Rapid Surrogate-Aided Multicriterial Optimization of Compact Microwave Passives Employing Machine Learning and ANNs
IEEE TRANSACTIONS ON MICROWAVE THEORY AND TECHNIQUES(2024)
摘要
This article introduces an innovative method for achieving low-cost and reliable multiobjective optimization (MOO) of microwave passive circuits. The technique capitalizes on the attributes of surrogate models, specifically artificial neural networks (ANNs), and multiresolution electromagnetic (EM) analysis. We integrate the search process into a machine learning (ML) framework, where each iteration produces multiple infill points selected from the present representation of the Pareto set. This collection is formed by optimizing the ANN metamodel by means of a multiobjective evolutionary algorithm (MOEA). The procedure concludes upon convergence, defined as a significant similarity between the sets of nondominated solutions acquired through consecutive iterations. Performing the majority of iterations at the low-fidelity EM simulation level enables additional computational savings. Our methodology has been showcased using two microstrip circuits. Comparative assessments against various surrogate-assisted benchmark methods demonstrate the algorithm's competitive performance in terms of computational efficiency and the quality of the Pareto set generated in the course of the optimization run.
更多查看译文
关键词
Computer-aided design,machine learning (ML),microwave engineering,multicriterial optimization (MOO),neural networks,variable-fidelity simulations
AI 理解论文
溯源树
样例
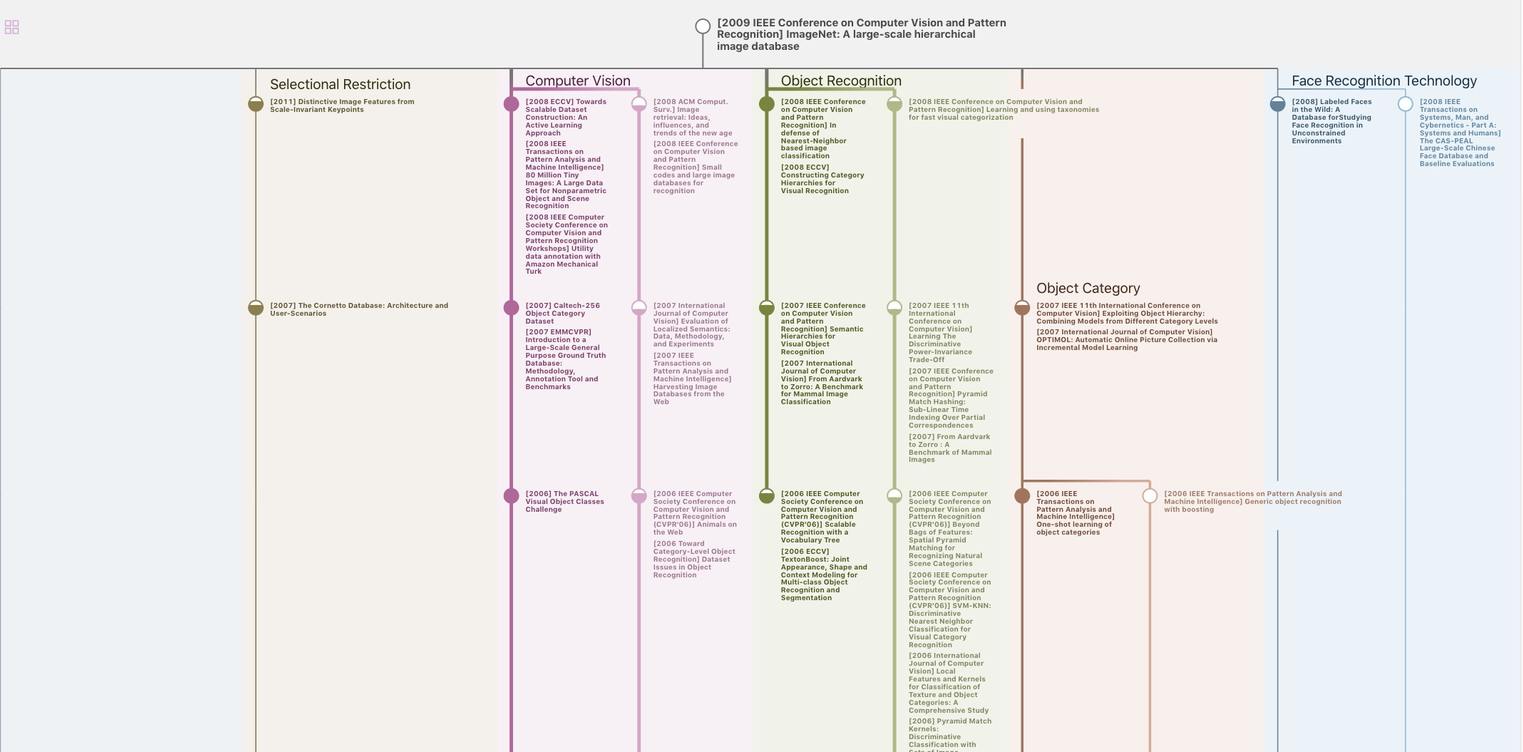
生成溯源树,研究论文发展脉络
Chat Paper
正在生成论文摘要