Deep Learning Based Joint Hybrid Precoding and Combining Design for mmWave MIMO Systems.
IEEE Syst. J.(2024)
摘要
To maximally enhance the hybrid precoding performance, an innovative deep learning-based optimization algorithm is given for a multiuser fully connected (MUFC) structure in this article. First, a joint optimization framework based on convolutional neural network (CNN) is suggested, namely, JOCNN, which can synchronously optimize the hybrid precoder and combiner. In the JOCNN, analog precoding neural network (AP-NN), digital precoding neural network (DP-NN), analog combining neural network (AC-NN), and digital combining neural network (DC-NN) are designed to satisfy the nonconvex constant modulus constraint and the power constraint. Then, using a training strategy based on unsupervised learning, the developed JOCNN can be trained to maximize the spectral efficiency (SE). At last, the thoroughly trained JOCNN model can take in the estimated channel matrix as the input and immediately output the analog precoding matrix, the digital precoding matrix, the analog combining matrix, and the digital combining matrix. Simulation results demonstrate that the suggested method can outperform the previous works in terms of SE while requiring less computation time.
更多查看译文
关键词
Convolutional neural network (CNN),deep learning (DL),hybrid precoding,multiple-input multiple-output (MIMO),millimeter-wave (mmWave)
AI 理解论文
溯源树
样例
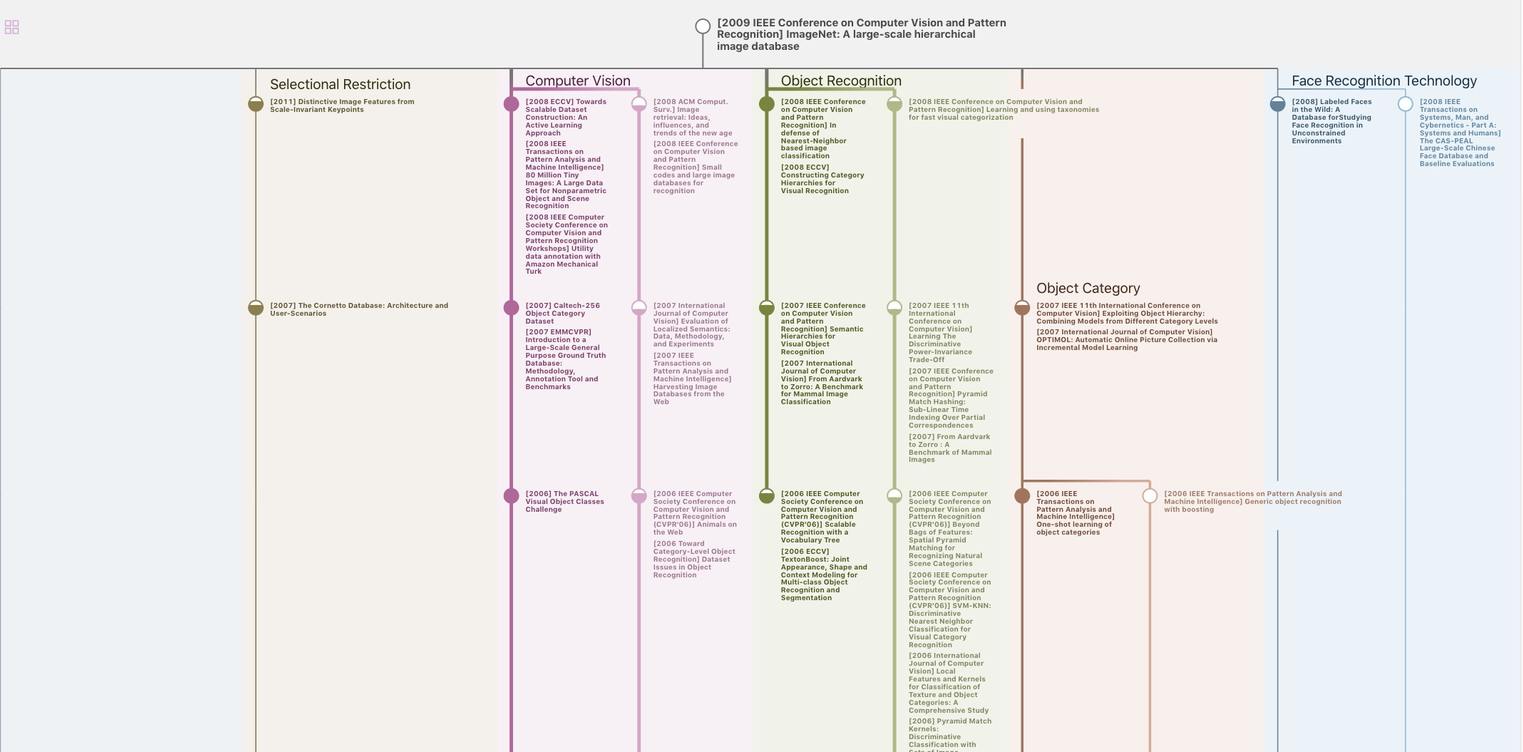
生成溯源树,研究论文发展脉络
Chat Paper
正在生成论文摘要