Risk-Sensitive Mobile Battery Energy Storage System Control With Deep Reinforcement Learning and Hybrid Risk Estimation Method
IEEE Transactions on Smart Grid(2024)
摘要
The mobile battery energy storage systems (MBESS) utilize flexibility in temporal and spatial to enhance smart grid resilience and economic benefits. Recently, the high penetration of renewable energy increases the volatility of electricity prices and gives MBESS an opportunity for price difference arbitrage. However, the strong randomness of both the traffic system and renewable energy leads to difficulty in achieving profit with acceptable risk. To address this problem, this paper proposes a risk-sensitive MBESS control framework based on safe deep reinforcement learning, which can constrain the risk under a certain level according to the company’s risk preference. The risk-estimation safe deep deterministic policy gradient (RE-SDDPG) algorithm is proposed to learn the optimal policy under the premise of lacking direct risk signals. Moreover, a hybrid risk estimation method is proposed to avoid local convergence caused by inaccurate estimation during the learning process. Last, a parameter-sharing method is applied to increase learning efficiency by sharing the Q networks’ parameters. The proposed methods are tested in the IEEE 30-bus system. The results show that the proposed method can keep the profit at a relatively high level while reducing the risk and increase learning efficiency compared with existing methods.
更多查看译文
关键词
Mobile battery energy storage system,safe deep reinforcement learning,hybrid risk estimation,parameter-sharing method
AI 理解论文
溯源树
样例
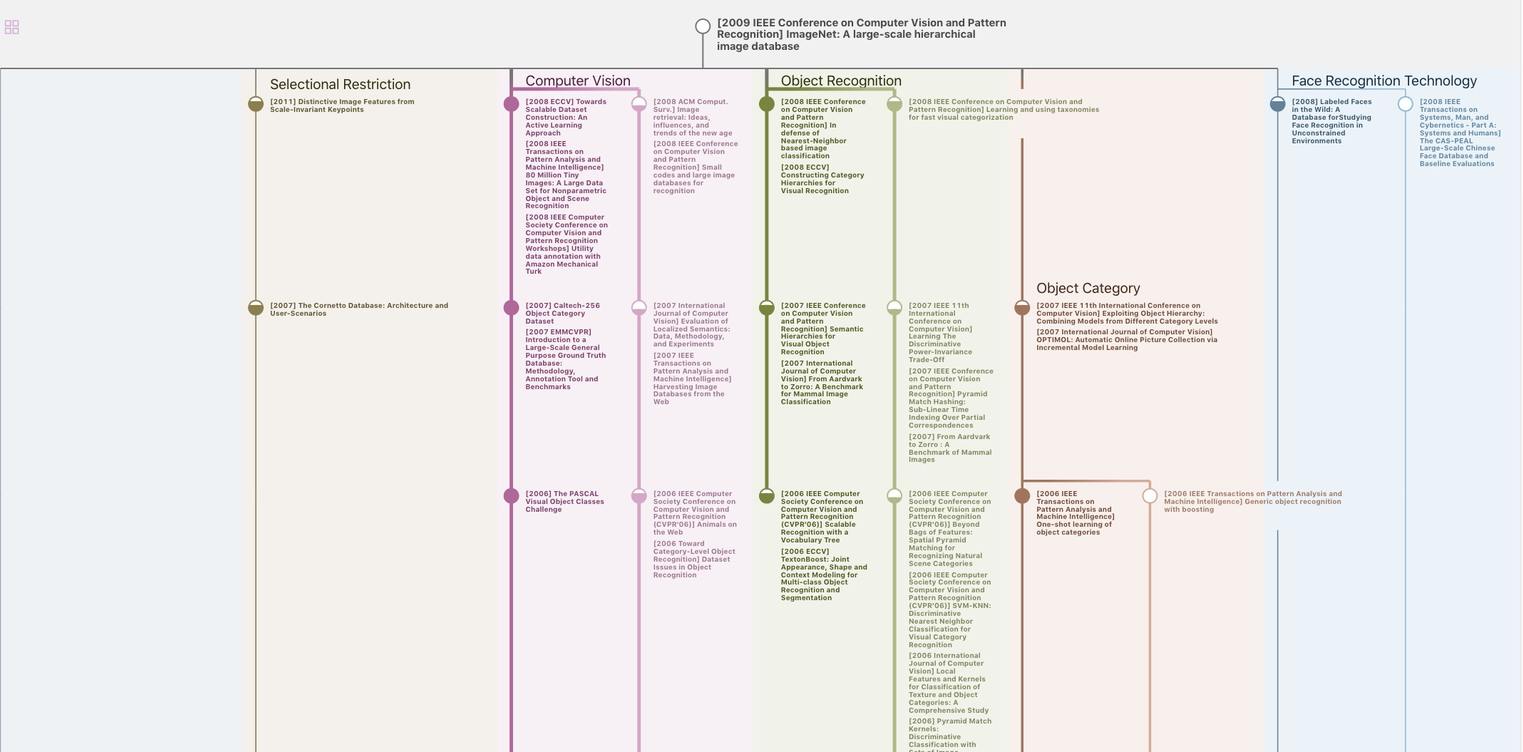
生成溯源树,研究论文发展脉络
Chat Paper
正在生成论文摘要