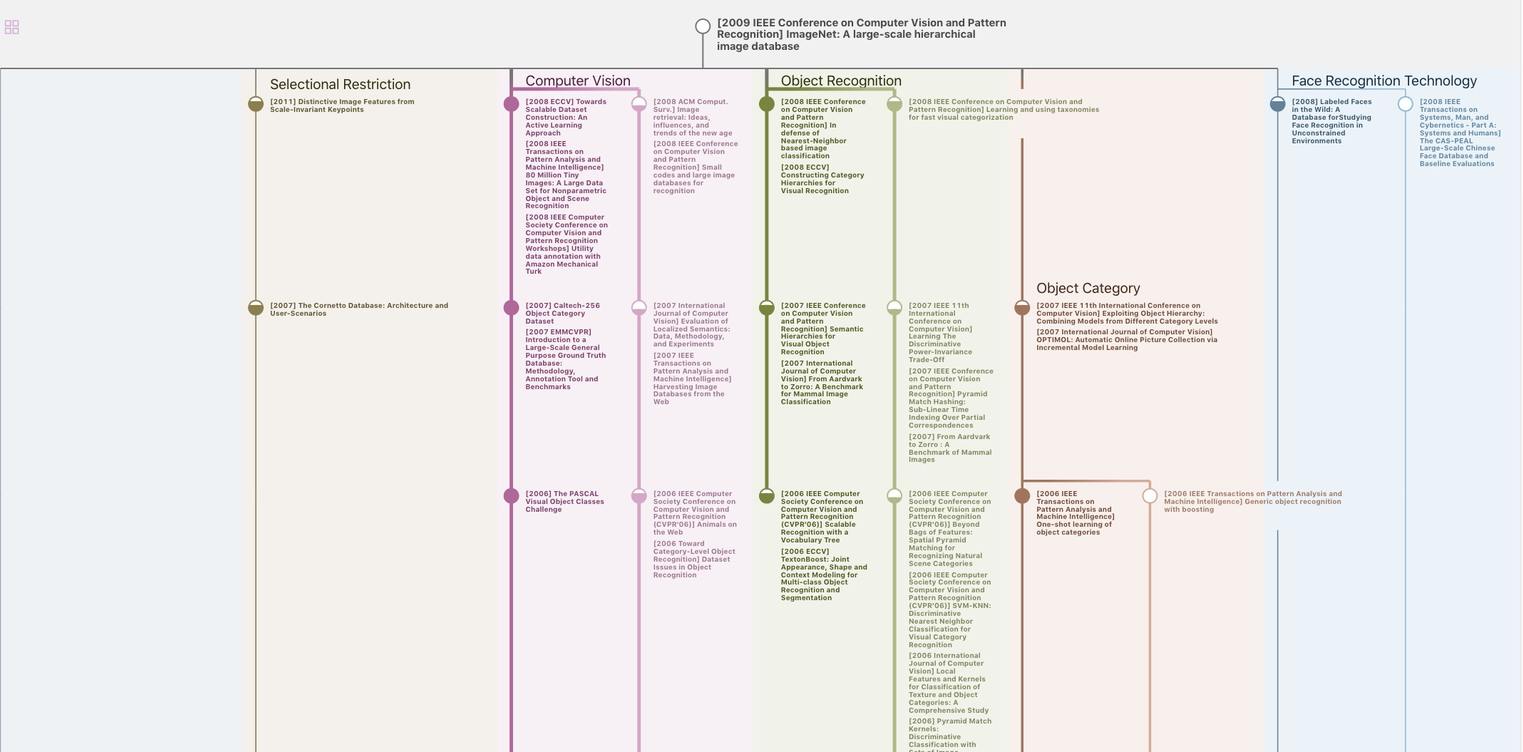
生成溯源树,研究论文发展脉络
Chat Paper
正在生成论文摘要