Fractional-Order Systems Optimal Control via Actor-Critic Reinforcement Learning and Its Validation for Chaotic MFET
IEEE TRANSACTIONS ON AUTOMATION SCIENCE AND ENGINEERING(2024)
摘要
Since the existence of fractional order dynamics, it is difficult to obtain an optimality equation to solve for fractional-order optimal control. In this paper, a fractional Hamilton-Jacobi-Bellman (HJB) equation based on error derivative is proposed, and a corresponding online learning algorithm is designed. The scheme can handle the optimal tracking problem for the 0 < alpha <= 1 order nonlinear systems. Since the traditional quadratic cost function is unbounded at infinite time and the optimal control derived from the discounted cost function fails to stabilize the system asymptotically, a cost function based on the error derivative is proposed, which can avoid these problems, and the system is not restricted to be zero equilibrium. Then, the fractional HJB equation is derived by constructing an auxiliary signal without directly using the chain rule of differentiation. The optimality, stability and convergence of its solution are proved, and actor-critic neural networks (NNs) are established to perform the RL algorithm. Finally, the algorithm is applied to a chaotic magnetic-field electromechanical transducer (MFET) system to verify the effectiveness and advantages.
更多查看译文
关键词
Fractional-order systems,optimal control,reinforcement learning (RL),neural networks,adaptive dynamic programming
AI 理解论文
溯源树
样例
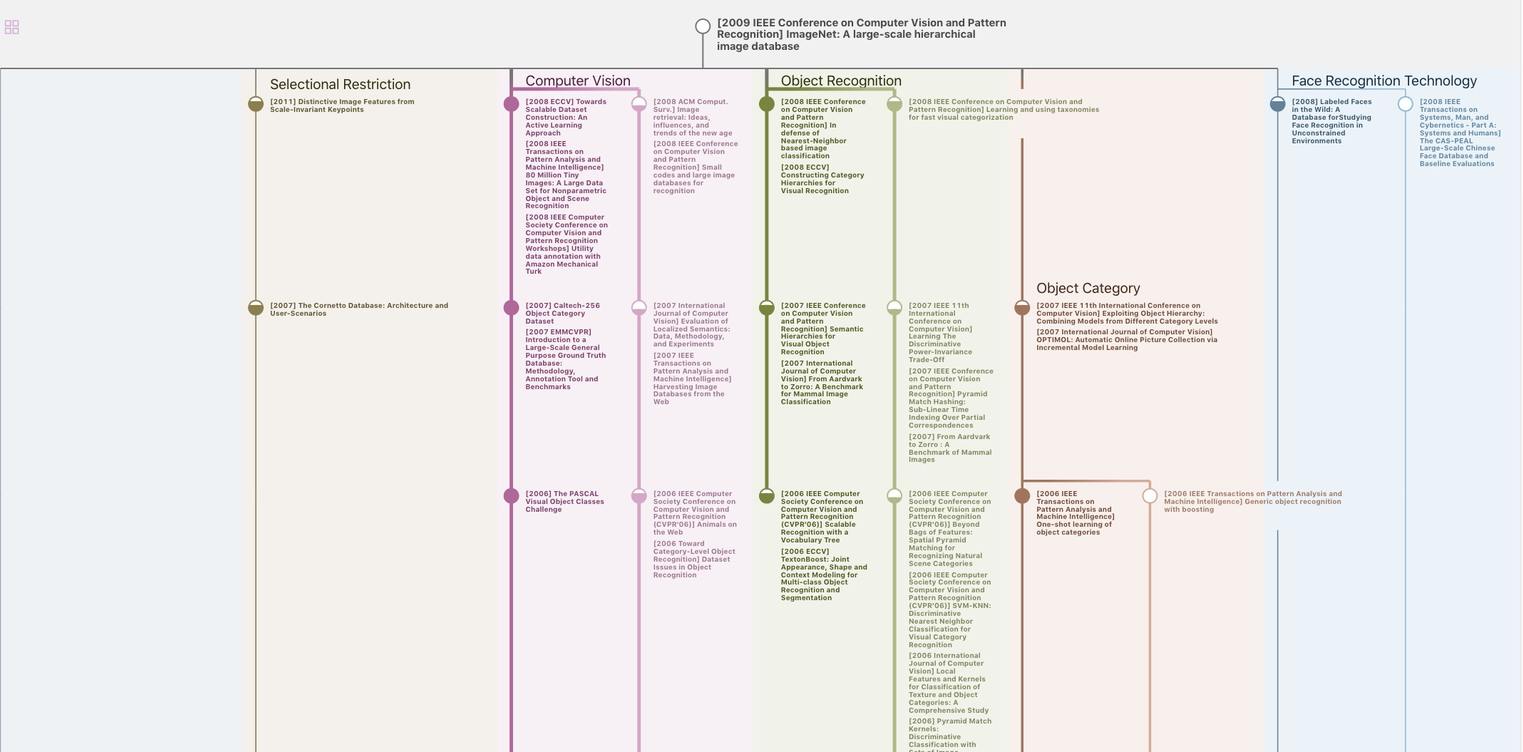
生成溯源树,研究论文发展脉络
Chat Paper
正在生成论文摘要