Quantification of dysnatremia using single-beam acoustic microbeam and convolutional neural networks
IEEE Sensors Journal(2024)
摘要
Recently, the use of artificial intelligence (AI) in cell analysis has gained significant attention, with particular focus on ultrasound-based AI for single-cell analysis. One application is diagnosing diseases by using ultrasound signals to analyze the physical properties contained in the signals. Dysnatremia, which can result in severe consequences to health such as stroke and cardiovascular disease, can be measured using blood sodium tests. However, these tests are performed by drawing blood, and obtaining the results requires a considerable amount of time. In addition, it has low reliability because the results vary depending on the inspection equipment and inspection method. In this study, we propose a novel approach for the quantification of dysnatremia using a single-beam acoustic microbeam (SBAM) and convolutional neural networks (CNNs). A 90 MHz transducer was fabricated and used to obtain reflected signals from red blood cells (RBCs), which are affected by the shape of the cells which, in turn, depend on the sodium concentration. Blood samples with varying sodium chloride concentrations were tested, and the reflected signals were analyzed using a CNN, for automation as opposed to manual analysis. The accuracy of the classification of the blood samples into 10 and 5-level groups, based on the sodium chloride concentration, was 0.961 and 0.942, respectively, as determined using CNNs. The results of this study demonstrate the potential of SBAM and CNN technologies for efficient quantification of sodium concentration in blood. This technology will help to diagnose dysnatremia in a non-invasive way with reduced analysis time and high accuracy.
更多查看译文
关键词
convolutional neural networks (CNN),dysnatremia,red blood cell (RBC),single-beam acoustic microbeam (SBAM),ultrasound
AI 理解论文
溯源树
样例
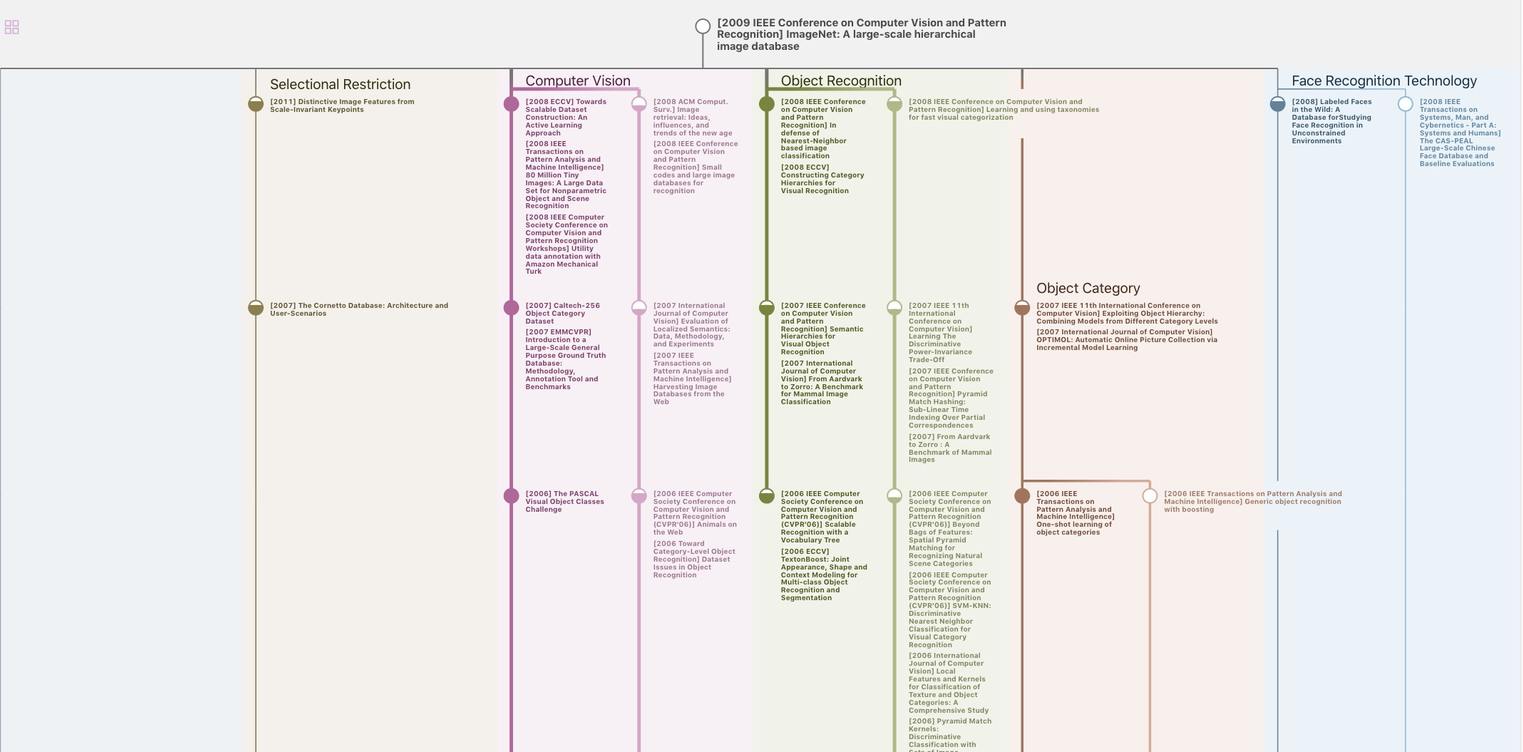
生成溯源树,研究论文发展脉络
Chat Paper
正在生成论文摘要