NNE -Tracking: A Neural Network Enhanced Framework for Device-free Wi-Fi Tracking
IEEE Transactions on Mobile Computing(2024)
摘要
The evolution of Wi-Fi to next-generation 802.11bf demonstrates the potential of device-free Wi-Fi sensing applications, where we can remotely infer the behaviors of users without bringing into physical contact with them. Among these sensing applications, Wi-Fi tracking is critical to provide location based services. Recent Wi-Fi tracking systems can be cataloged into model-based and data-based approaches: (1) the model-based approach is to build the mathematical tracking model. However, this method is sensitive to environmental noise, and spends more execution time; (2) the data-based approach is to train a neural network. However, this method requires a lot of efforts to collect training dataset, and cannot handle all types of trajectories well. To resolve these issues, we propose the
NNE-Tracking
, a Neural Network Enhanced tracking framework. The core design principle of
NNE-Tracking
is as follows: we improve the tracking accuracy based on the data-based approach, and utilize the model-based approach to supervise whether the neural network is already working well. Moreover, we also design a framework to estimate unknown parameters of the tracking model, so that the system can automatically generate the Wi-Fi map. We take the Wi-Fi passive tracking as a specific example to explain how to apply
NNE-Tracking
in practical applications. Experimental results demonstrate that our design can reduce 59.4% ∼ 85.3% tracking errors while significantly saving execution time. As for deployment costs, we can automatically infer the Wi-Fi map without manual calibration; As for stability, when we repeat the training process with different hidden layers and random seeds, the tracking standard deviation of these neural networks is only 1.4cm.
更多查看译文
关键词
Indoor localization,Channel state information (CSI),Neural network
AI 理解论文
溯源树
样例
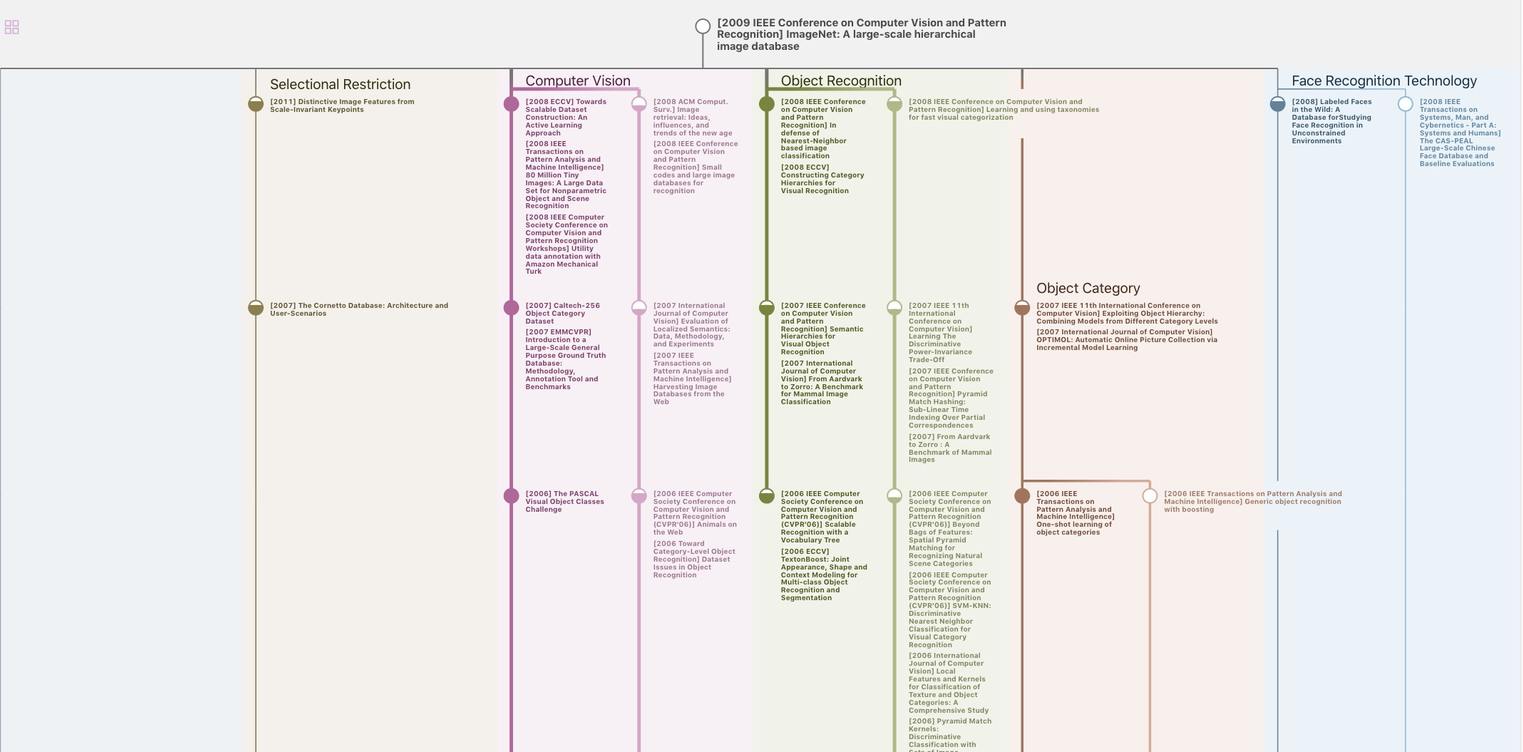
生成溯源树,研究论文发展脉络
Chat Paper
正在生成论文摘要