When is It Likely to Fail? Performance Monitor for Black-Box Trajectory Prediction Model
IEEE transactions on automation science and engineering(2024)
摘要
Accurate trajectory prediction is vital for various applications, including autonomous vehicles. However, the complexity and limited transparency of many prediction algorithms often result in black-box models, making it challenging to understand their limitations and anticipate potential failures. This further raises potential risks for systems based on these prediction models. This study introduces the performance monitor for black-box trajectory prediction model (PMBP) to address this challenge. The PMBP estimates the performance of black-box trajectory prediction models online, enabling informed decision-making. The study explores various methods’ applicability to the PMBP, including anomaly detection, machine learning, deep learning, and ensemble, with specific monitors designed for each method to provide online output representing prediction performance. Comprehensive experiments validate the PMBP’s effectiveness, comparing different monitoring methods. Results show that the PMBP effectively achieves promising monitoring performance, particularly excelling in deep learning-based monitoring. It achieves improvement scores of 0.81 and 0.79 for average prediction error and final prediction error monitoring, respectively, outperforming previous white-box and gray-box methods. Furthermore, the PMBP’s applicability is validated on different datasets and prediction models, while ablation studies confirm the effectiveness of the proposed mechanism. Hybrid prediction and autonomous driving planning experiments further show the PMBP’s value from an application perspective. Project page: https://swb19.github.io/PMBP/. Note to Practitioners —This research presents PMBP, a valuable tool for practitioners in the automation industry. The PMBP enables online monitoring of black-box trajectory prediction models, enhancing system reliability and facilitating informed decision-making. The practical application of PMBP lies in improving safety and reliability in critical domains, especially in the context of autonomous vehicles. Black-box trajectory prediction models commonly used in these domains may exhibit unexpected deficiencies, potentially leading to risks. By monitoring the prediction performance online, systems can proactively identify potential insufficiencies and make informed decisions to ensure safer and more reliable operations. The PMBP offers practitioners different monitoring solutions based on various approaches, addressing their specific needs effectively. While the PMBP has shown promising outcomes, further exploration and testing are necessary to fully harness and apply its monitoring results in automated systems. Practitioners are encouraged to adopt the PMBP as an essential monitoring mechanism to enhance the reliability of their trajectory prediction models and achieve safer and more efficient automation in their domains.
更多查看译文
关键词
Monitor,trajectory prediction,black-box model,autonomous vehicle,reliability
AI 理解论文
溯源树
样例
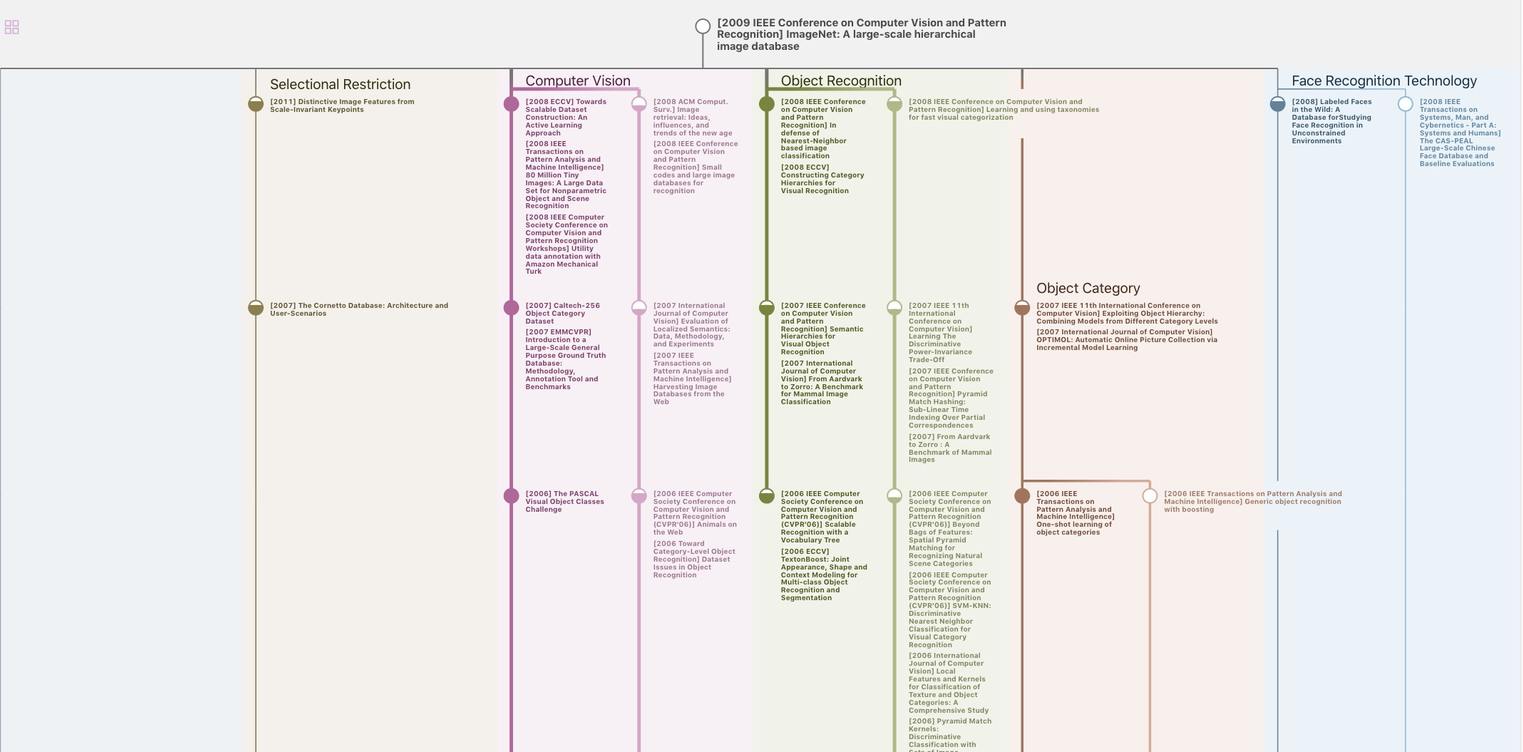
生成溯源树,研究论文发展脉络
Chat Paper
正在生成论文摘要