ECNet: Network Based on Encoder with Local Convolutional Attention and Cross Layer Information Fusion for Natural Gas Pipeline Leakage Detection
IEEE sensors journal(2024)
摘要
To address the issues of insufficient temporal information feature extraction and detection accuracy in natural gas pipeline leakage detection, we propose ECNet, an intelligent detection network based on an improved encoder [containing local convolutional attention (LCA)] and cross-layer information fusion (CLIF). First, by using the dual-branch cascading method to separately connect two encoders, we can extract features from the two-channel combined leakage time series data in a channel-wise and time-step-wise manner, allowing us to consider both global and local temporal information simultaneously. Second, we design a CLIF method to enhance the model's multilevel expression ability by achieving an interactive fusion of feature information between the intermediate layers and the top layer in the cascaded structure of the dual branches. Finally, we compare ECNet's performance with other deep learning methods by commonly used evaluation metrics. The experimental results show that ECNet has higher detection accuracy, better stability performance, and relatively moderate operating efficiency, which makes it effective and reliable in the field of natural gas pipeline leak detection.
更多查看译文
关键词
Feature extraction,Pipelines,Convolution,Natural gas,Time series analysis,Data models,Deep learning,Cross-layer information fusion (CLIF),deep learning,leaky aperture,local convolutional attention (LCA) encoder,multivariate time series classification
AI 理解论文
溯源树
样例
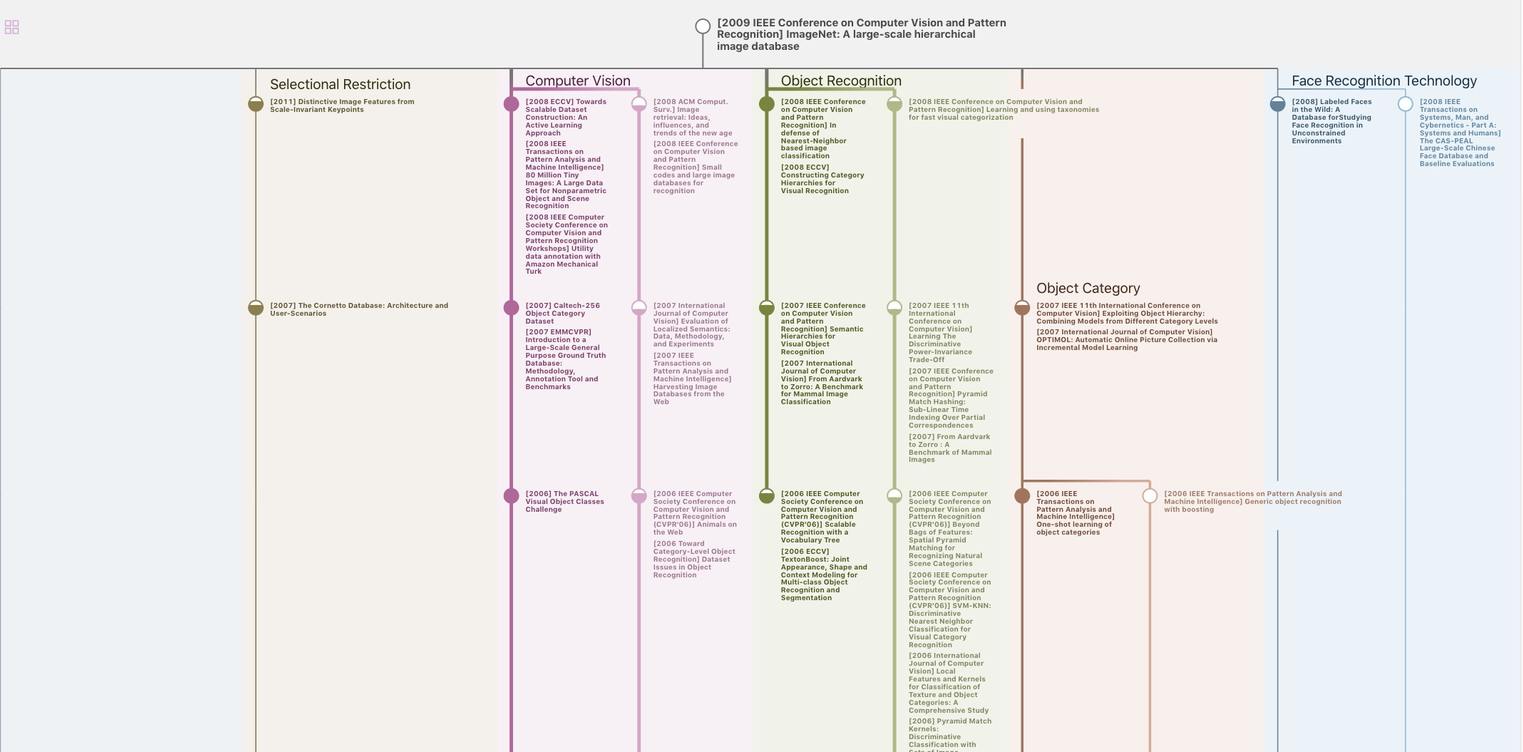
生成溯源树,研究论文发展脉络
Chat Paper
正在生成论文摘要