Uncertainty-Aware Autonomous Robot Exploration Using Confidence-Rich Localization and Mapping
IEEE TRANSACTIONS ON AUTOMATION SCIENCE AND ENGINEERING(2024)
摘要
Information-based autonomous robot exploration methods, aiming to maximize the exploration rewards, e.g., mutual information (MI), get more prevalent in field robotics applications. However, most MI-based exploration methods assume known poses or use inaccurate pose uncertainty approximation, which may lead to deviation or even failure when exploring prior unknown environments. In this paper, we explicitly consider full-state (pose & map) uncertainty for balancing exploration and localizability, i.e., avoiding the robot guiding itself to complex scenes with high exploration rewards but hard to localize. We first propose a Rao-Blackwellized particle filter-based localization and mapping framework (RBPF-CLAM) for a dense environmental map with continuous occupancy distribution. Then we develop a new closed-form particle weighting method to improve the localization accuracy and robustness. We further use these weighted particles to approximate the unknown pose uncertainty and combine it with our previous confidence-rich mutual information (CRMI) metric to evaluate the expected information utility of the robot's new control actions. This new information metric is called uncertain CRMI (UCRMI). Dataset experiments show our RBPF-CLAM improves about 44.7% average root mean square error than the state-of-the-art RBPF localization method, and real-world experimental results show that our UCRMI reduces the pose uncertainty about 32.85% more than CRMI and 25.36% time cost than UGPVR in the exploration of unknown and unstructured scenes given sparse measurements, which shows better performance than other state-of-the-art information metrics.
更多查看译文
关键词
Robots,Uncertainty,Simultaneous localization and mapping,Location awareness,Autonomous robots,Measurement,Trajectory,Autonomous robot exploration,active SLAM,mutual information,range sensors,particle filter
AI 理解论文
溯源树
样例
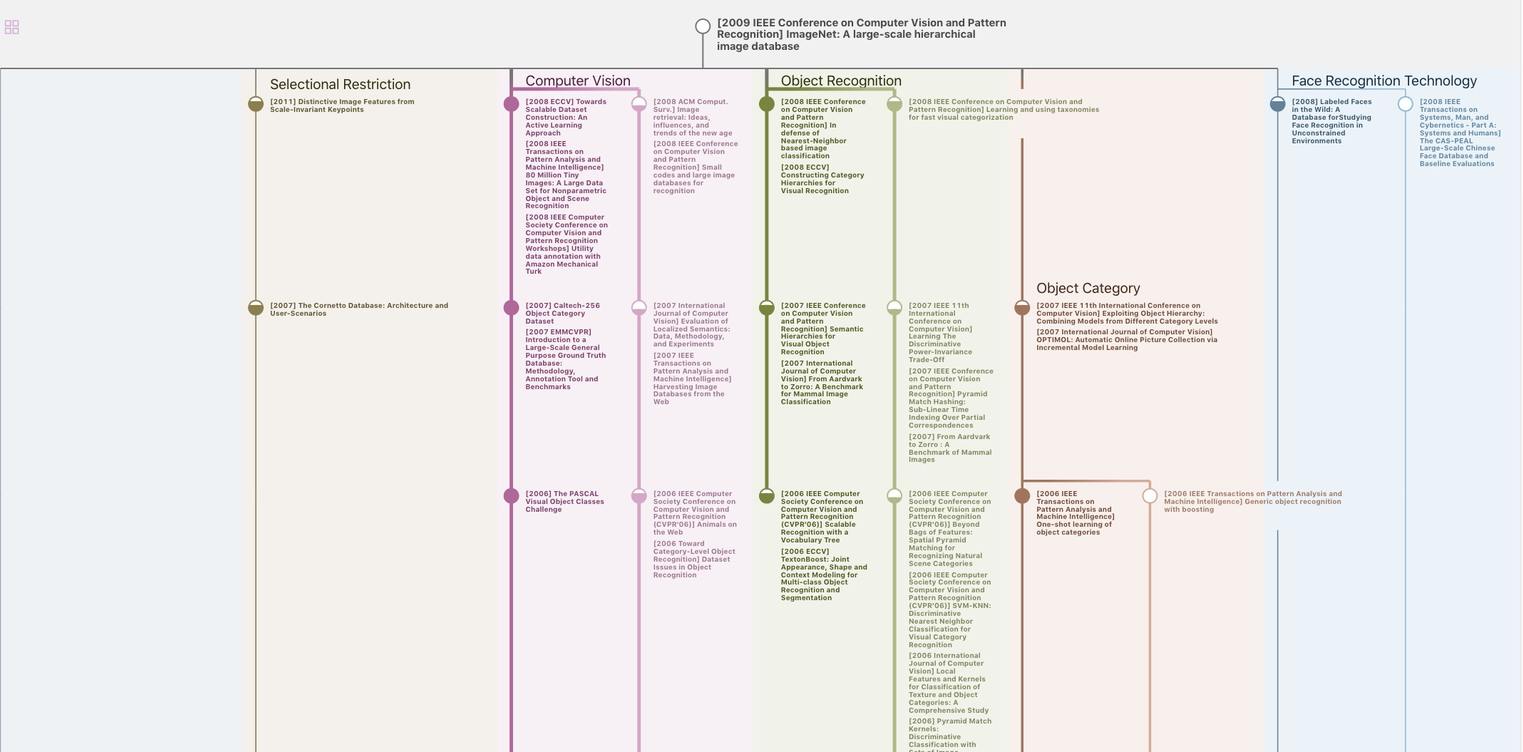
生成溯源树,研究论文发展脉络
Chat Paper
正在生成论文摘要