A Few-Shot Learning method for Non-Intrusive Load Monitoring with V-I Trajectory Features
IEEE Sensors Journal(2024)
摘要
Non-intrusive load monitoring (NILM) is a technique that determines the status of electrical appliances as well as their detail power consumption data by analyzing the total power consumption of a user. In practice, the electrical appliances in different households are different and the types of appliances in the same home are constantly changing. Therefore, it is challenging to obtain sufficient labeled data to train a model and to handle situations where there are changes in the types of appliances within a household. Consequently, it is necessary to propose an algorithm that needs merely a small amount of labeled data for identifying newly added appliances and does not necessitate retraining. In this paper, a few-shot learning method based on V–I trajectory signature has been proposed for NILM. Based on the prototype network and the matching network, we construct prototypes for each class using multiple labeled V-I trajectory images. These labeled images represent different brands, operational states, and devices within the class. To predict the label of a test sample, we compute the squared Euclidean distance between the test sample and all prototypes of each class. Different from the most of traditional methods, the model can classify any number of classes of V-I trajectory images without retraining or fine-tuning. Moreover, the proposed approach demonstrates its efficacy and superiority by utilizing only a limited number of V-I trajectory images for each appliance category, as evidenced by the experimental results on two publicly available NILM datasets.
更多查看译文
关键词
Classification,Non-intrusive load monitoring,few-shot learning,load identification,V-I trajectory,load signature
AI 理解论文
溯源树
样例
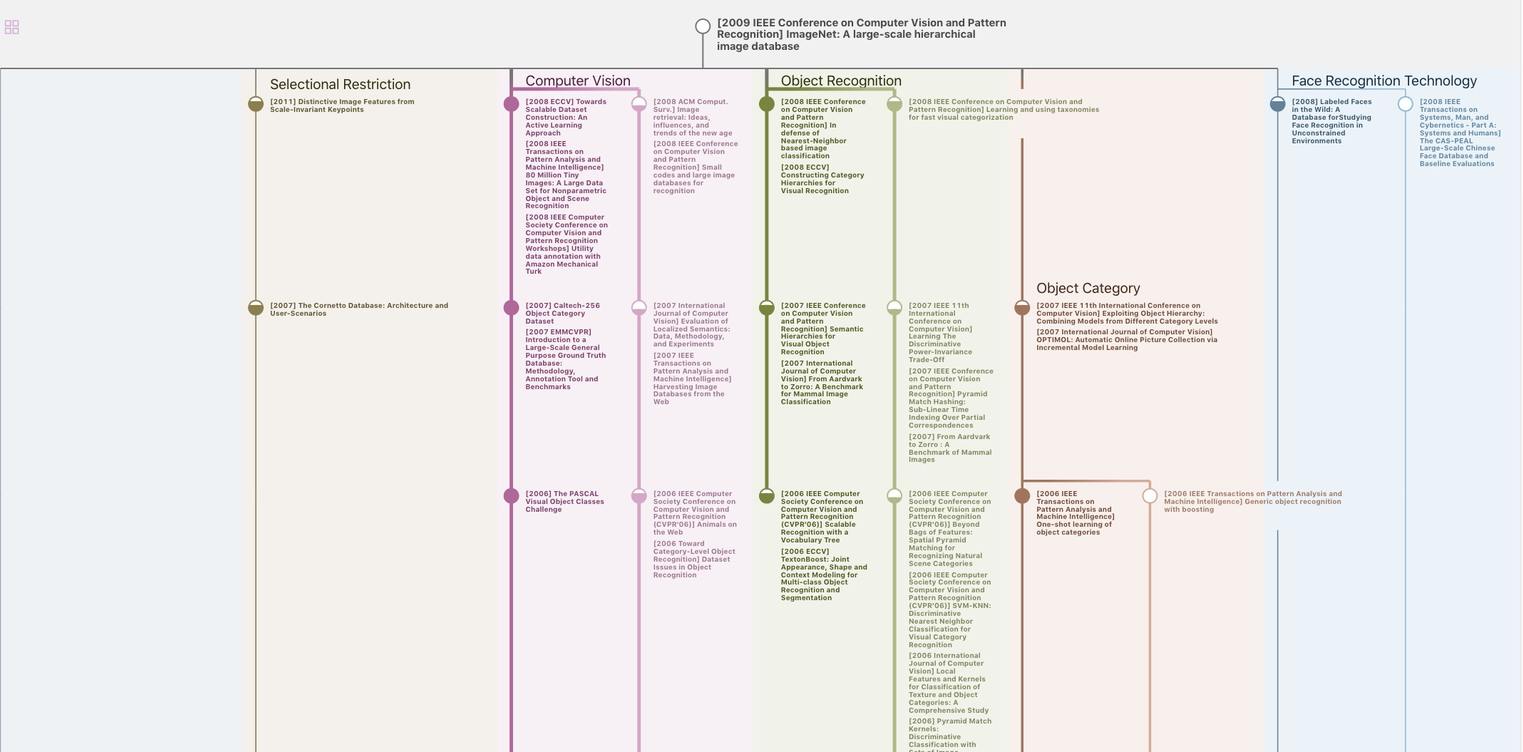
生成溯源树,研究论文发展脉络
Chat Paper
正在生成论文摘要