Decentralized Resource Allocation for Multi-Radar Systems Based on Quality of Service Framework
IEEE Transactions on Signal Processing(2024)
Univ Elect Sci & Technol China | Seoul Natl Univ
Abstract
Resource allocation plays a crucial role in the design of multi-radar systems (MRS) for sensing applications. Conventional approaches involve centrally computing the resource allocation solution, assuming the existence of a fusion center (FC). However, these approaches lead to a significant computational burden associated with the FC and fail to yield a viable solution when employing decentralized network architectures. To address the limitations of the centralized approach, this paper proposes a decentralized resource allocation framework. The general resource allocation problem for MRS is comprehensively formulated as an optimization problem based on the quality of service model. To facilitate decentralized optimization, a logarithmic barrier method is employed to approximate the objective function as a linear combination of individual task utility functions. These utility functions can be sequentially updated at each node by communicating with adjacent nodes. The global solution of the optimization problem is obtained when all nodes reach an agreement on resource allocation after a sufficient number of iterations. It is demonstrated that the formulated objective function is unbounded, which is incongruent with the applicable form of common decentralized solution algorithms. To overcome this, a constrained walk alternating direction method of multipliers (CW-ADMM) algorithm is proposed, which ensures an acceptable communication cost while finding the solution. A parallel acceleration approach that employs a broadcast-oriented mechanism is provided to further improve the solution efficiency. Finally, two typical scenarios of MRS resource allocation are investigated to empirically validate the effectiveness of the proposed algorithms.
MoreTranslated text
Key words
Multi-radar system,resource allocation,target localization,target tracking,decentralized optimization
求助PDF
上传PDF
View via Publisher
AI Read Science
AI Summary
AI Summary is the key point extracted automatically understanding the full text of the paper, including the background, methods, results, conclusions, icons and other key content, so that you can get the outline of the paper at a glance.
Example
Background
Key content
Introduction
Methods
Results
Related work
Fund
Key content
- Pretraining has recently greatly promoted the development of natural language processing (NLP)
- We show that M6 outperforms the baselines in multimodal downstream tasks, and the large M6 with 10 parameters can reach a better performance
- We propose a method called M6 that is able to process information of multiple modalities and perform both single-modal and cross-modal understanding and generation
- The model is scaled to large model with 10 billion parameters with sophisticated deployment, and the 10 -parameter M6-large is the largest pretrained model in Chinese
- Experimental results show that our proposed M6 outperforms the baseline in a number of downstream tasks concerning both single modality and multiple modalities We will continue the pretraining of extremely large models by increasing data to explore the limit of its performance
Upload PDF to Generate Summary
Must-Reading Tree
Example
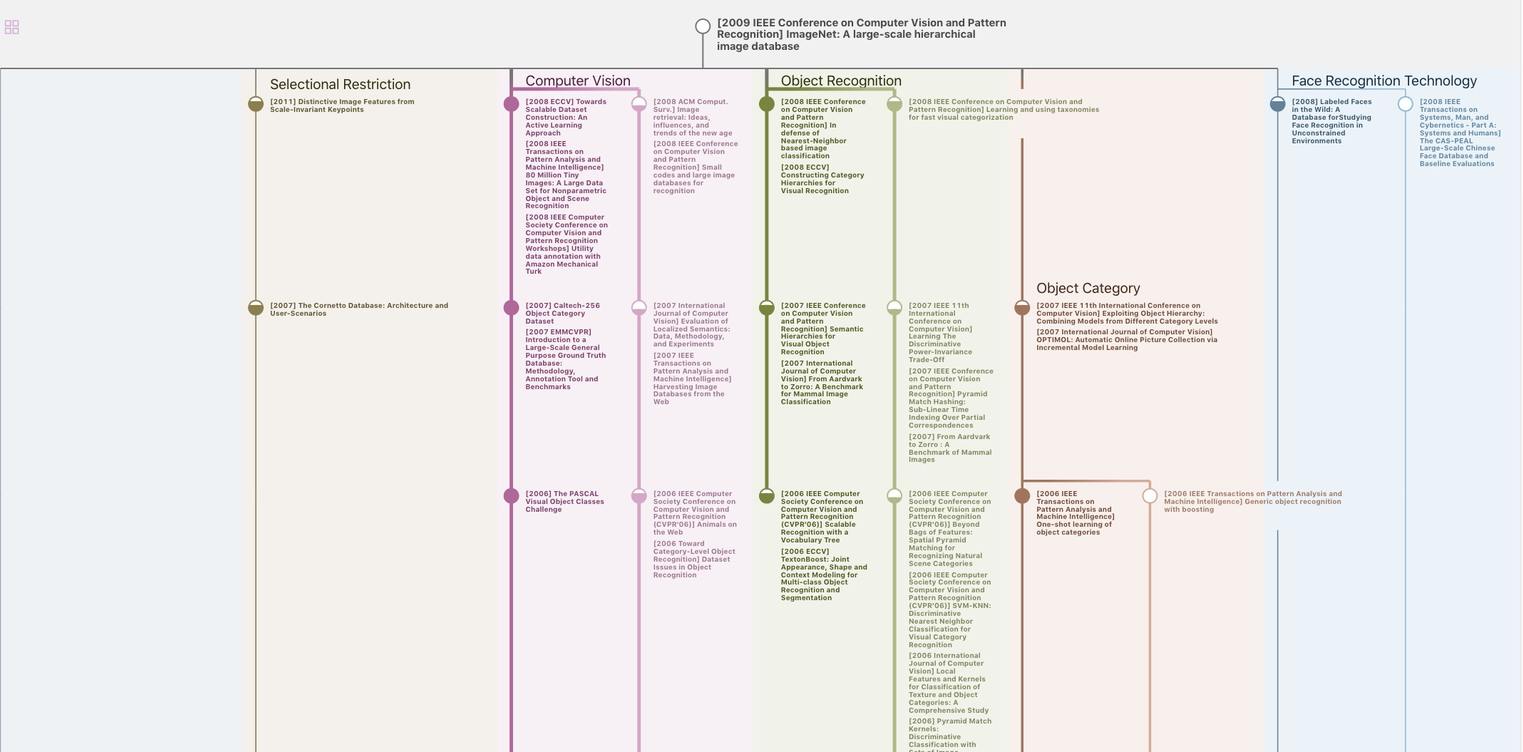
Generate MRT to find the research sequence of this paper
Related Papers
2010
被引用23833 | 浏览
2024
被引用272 | 浏览
2015
被引用36 | 浏览
2016
被引用128 | 浏览
2017
被引用72 | 浏览
2019
被引用28 | 浏览
2019
被引用70 | 浏览
2020
被引用64 | 浏览
Collaborative Detection and Power Allocation Framework for Target Tracking in Multiple Radar System.
2020
被引用135 | 浏览
2020
被引用156 | 浏览
2020
被引用121 | 浏览
2021
被引用82 | 浏览
2021
被引用81 | 浏览
2023
被引用9 | 浏览
Data Disclaimer
The page data are from open Internet sources, cooperative publishers and automatic analysis results through AI technology. We do not make any commitments and guarantees for the validity, accuracy, correctness, reliability, completeness and timeliness of the page data. If you have any questions, please contact us by email: report@aminer.cn
Chat Paper